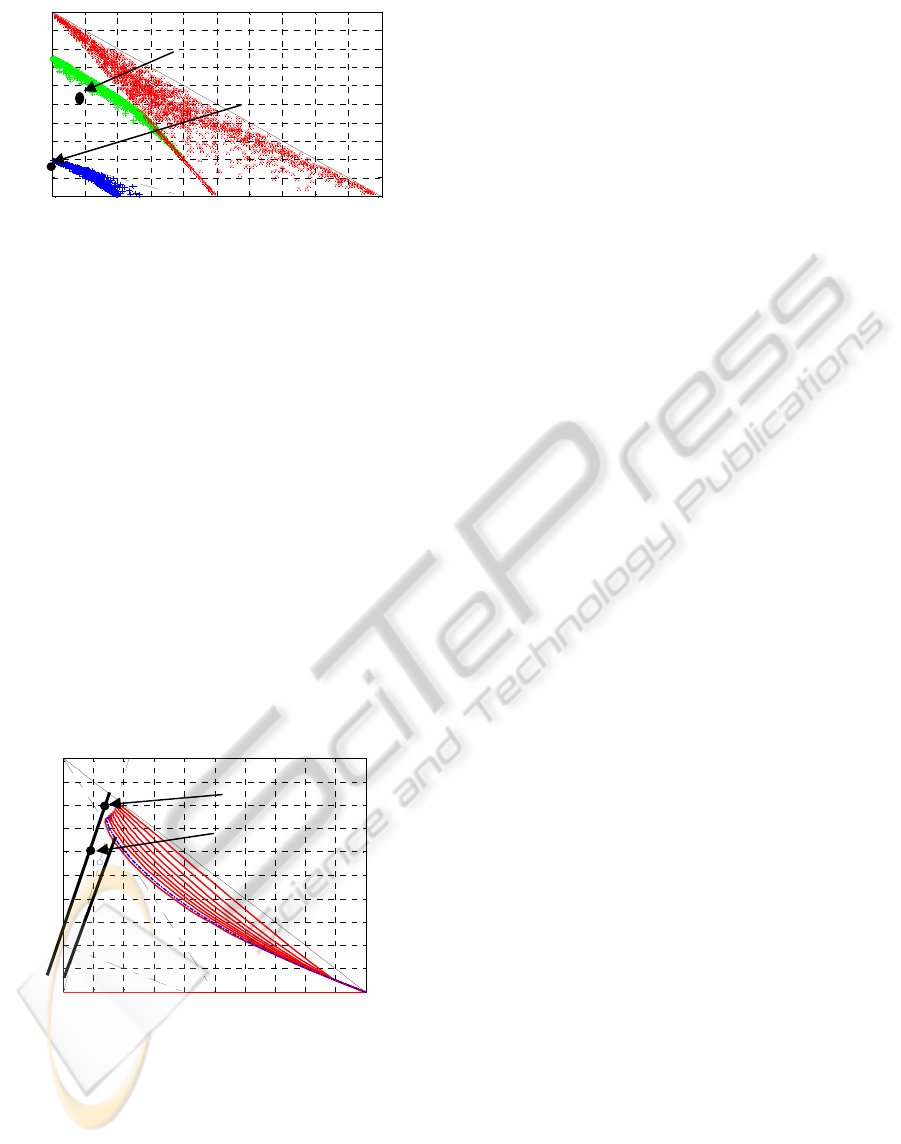
Figure 3: Projection in plan (m
1
, m
2
+2.m
3
) of M
mmc
for the
CPN of fig. 1 with various x
max j
[0.1 : 10], j=1,…,4.
The propositions 2 and 3 are used to work out the
admissible ratio
and the maximal firing speeds
that lead to homothetic approximations of
M
mms1
(SPN) . In the region A
2
, (7) leads to (10):
51
1
5
2
3
4
5
0
.'
0
.'
10010 0
..'
1/2000 1 4
"
5
"
4
5
x
mms
mms
mms
T
mms
T
mms
m
I
m
m
m
Y
m
Y
(9)
and then to the admissible interval
[5/(2.m
mms1
+
m
mms2
+2.m
mms3
) : 5/(m
mms1
+m
mms2
+2.m
mms3
)]. For the
considered example
[1.26: 1.48]. The figure 4
illustrates various homothetic marking trajectories
for SPN obtained for some values of parameter
in
admissible interval in order to reach
.M’
mms1
(μ).
Figure 4: Projection in plan (m
1
, m
2
+2.m
3
) of the
homothetic convergence to
.M’
mms
(μ).
The proposition 3 is used to work out the set of the
admissible maximal firing speeds that depend on the
parameter
such that the CPN with same structure
and initial marking tends to M
mms
:
x
max1
= 2.x
max4
.m
mms3
/ m
mms1
x
max2
=
.x
max4
. m
mms3
/ (5-
.(m
mms1
+m
mms2
+2.m
mms3
))
x
max3
= x
max4
m
mms3
/ m
mms2
(10)
where x
max4
is a dof. For example, consider the
particular homothetic ratio
= 4/3. The trajectory
(dotted line in figure 4), obtained for X
max
=(4.25,
3.41, 6, 1) results in asymptotic marking m’
mmc1
=
0.80, m’
mmc2
= 0.28, m’
mmc3
= 1.71. From this
approximation, it is easy to recover the asymptotic
stochastic mean marking M
mms
(
).
5 CONCLUSIONS
This paper has proposed partial homothetic
transformations of the SPN mean marking to
approximate. The proposed results concern the
existence of solutions but are not constructive in the
sense that the asymptotic stochastic mean markings
to estimate by CPNs must a priori be known. The
selection of the best projectors and ratios will be
investigated in our further works. Our future work is
also to investigate continuous approximations
directly derived from the SPNs transition firing
rates.
REFERENCES
Bobbio A., Puliafito A., Telek M., Trivedi K. (1998)
Recent Developments in Stochastic Petri Nets, J. of
Cir., Syst., and Comp., Vol. 8, No. 1, pp. 119--158.
David R., Alla H., (1992) Petri nets and grafcet – tools for
modelling DES, Prentice Hall, London.
Júlvez G., Recalde L. Silva M. (2005) Steady-state
performance evaluation of continuous mono-T-
semiflow Petri nets, Automatica, 41 (4), pp. 605-616.
Lefebvre D., Leclercq E., Khalij L., Souza de Cursi E., El
Akchioui N. (2009), Approximation of MTS
stochastic Petri nets steady state by means of
continuous Petri nets: a numerical approach, Proc.
IFAC ADHS, pp. 62-67, Zaragoza, Spain.
Lefebvre D., Leclercq E., (2010), Approximation of
Stochastic Petri Nets steady state by CPNs with
constant and piecewise-constant maximal firing
speeds, MOSIM 2010, Hammamet, Tunisia.
Molloy M.K., (1982) Performance analysis using
stochastic Petri nets, IEEE Tran. on Computers C, vol.
31, pp. 913 – 917.
Rausand M. and Hoyland A. (2004), System reliability
theory: models, statistical methods, and applications,
Wiley, Hoboken, New Jersey.
Silva M. and Recalde L. (2004) On fluidification of Petri
Nets: from discrete to hybrid and continuous models,
An. Reviews in Control, Vol. 28, no. 2, pp. 253-266.
Vazquez R., Recalde L., Silva M., (2008) Stochastic
continuous-state approximation of markovian Petri net
systems, Proc. IEEE – CDC08, pp. 901 – 906, Cancun,
Mexico.
0 0.5 1 1.5 2 2.5 3 3.5 4 4.5 5
0
0.5
1
1.5
2
2.5
3
3.5
4
4.5
5
a)
m
m
2
+ 2m
3
0 0.5 1 1.5 2 2.5 3 3.5 4 4.5 5
0
0.5
1
1.5
2
2.5
3
3.5
4
4.5
5
m1
m2+2m3
a
= 1.26
= 1.48
M
mms
(
)
.M’
mms1
(
)
M
mmc
(X
max
)
M
mms
(
)
A
2
A
1
A
3
HOMOTHETIC APPROXIMATIONS FOR STOCHASTIC PN
367