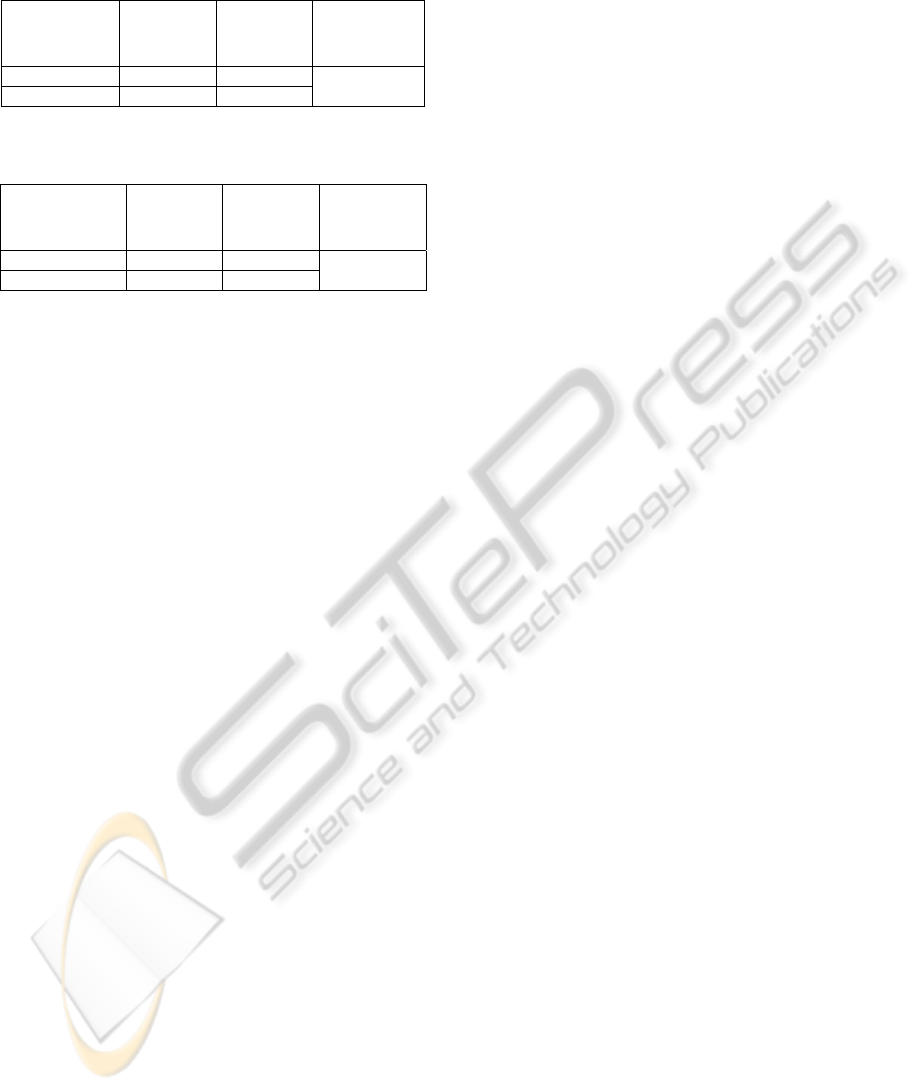
Table 5: Closed loop performances Values Obtained for
the First SISO System.
Overshoot
(
%
)
Settling
time (
T )
Variance of
the control
(
v
V
)
k∈ [0;400]
12 64s
0.69
k∈[401;800]
24 66s
Table 6: Closed loop Performances values for the Second
SISO System.
Overshoot
(
%
)
Settling
time (
T )
Variance of
the control
(
v
V )
k∈ [0;300]
05.8 71s
10.06
k∈[301;800]
12.8 77s
5 CONCLUSIONS
In this paper, a new method allowing the on line
adjustment of the predictive controller synthesis
parameters for multivariable systems has been
presented. The decentralized control using the
decoupling network is applied to decouple the
different subsystems and to control the MIMO
system using multiple SISO controllers. Genetic
algorithms and the weighted sum method are
exploited to find the synthesis parameters by
minimizing simultaneously three criteria which are
the overshoot, the settling time and the variance of
the control. The obtained simulation results have
shown that the proposed method can lead to
acceptable closed loop performances.
REFERENCES
Albertos, P., Sala, A., 2004. Multivariable control
systems: an engineering approach, Springer.
Bemporada, A., Muñoz de la Peñab, D., 2009.
Multiobjective model predictive control, Automatica,
vol. 45, issue 12, p. 2823-2830.
Ben Abdennour, R., Ksouri, M., Favier, G., 1998.
Application of fuzzy logic to the on-line adjustement of
the parameters of a generalized predictive controller.
Intelligent Automation and soft computing, vol.4, No
3, p.197-214.
Bouani, F., Laabidi, K., Ksouri, M., 2006. Constrained
Nonlinear Multi-objective Predictive Control, IMACS
Multiconference on "Computational Engineering in
Systems Applications"(CESA), Beijing, China, p.
1558-1565.
Bristol, E.H., 1966. On a new measure of interaction for
multivariable process control. IEEE Transactions on
control, p133-134.
Clarke, W., Mohtadi, C., Tuffs, P. S., 1987. Generalised
Predictive Control- parts I & II. Automatica, vol.23,
N° 2, p. 137-160.
Collette, Y., Siarry, P., 2002. Optimisation multiobjectif,
Editions Eyrolles.
Gambier, A., 2008. MPC and PID Control Based on
Multi-Objective Optimization. American Control
Conference, Washington, USA, p. 4727-4732.
Goldberg, D. E., 1991. Genetic Algorithms in search,
optimization and machine learning, Addison-Wesley,
Massachusetts.
Khelassi, A., Wilson, J.A., Bendib,R., 2004. Assessment of
Interaction in Process, Control Systems Dynamical
Systems and Applications Proceedings, Antalya,
Turkey, p. 463-471.
Miskovic, L., Karimi, A., Bonvin, D., Gevers, M., 2007.
Correlation-based tuning of decoupling multivariable
controllers, Automatica,
vol. 43, p. 1481-1494.
Moaveni, C., Khaki-Sedigh, A., 2006. Input-Output
Pairing based on Cross-Gramian Matrix, SICE-
ICASE International joint conference, Korea, p. 2378-
2380.
Muldera, E. F., Tiwari, P. Y., Kothare, M. V., 2009.
Simultaneous linear and anti-windup controller
synthesis using multiobjective convex optimization,
Automatica, vol.45, issue 3, p.805-811.
Popov, A., Farag, A., Werner, H., 2005. Tuning of a PID
controller Using a Multi-objective Optimization
Technique Applied to a Neutralization Plant, 44th
IEEE Conference on Decision and Control, and the
European Control Conference, Seville, Spain, p. 7139-
7143.
Richalet, J., Lavielle, G., Mallet, J., 2005. La commande
prédictive : mise en œuvre et applications
industrielles, Editions Eyrolles.
Yang, Z., Pedersen, G., 2006. Automatic Tuning of PID
Controller for a 1-D Levitation System Using a
Genetic Algorithm - A Real Case Study, IEEE
International Symposium on Intelligent Control,
Munich, Germany, p. 3098-3103.
Zalkind, C.S., 1967. Practical approach to non-
interacting control parts I and II. Instruments and
control systems, vol.40, No.3 and No.4.
DESIGN OF A MULTIOBJECTIVE PREDICTIVE CONTROLLER FOR MULTIVARIABLE SYSTEMS
115