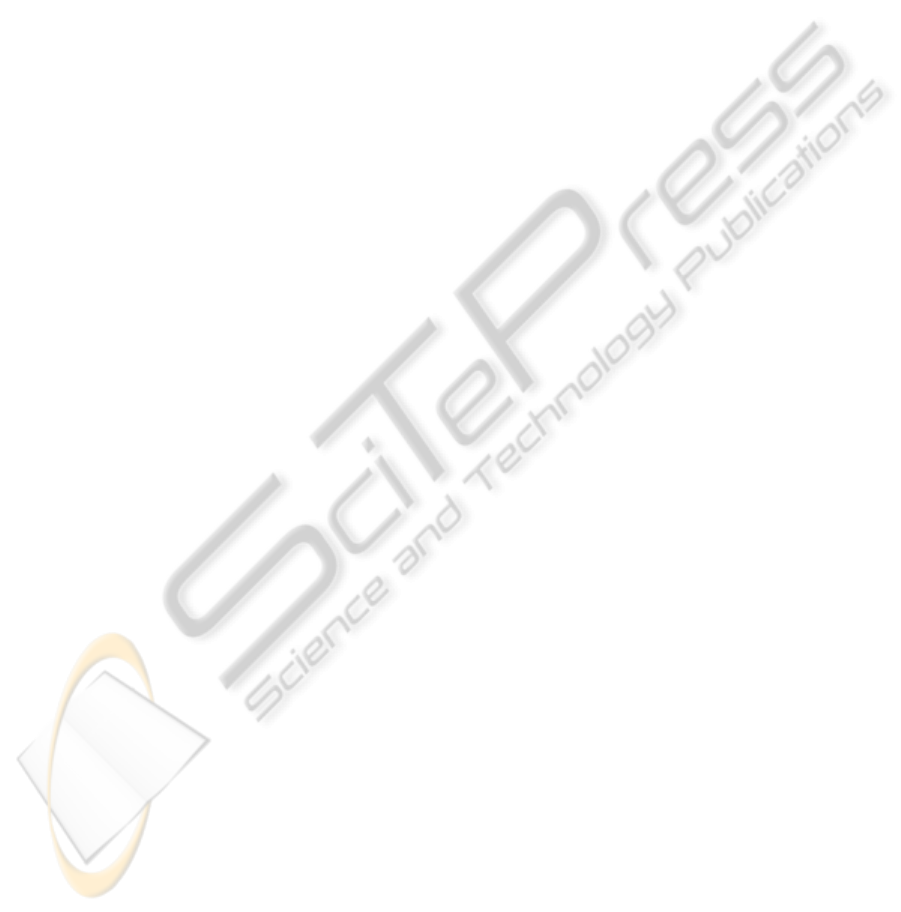
behavior in housing with regards to his energy con-
sumption. The proposed system builds a set of pro-
files from the IRISE databases for each appliance. A
profile is defined by the probability that the associated
service starts, the average consumed energy and the
average duration. Also, each profile is characterized
by the set of days and the set of months during which
the consumption is specific. A questionnaire is pro-
posed to the user concerning the use of its appliances.
The comparison between the response of the user and
the set of standard profiles allows to provide start-
ing standard profiles to the Home Automation System.
These values are introduced into a Bayesian Network
to be adjusted with the actual consumption of the user.
Future works will be dedicated to perform the seg-
mentation to the IRISE data in order to identify the
standard profiles. Then a questionnaire will be de-
fined and the way how to process the comparison be-
tween the response and the standard profiles will be
addressed.
REFERENCES
Abras, S., Ploix, S., Pesty, S., and Jacomino, M. (2007).
A multi-agent design for a home automation system
dedicated to power management. In Proceedings of
the IFIP Conference on Artificial Intelligence Appli-
cations and Innovations, Athen, Greece. Springer.
Abras, S., Ploix, S., Pesty, S., and Jacomino, M. (2008). An
anticipation mechanism for power management in a
smart home using multi-agent systems. In ICTTA’08:
Proceedings of the 3rd International Conference on
Information and Communication Technologies: from
Theory to Applications, pages 110–116, Damascus,
Syria. IEEE Computer Society.
Barco, R., Nielsen, L., Guerrero, R., Hylander, G., and
Patel, S. (2002). Automated troubleshooting of a
mobile communication network using bayesian net-
works. Mobile and Wireless Communications Net-
work, 2002. 4th International Workshop on, pages 606
– 610.
Becker, A., Geiger, D., Schffer, A. A., and Schaffer, A. A.
(1998). Automatic selection of loop breakers for ge-
netic linkage analysis. Human Heredity, 48:49–60.
Ezawa, K. and Schuermann, T. (1995). Fraud/uncollectible
debt detection using a bayesian network based learn-
ing system: A. In Proceedings of the 11th Annual
Conference on Uncertainty in Artificial Intelligence
(UAI-95), pages 157–16, San Francisco, CA. Morgan
Kaufmann.
HA, L., Ploix, S., Zamai, E., and Jacomino, M. (2006).
A home automation system to improve the household
energy control. In In INCOM2006 12th IFAC Sympo-
sium of Information Control Problems in Manufactur-
ing, Saint Etienne, France.
Ha, S., Jung, H., and Oh, Y. (2006). Method to analyze user
behavior in home environment. Personal Ubiquitous
Comput., 10(2-3):110–121.
Ha, D. L.; Ploix, S. Z. E. . J. M. (2005). Control of en-
ergy consumption in home automation by ressource
constraint scheduling. In The 15th International Con-
ference on Control System and ComputerScience.
Hart, P. E. and Graham, J. (1997). Query-free information
retrieval. IEEE Intelligent Systems, 12(5):32–37.
Heckerman, D. (1995). A tutorial on learning bayesian
networks. Technical report, Communications of the
ACM.
Horvitz, E. and Barry, M. (1995). Display of information
for time-critical decision making. In In Proceedings of
the Eleventh Conference on Uncertainty in Artificial
Intelligence, pages 296–305. Morgan Kaufmann.
Na¨ım, P., Wuillemin, P.-H., Leray, P., Pourret, O., and
Becker, A. (2004). R´eseaux bay´esiens. Eyrolles, Paris.
Palensky, P., Dietrich, D., Posta, R., and Reiter, H. (1997).
Demand side management in private homes by us-
ing lonworks. Vortrag: WFCS97 2nd IEEE Work-
shop on Factory Communication Systems, Barcelona,
pages 341 – 347.
Pearl, J. (1986). Fusion, propagation, and structuring in
belief networks. Artif. Intell., 29(3):241–288.
Russell, S. J. and Norvig, P. (2003). Artificial Intelligence:
A Modern Approach. Pearson Education.
Wood, G. and Newborough, M. (2003). Dynamic energy-
consumption indicators for domestic appliances: en-
vironment, behaviour and design. Energy and Build-
ings, pages 821–841.
Wood, G. and Newborough, M. (2007). Influencing user be-
haviour with energy information display systems for
intelligent homes. International journal of energy re-
search, vol. 31, no1:56–78.
Zaane, O. R. (1999). Principles of Knowledge Discovery in
Databases - Chapter 8: Data Clustering.
Zimmerman, G. (2007). Modeling and simulation of in-
dividual user behavior for building performance pre-
dictions. In SCSC: Proceedings of the 2007 summer
computer simulation conference, pages 913–920, San
Diego, CA, USA. Society for Computer Simulation
International.
SMART HOME - From User's Behavior to Prediction of Energy Consumption
153