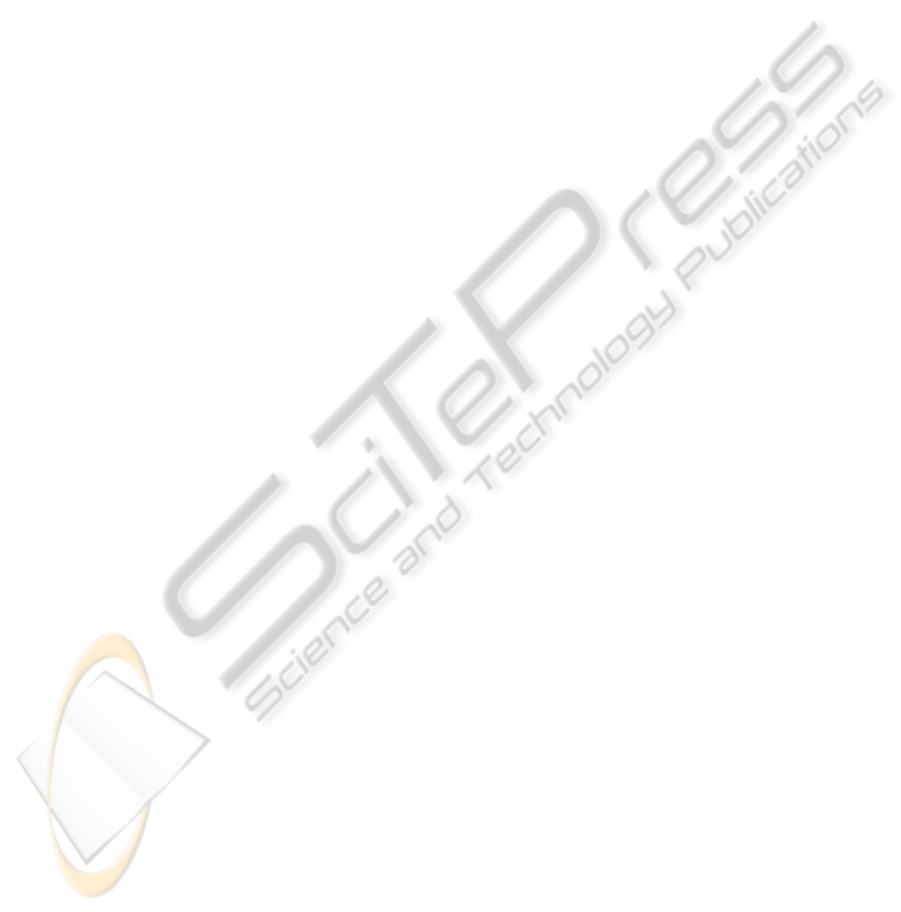
Active Silicon, Uxbridge, U.K.). Although da Vinci
system provides stereo vision, we only use the image
sequence from left camera to perform the mosaicing
in order to compare with other methods. 150 images
were captured from the endoscope but we use only
30 frames (every 5 frame from the sequence) for the
mosaicing. Our aim is to create a mosaicing image
which includes the whole structure of the coronary
artery. The main challenge is the large complicated
non-rigid motion introduced by the beating heart
surface, which is shown in the right bottom of Fig. 2
(b) and (c).
Fig. 2 (d) displays the mosaicing result of the
proposed method. We can notice that the whole
vessel structure has been built correctly. So the
surgeon can realize the environment outside the
current scene when he views a part of the vessel.
More importantly, the mosaicing image can help him
link the endoscopic video with the preoperative
information from CT/MRI scan. Brown’s method
was also tested using this image sequence and the
mosaicing result was displayed in Fig. 2 (e). It is
noticed that only part (around three quarters) of the
whole vessel had been constructed and the images
affected badly by the beating heart surface could not
be used by Brown’s method. The possible reason is
that SIFT feature descriptor could not find enough
reliable features from the images with severe
deformation from the internal organ or soft tissue.
4 CONCLUSIONS
In this paper, we proposed a robust video mosaicing
method for robotic assisted minimally invasive
surgery. The mosaicing image displays a much wider
field-of-view of the operation scene and helps the
surgeon realize the surrounding environment outside
the current view. Experiments with TECAB
endoscopic images and FCM images show that the
proposed method performs better than other typical
methods. It is robust to deformation caused by
organs and soft tissues and can even deal with
artefacts involved in the images.
Effort in the near future will focus on future
improvement of robustness to deformation and
artefacts. Our long term goal is to automatically
construct mosaicing image of the surgical scene,
reconstruct the internal organ surfaces and register
these with the preoperative data (CT or MRI) to
provide more information for image guided
diagnosis and treatment.
REFERENCES
Alizadeh, F. and Goldfarb, D, 2003. Second-order cone
programming,
Mathematical Programming, 95 (1),
3-51.
Brown, M. and Lowe, D. G, 2007. Automatic Panoramic
Image Stitching using Invariant Features,
International Journal of Computer Vision, 74, 59–73.
Capel D. P. , 2001. Image Mosaicing and
Super-Resolution, Ph.D thesis, Dept. of Eng. Science,
Univ. of Oxford.
Kahl, F. and Hartley, R, 2008. Multiple-View Geometry
Under the Linfinity-Norm,
IEEE Trans. Pattern Anal.
Mach. Intell.
30(9): 1603-1617
Lowe, D. G., 2004: Distinctive Image Features from
Scale-Invariant Keypoints.
International Journal of
Computer Vision
, 60, 91–110.
McLauchlan, P. and Jaenicke, A, 2002. Image mosaicing
using sequential bundle adjustment.
Image and Vision
Computing
, 20(9–10):751–759.
Milgram D. L., 1975. Computer Methods for Creating
Photomosaics,
IEEE Trans. Computers, 24(11),
1113-1119.
Miranda-Luna, R., Daul, C., Blondel, W.C.P.M.,
Hernandez-Mier, Y., Wolf, D., Guillemin, F., 2008.
Mosaicing of Bladder Endoscopic Image Sequences:
Distortion Calibration and Registration Algorithm.
IEEE Trans. on Biomedical Engineering 55, 541–553.
Quan, L., 1994. Invariants of 6 points from 3 uncalibrated
images.
In: Proc. ECCV , 2, 459-470.
Shashua, A., 1995. Algebraic functions for recognition.
IEEE Trans. Pattern Analysis and Machine
Intelligence
, 17 (8), 779-789.
Seshamani, S., Lau W., and Hager, G., 2006. Real-Time
Endoscopic Mosaicking.
In: Proc. MICCAI, 355-363.
Tomasi, C., and Kanade, T., 1992. Shape and Motion from
Image Streams under Orthography: a Factorization
Method.
Int. J. Computer Vision, 9(2), 137-54.
Triggs, W., McLauchlan, P., Hartley, R., and Fitzgibbon,
A. 1999. Bundle adjustment: A modern synthesis.
In
Vision Algorithms: Theory and Practice
, number 1883
in LNCS. Springer-Verlag. Corfu, Greece, pp.
298–373.
Vercauteren, T., Perchant, A., Malandain, G., Pennec, X.,
Ayache, N., 2006. Robust mosaicing with correction
of motion distortions and tissue deformation for in
vivo fibered microscopy.
Medical Image Analysis, 10
(5), 673-692.
Zoghlami, I., Faugeras, O., and Deriche, R. 1997. Using
geometric corners to build a 2D mosaic from a set of
images. In Proc. CVPR, 420-425.
http://www.ics.forth.gr/~lourakis/sba/
A ROBUST MOSAICING METHOD FOR ROBOTIC ASSISTED MINIMALLY INVASIVE SURGERY
211