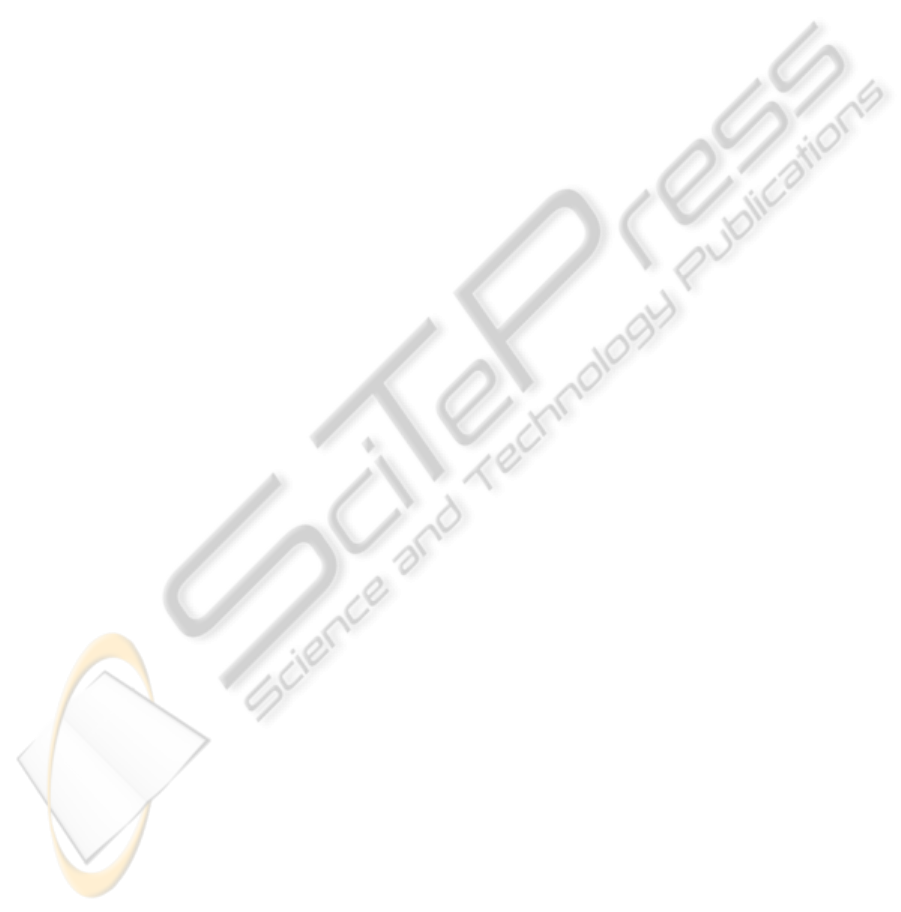
operation, defined at the configuration level, can re-
alize the same function. The obvious solution is to
reconfigure the system. Although, we propose to im-
prove the generated configuration and optimise the re-
quest completion time. Therefore, we need to extend
the “simple configuration” and add “reserved opera-
tions”. At this level, we define a “reserved operation”
as an operation chosen to replace a failed operation
in a configuration. This will help us carrying out
the request in the system without going through the
reconfiguration process. To do so, “reserved opera-
tions” are selected using the ‘AHP’ algorithm. When
the “reserved operations” are specified, we generate
the corresponding operation sequences to fill out the
generated configuration. In other words, we generate
reserved operation sequences to extend the obtained
“simple configuration” and get the “flexible configu-
ration” to improve system responsiveness. Projecting
this extension on our example, we get the “flexible
configuration” by duplicating operations since each
function (or transfer) can be realized using four re-
sources (M
1
- M
4
) for a function, and the robot or
a convoyer for a tranfer). We note that in our case
the obtained “flexible configuration” corresponds to
“162” operation sequence of the “maximal configu-
ration” using two machining resources (M
1
and M
2
)
and “used operations” are realized in priority.
5 CONCLUSIONS
Reconfigurable Manufacturing Systems are faced
with frequent disruptions that impact the production
quality. A low-cost solution is needed to recover
the system. In this paper, the use of a high con-
ception level for reconfigurable manufacturing sys-
tems is presented to include different features and de-
scribe both architecture and configuration. The sug-
gested framework produces “near-optimal ” configu-
ration by generating the corresponding “flexible con-
figuration” based on the generated “simple configu-
ration” and the architecture. A simple example illus-
trating the way we can use the proposed framework
is presented in section 4. First, the production envi-
ronment has been presented. Secondly, the “maximal
configuration” generation is presented by refining the
transformation module. Third, the “simple configura-
tion” generation process is described before moving
on to presenting the “flexible configuration” genera-
tion process. Configuration choices usually depend
on preferences, on choices obtained at the scheduling
level, and on constraintes defined by clients at the re-
quest level. “Minimal” and “Simple” configurations
are not always the best solutions. Configurations with
a higher degree of flexibility may respond to requests
with better measures, and may answer a better cost-
performance trade-off. Although the existing frame-
work provides opportunity for many different types
of analyses, additional extensions will be beneficial
as well. These include inventory management during
production and transfer sequence management; new
configuration extension rules; and more specific crite-
ria to improve operation choice. More generally, we
would like to permit a broader class of configurations,
such as serial parallel lines with crossover, so that the
framework can be applied in an even greater number
of circumstances.
ACKNOWLEDGEMENTS
The authors are pleased to acknowledge the sup-
port by the associate professor at Polytech’Marseille
Fouzia Ounnar.
REFERENCES
B´ezivin, J., Jouault, F., Rosenthal, P., and Valduriez, P.
(2005). The AMMA platform support for modeling
in the large and modeling in the small.
Dpto, A. G., Gmez, A., Fuente, D. D. L., Parreo, J., and
Puente, J. (2002). Scheduling in flexible manufactur-
ing systems. Applied Artificial Intelligence, 15:949–
963.
Hamani, N., Dangoumau, N., and Craye, E. (2009). Re-
active mode handling of flexible manufacturing sys-
tems. Mathematics and Computers in Simulation,
79(5):1421–1439.
Kanso, M., Berruet, P., and Philippe, J. (2009). Multi-
criteria decision making approach for reconfigurable
manufacturing systems. In proceeding ISBN of the
28th EAM Conference on Human Decision-Making
and manual Control, SEPT. 3-4, 2009, pages 37–44.
Kurnaz, S., Cohn, A., and Koren, Y. (2005). A frame-
work for evaluating production policies to improve
customer responsiveness.
Lamotte, F. D. (2006). Proposition d’une approche haut
niveau pour la conception, l’analyse et l’implantation
des systmes reconfigurables 2006. PhD thesis. Uni-
versit de Bretagne Sud, Lorient, France.
Nucci, F. and Grieco, A. (2008). The operational strategies
in focused flexible manufacturing systems. ISC’08
International Supercomputer Conference. Heidelberg,
Germany, June 18 20.
Ryu, K., Son, Y., and Jung, M. (2003). Modeling and spec-
ifications of dynamic agents in fractal manufacturing
systems. Comput. Ind., 52(2):161–182.
Terkaj, W., Tolio, T., and Valente, A. (2009). Designing
manufacturing flexibility in dynamic production con-
texts. In Design of Flexible Production Systems, pages
1–18.
ICINCO 2010 - 7th International Conference on Informatics in Control, Automation and Robotics
248