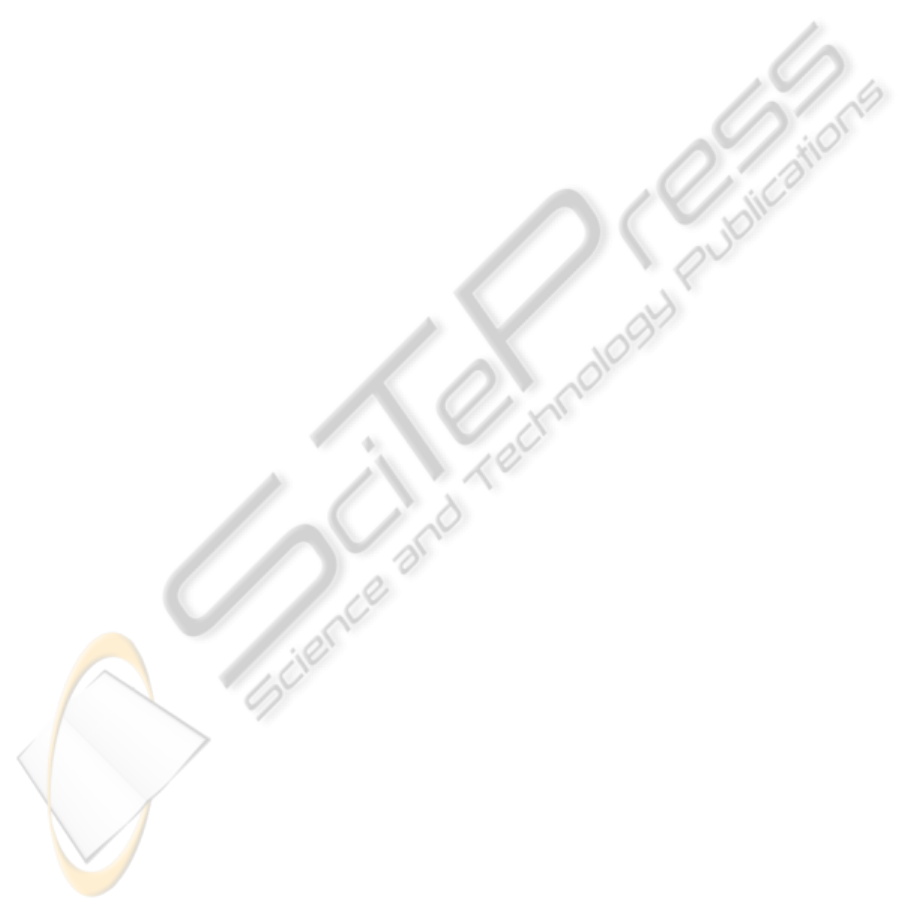
A Crossing Network(Γ) is a graph whose nodes
are Crossing Network. Edges represent spatial ad-
jacency relations between two nodes described in
SpaceOntology giving a contact point between these
nodes, known as gateways. A gateway (i.e door, pass-
ing lane, . . . ) allows transitions between adjacent
spaces and between spaces adjacent in different hier-
archical levels. Edges are labeled by a couple (pass,
dist), where pass gives the gateway connecting these
regions (or networks) and dist is the distance sep-
arating these regions (or networks) passing through
this gateway. There are two types of crossing net-
works: (1) Low-level crossing Networks are crossing
networks whose nodes are crossing networks. It used
such a network mainly as we have not reached the
level of specialization wanted (or fixed). (2) High-
level crossing Networks are crossing networks whose
nodes are the regions. It used when level of special-
ization desired (or fixed) is reached.
The construction of Crossing Network Graph is
done from the abstract level to fixed detailed level.
First, we consider the target zone of the most abstract
level. In the same way, we consider the initial zone
of the most abstract level in the ontology. We select
the most abstract target zone and initial zone targeted
areas as these two zones are included in the same re-
gion. For instance, we consider the third floor (target
zone) and the first floor (initial). It requires setting
different gateways allowed to exit the initial zone and
enter to the target zone. From the spatial relationships
of adjacency defined SpaceOntology, we can find with
backward mechanism all possible paths to reach the
initial region.
6 CONCLUSIONS
This paper presents a spatial representation using an
ontology allowing us to represent and reason on spa-
tial objects represented from different point of views
(human and robot). Future work will concern to in-
tegrate it in planning by extending the planning lan-
guage PDDL. This is an innovative concept. In this
paper, several aspects are still cause for thought as the
assessment of a relationship without a fixed target or
the implementation of an algorithm to generate a path
according to some optimality criteria. These points
will be the subject of future work.
REFERENCES
Allen, J. (1983). Maintaining knowledge about temporal
intervals.
Baader, F., Calvanese, D., McGuinness, D., Patel-
Schneider, P., and Nardi, D. (2003). The description
logic handbook: theory, implementation, and applica-
tions. Cambridge Univ Pr.
Balbiani, P., Condotta, J.-F., and del Cerro, L. F. (1999).
A new tractable subclass of the rectangle algebra. In
IJCAI, pages 442–447.
Bloch, I. (2005). Fuzzy spatial relationships for image pro-
cessing and interpretation: a review. Image and Vision
Computing, 23:89–110.
Bloch, I. and Ralescu, A. (2003). Directional relative posi-
tion between objects in image processing: a compari-
son between fuzzy approaches. Pattern Recognition.
Casati, R., Smith, B., and Varzi, A. (1998). Ontological
tools for geographic representation. (FOIS’98).
Dasiopoulou, S., Mezaris, V., Kompatsiaris, I., Papastathis,
V., and Strintzis, M. (2005). Knowledge-assisted se-
mantic video object detection. IEEE Transactions on
Circuits and Systems for Video Technology.
Dominey, P., Boucher, J., and Inui, T. (2004). Building
an adaptive spoken language interface for perceptu-
ally grounded human–robot interaction. In Proceed-
ings of the IEEE-RAS/RSJ international conference on
humanoid robots.
Fern
´
andez-Madrigal, J., Galindo, C., and Gonz
´
alez, J.
(2004). Assistive navigation of a robotic wheelchair
using a multihierarchical model of the environment.
Integrated Computer-Aided Engineering.
Gruber, T. et al. (1995). Toward principles for the design of
ontologies used for knowledge sharing. International
Journal of Human Computer Studies.
Hudelot, C. (2005). Towards a cognitive vision platform
for semantic image interpretation; application to the
recognition of biological organisms.
Hudelot, C., Atif, J., and Bloch, I. (2008). Fuzzy spatial
relation ontology for image interpretation. Fuzzy Sets
and Systems.
Kuipers, B. (2000). The spatial semantic hierarchy. Artifi-
cial Intelligence, 119:191–233.
Kuipers, B. and Levitt, T. (1988). Navigation and mapping
in large scale space. AI magazine, 9:25.
Laborie, S., Euzenat, J., and Layaıda, N. (2006). A spatial
algebra for multimedia document adaptation. SMAT.
McGuinness, D., Van Harmelen, F., et al. (2004). OWL web
ontology language overview. W3C recommendation.
Schockaert, S. (2008). Reasoning about fuzzy temporal and
spatial information from the web. PhD thesis, Ghent
University, 2008.
A SPATIAL ONTOLOGY FOR HUMAN-ROBOT INTERACTION
159