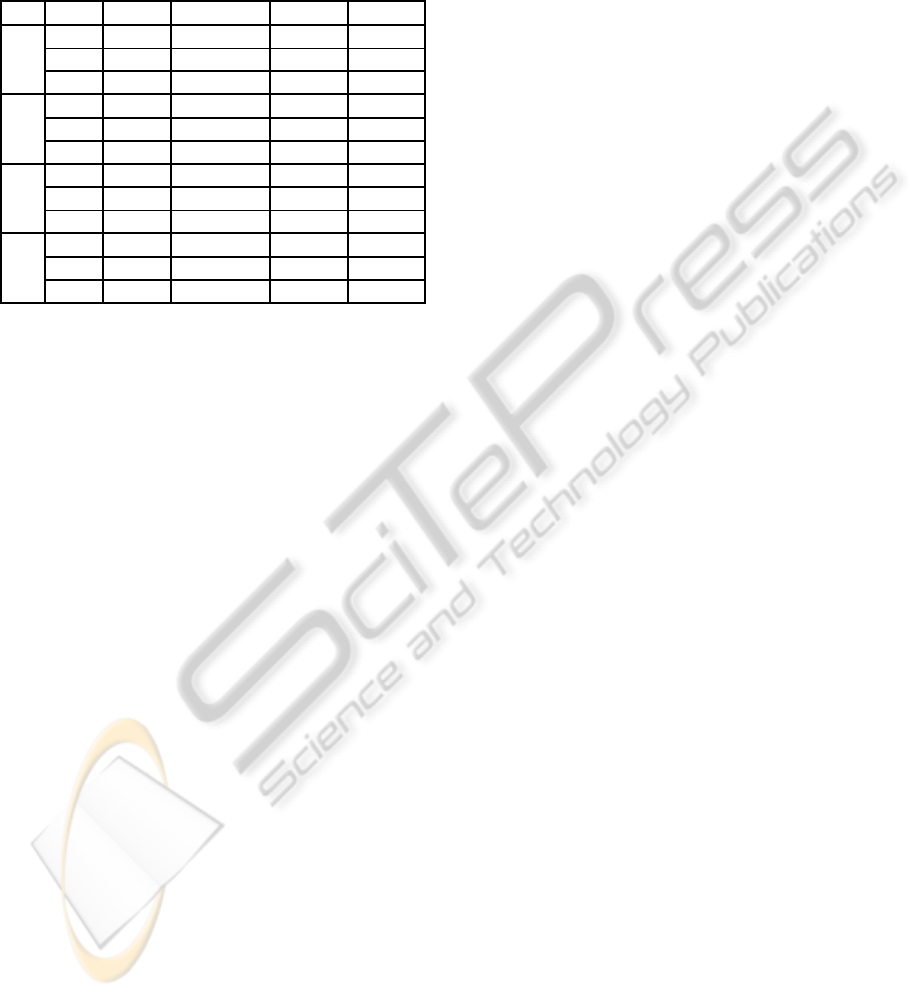
Table 1: Performance of our control approach (C) in com-
parison with the Horn and Schunck approach (HS) and the
dynamic image motion approach (Dy) in presence of noise:
We added random Gaussian noise with zero mean and stan-
dard deviation σ = 0, 10, 20, and 40 to the Yosemite image
sequence.
σ app. α β RMSE AAE
0 HS 0.005 - 0.177 3.04
◦
Dy 0.006 0.00002 0.178 3.09
◦
C 0.007 0.0005 0.169 2.88
◦
10 HS 0.008 - 0.283 5.74
◦
Dy 0.01 0.0003 0.275 5.68
◦
C 0.009 0.0001 0.243 4.92
◦
20 HS 0.02 - 0.429 8.61
◦
Dy 0.025 0.001 0.395 7.54
◦
C 0.02 0.00001 0.350 6.67
◦
40 HS 0.05 - 0.640 13.27
◦
Dy 0.05 0.005 0.523 9.89
◦
C 0.05 0.000003 0.497 9.16
◦
porates also future knowledge of the image sequence
instead of using only past information with a predic-
tion as in (Stahl et al., 2006).
5 CONCLUSIONS
We have presented an optimal control approach to
image motion estimation including physical prior
knowledge in a novel and exploratory way. It leads to
an unconstrainedoptimisation problem, where the op-
timality system - from which the optimal state and the
optimal control are determined - can be solved using
an iterative gradient descent method. The forward-
backward structure of the model allows for a robust
estimation of the coherent flows by including prior
knowledge that enforce spatio-temporal smoothness
of the minimising vector field.
In the case that the image measurements indicate
changes of the current velocity distribution, fictive
control forces modify the system state accordingly.
The presence of such forces may serve as an indicator
notifying a higher-level processing stage about unex-
pected motion events in video sequences.
The comparison of our results with the approach
from (Horn and Schunck, 1981) and the approach
from (Stahl et al., 2006) demonstrates the ability of
the control formulation to determine image motion
from video sequences, and shows improved perfor-
mance, especially for highly noisy image data. Our
further work will include the modification of the
Burgers equation to achieve better motion boundaries
in the rarefaction area and the reformulation of the
approach to a receding horizon formulation.
ACKNOWLEDGEMENTS
We would like to thank Dr. Christian Schellewald,
Dr. Paul Ruhnau, Prof. Christoph Schn¨orr, and Prof.
Øyvind Stavdahl for some inspiring discussions and
comments.
REFERENCES
Alvarez, L., Esclar`ın, J., Lefebure, M., and S`anchez, J.
(1999). A PDE model for computing the optical flow.
In Proceedings of CEDYA XVI, pages 1349–1356.
Barron, J. L., Fleet, D. J., and Beauchemin, S. S. (1994).
Performance of optical flow techniques. Int. J. of
Computer Vision, 12(1):43–77.
Borzi, A., Ito, K., and Kunisch, K. (2002). Optimal control
formulation for determining optical flow. SIAM J. Sci.
Comput., 24(3):818–847.
Burgers, J. M. (1948). A mathematical model illustrating
the theory of turbulence. Adv. Appl. Mech., 1:171–
199.
Colella, P. and Puckett, E. G. (1998). Modern Nu-
merical Methods for Fluid Flow. Lecture Notes,
Dep. of Mech. Eng., Uni. of California, Berke-
ley, CA. http://www.rzg.mpg.de/ bds/numerics/cfd-
lectures.html.
Deriche, R., Kornprobst, P., and Aubert, G. (1995). Optical-
flow estimation while preserving its discontinuities: A
variational approach. In ACCV, pages 71–80.
Gunzburger, M. (2002). Perspectives in Flow Control
and Optimization. Society for Industrial and Applied
Mathematics.
Hirsch, C. (2000). Numerical Computation of Internal and
External Flows (Vol.I+II). John Wiley & Sons.
Horn, B. and Schunck, B. (1981). Determining optical flow.
Artificial Intelligence, 17:185–203.
Jain, R., Kasturi, R., and Schunck, B. G. (1995). Machine
Vision. McGraw-Hill, Inc.
Lucas, B. D. and Kanade, T. (1981). An iterative image reg-
istration technique with an application to stereo vision
(darpa). In Proc. of the 1981 DARPA Image Under-
standing Workshop, pages 121–130.
McCane, B., Novins, K., Crannitch, D., and Galvin, B.
(2001). On benchmarking optical flow. Comput. Vis.
Image Underst., 84(1):126–143.
Nagel, H. H. (1990). Extending the ‘oriented smoothness
constraint’ into the temporal domain and the estima-
tion of derivatives of optical flow. In Proc. of the first
european conf. on computer vision, pages 139–148.
Springer.
Ruhnau, P. and Schn¨orr, C. (2007). Optical Stokes flow:
An imaging-based control approach. Experiments in
Fluids, 42:61–78.
Schn¨orr, C. (1991). Determining optical flow for irregular
domains by minimizing quadratic functionals of a cer-
tain class. Int. J. of Computer Vision, 6(1):25–38.
ICINCO 2010 - 7th International Conference on Informatics in Control, Automation and Robotics
20