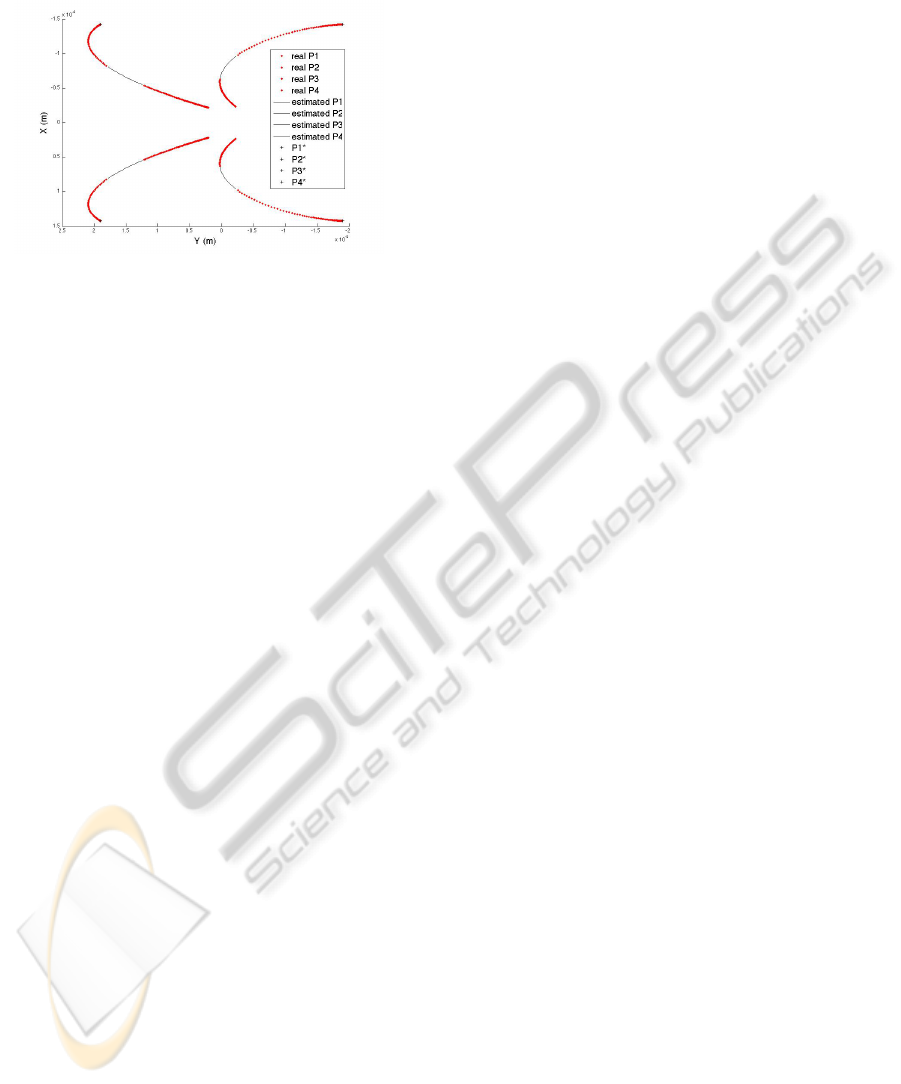
rithm accuracy is then significantly improved thanks
to our approach.
Figure 4: Evolution of estimated and real visual features.
5 CONCLUSIONS
In this paper, we have presented a method allowing
to estimate the depth z
i
during a vision-based navi-
gation task. The proposed approach relies on a pre-
dictor/estimator pair able to provide an estimation of
z
i
, even when the visual data are noisy. The advan-
tage of the proposed approach is that it relies on a
parameterizable number of images, which can be ad-
justed depending on the computation abilities of the
considered processor. The reconstructed depth value
is then used to feed Folio’s algorithm, increasing its
accuracy. The obtained results have proven the ef-
ficiency of our technique in a noisy context. Up to
now, we have only used the estimated value of z
i
to
improve Folio’s work. In the future, we plan to ben-
efit from this value at two different levels. The first
one concerns the control law design with the compu-
tation of L
(s,z)
. The approximations classically made
in the visual servoing area could then be overcome.
The second level is related to the determination of the
reference visual signals s
∗
. This term is computed ei-
ther experimentally by taking an image at the desired
position or theoretically by means of models. These
solutions significantly reduce autonomy. We believe
that a precise estimation of the depth can be very help-
ful to automatically on-line compute the value of s
∗
,
suppressing the above mentioned drawbacks. Finally,
another challenging aspect of our future work will
consist experimenting our approach on a real robot.
REFERENCES
Cervera, E., Martinet, P., and Berry, F. (2002). Robotic
manipulation with stereo visual servoing. Robotics
and Machine Perception, SPIE International Group
Newsletter, 1(1):3.
Chaumette and Hutchinson (2006). Visual servo control,
part 1 : Basic approaches. IEEE Robotics and Au-
tomation Magazine, 13(4).
Comport, Pressigout, Marchand, and Chaumette (2004).
Une loi de commande robuste aux mesures aberrantes
en asservissement visuel. In Reconnaissance des
Formes et Intelligence Artificielle, Toulouse, France.
Corke, P. (1996). Visual control of robots : High perfor-
mance visual servoing. Research Studies Press LTD.
De Luca, Oriolo, and Giordano (2008). Features depth
observation for image based visual servoing: theory
and experiments. Int. Journal of Robotics Research,
27(10).
Djeridane (2004). Sur la commandabilit
´
e des syst
`
emes non
lin
´
eaires
`
a temps discret. PhD thesis, Universit
´
e Paul
Sabatier - Toulouse III.
Durand Petiteville, A., Courdesses, M., and Cadenat, V.
(2009). Reconstruction of the features depth to im-
prove the execution of a vision-based task. In 9th In-
ternational workshop on Electronics, Control, Mod-
elling, Measurement and Signals 2009, Mondragon,
Spain.
Espiau, Chaumette, and Rives (1992). A new approach to
visual servoing in robotics. IEEE Trans. Robot. Au-
tomat., 8:313–326.
Folio and Cadenat (2008). Computer Vision, chapter 4.
Xiong Zhihui; IN-TECH.
Jerian, C. and Jain, R. (1991). Structure from motion: a
critical analysis of methods. IEEE Transactions on
systems, Man, and Cybernetics, 21(3):572–588.
Ma, Y., Soatto, S., Kosecka, J., and Sastry, S. (2003). An in-
vitation to 3-D vision: from images to geometric mod-
els. New York: Springer-Verlag.
Matthies, Kanade, and Szeliski (1989). Kalman filter-based
algorithms for estimating depth in image sequences.
Int, Journal of Computer Vision, 3(3):209–238.
Pissard-Gibollet and Rives (1995). Applying visual ser-
voing techniques to control a mobile handeye sys-
tem. In IEEE Int., Conf. on Robotics and Automation,
Nagoya, Japan.
Samson, Borgne, and Espiau (1991). Robot control : The
task function approach. Oxford science publications.
A NEW PREDICTOR/CORRECTOR PAIR TO ESTIMATE THE VISUAL FEATURES DEPTH DURING A
VISION-BASED NAVIGATION TASK IN AN UNKNOWN ENVIRONMENT - A Solution for Improving the Visual
Features Reconstruction During an Occlusion
273