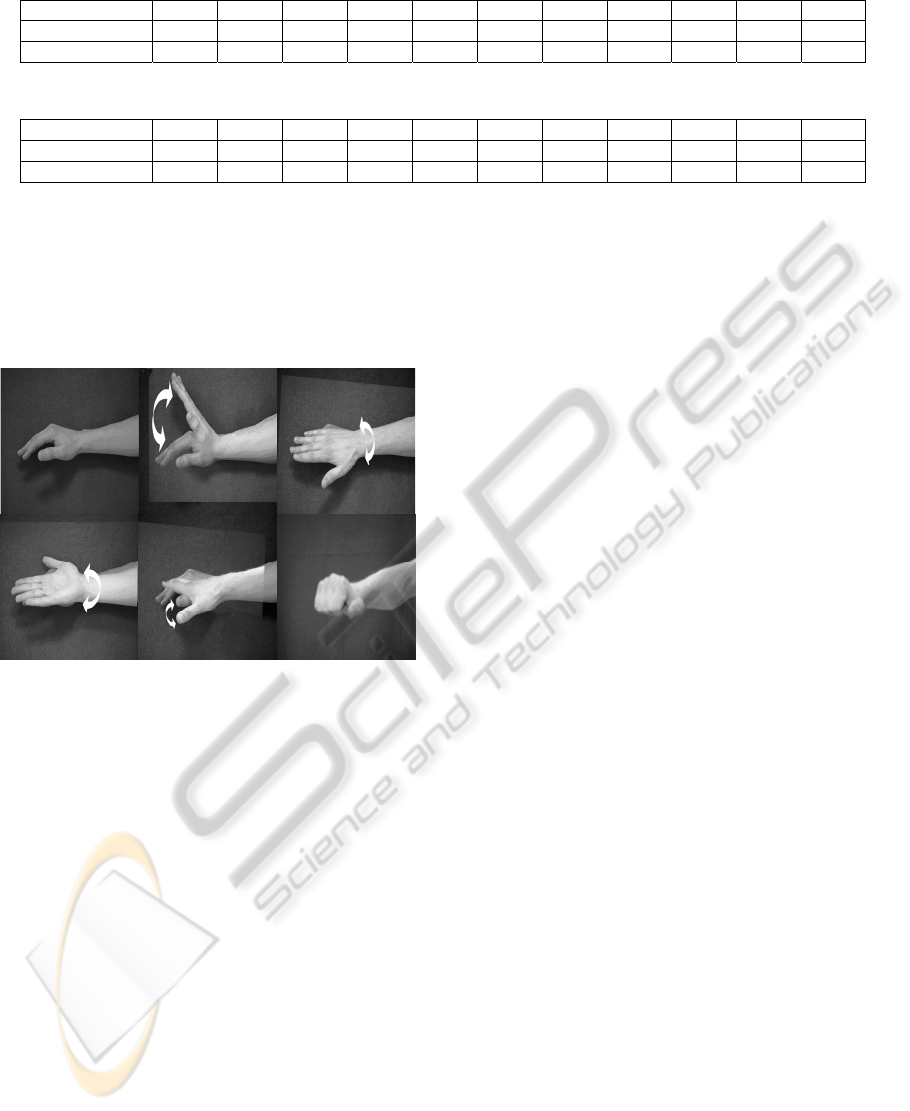
Table 1: Averaged classification errors of the tested movements for FFT input vector (Err
1
-DT, Err
2
-NNTree).
Class 1 2 3 4 5 6 7 8 9 10 11
Err
1
[%] 13 16 14 19 17 12 18 23 19 17 19
Err
2
[%] 9 14 14 17 9 10 13 21 12 11 14
Table 2: Averaged classification errors of the tested movements for DWT input vector (Err
1
-DT, Err
2
-NNTree).
Class 1 2 3 4 5 6 7 8 9 10 11
Err1 [%] 12 14 19 17 12 13 15 18 17 18 15
Err2 [%] 6 11 11 13 13 7 14 16 10 8 8
frequency 1 kHz). The data were located in the SQL
database. The measurement data (1/3 of the signals
selected at random) were used to generate the
decision tree and MLPs. The remaining part of the
set (2/3 of the signals selected at random) was
assigned as a testing set.
Figure 11: Tested movement classes.
The NNTree generated in this way gave an
average recognition value equal to 87% (FFT) when
the DT with classical nodes in turn gave a value
equal to 83% (FFT). The detail results for tested
movements are presented in Table1; were Err1 and
Err2 represent the errors obtained respectively by
DT and by NNTree methods for the FFT feature
vectors. As we can see the best results were for
classes 1, 5, 6 and 10.
In the case of DWT feature vectors an average
recognition values were equal to 89% for NNTree
and 85% for DT method. I this case the best results
were for classes 1, 6, 10 and 11 (see: Table 2).
5 CONCLUSIONS
The tests with the presented “EMG Analysis”
software are in the incipient stage. The conducted
experiments aimed on principle at comparing the
features extraction methods for EMG signals and
their further classification. They prove the
usefulness of the presented multistage analysis
methods for the recognition of the decisions that
control the manipulation and grasping movements of
the artificial hand.
REFERENCES
Wolczowski A., 2001. Smart hand: the concept of sensor
based control. In MMAR’01, 7th IEEE International
Conference on Methods and Models in Automation
and Robotics. Szczecin Univ. of Technol. Publishing.
Su Y., Fisher M. H., Wolczowski A., Bell D. G., Burn D.
J., Gao R. X., 2007. Towards and EMG-controlled
prosthetic hand using a 3-D electromagnetic
positioning system. IEEE Transactions on
Instrumentation and Measurement, (56/1).
Mohammadreza A. O., 2007. Myoelectric control system
– A survey. Biomedical Signal Processing and
Control, vol. 2, issue 4, pp 275-294.
Krysztoforski, K., Wolczowski, A., 2005. Progress of
Robotics - Industrial and medical robotic systems,
A laboratory stand for EMG signal measurements.
WKL. Warszawa.
De Luca, C. J., 2006. Electromyography. Encyclopedia of
Medical Devices and Instrumentation. (John G.
Webster, Ed.) John Wiley Publisher.
Wolczowski, A., Suchodolski T., 2007. Bioprosthesis
Control: Human-Machine Interaction Problem. In
Challenges for Assistive Technology - AAATE 07. IOS
Press.
Wojtczak P., Amaral T. G., Dias O. P., Wolczowski A.,
Kurzynski M., 2008. Hand movement recognition
based on biosignal analysis. Engineering Applications
of Artificial Intelligence, (22/(4/5)).
Mallat, S., 1989. A Theory for Multiresolution Signal
Decomposition, IEEE Trans. Pattern Analysis and
Machine Intelligence, (11).
Zhao, Q. F., 2001. Evolutionary design of neural network
tree – integration of decision tree, neural network and
GA. In IEEE Congress on Evolutionary Computation.
Quinlan, J. R., 1993. C4.5: Programs for Machine
Learning, Morgan Kaufmann. San Mateo, California.
ICINCO 2010 - 7th International Conference on Informatics in Control, Automation and Robotics
326