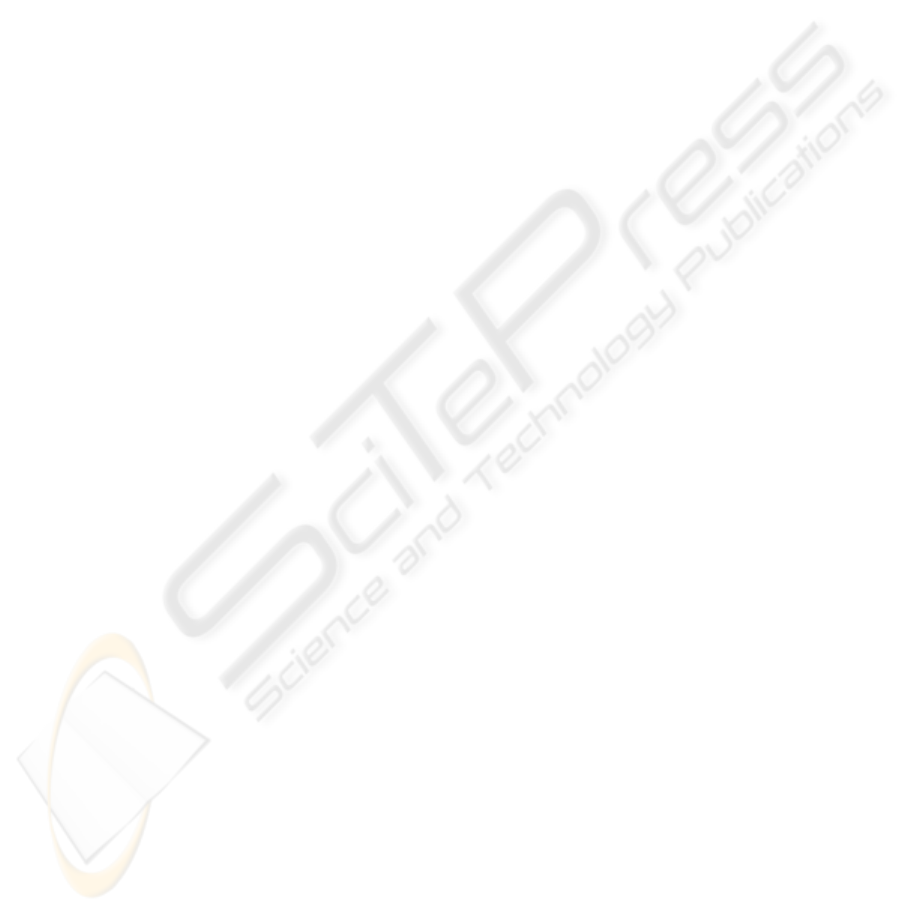
with an application on a very complex real world pro-
cess (a blast furnace) are presented to show the opera-
tional character of the TOM4L process. These results
provide new insights about the blast furnace behavior.
So our current works are now focusing on the defini-
tion of a verity principle that is required to qualified
the discovered relations.
REFERENCES
Agrawal, R. and Psaila, G. (1995). Active data mining.
In Fayyad, Usama, M. and Uthurusamy, R., editors,
First International Conference on Knowledge Discov-
ery and Data Mining (KDD-95), pages 3–8, Montreal,
Quebec, Canada. AAAI Press, Menlo Park, CA, USA.
Ayres, J., Flannick, J., Gehrke, J., and Yiu, T. (2002). Se-
quential pattern mining using a bitmap representation.
KDD02: Proceedings of the eighth ACM SIGKDD in-
ternational conference on Knowledge discovery and
data mining, pages 429–435.
Benayadi, N. and Le Goc, M. (2008). Using a measure
of the crisscross of series of timed observations to
discover timed knowledge. Proceedings of the 19th
International Workshop on Principles of Diagnosis
(DX’08).
Blachman, N. M. (1968). The amount of information that
y gives about x. IEEE Transcations on Information
Theory IT, 14.
Bouch´e, P. (2005). Une approche stochastique de
mod´elisation de s´equences d’´ev´enements discrets
pour le diagnostic des syst`emes dynamiques. Th`ese,
Facult´e des Sciences et Techniques de Saint J´erˆome.
Cover, T. M. and Thomas, J. A. (August 12. 1991). Ele-
ments of Information Theory. Wiley-Interscience.
Dousson, C. and Duong, T. V. (1999). Discovering chron-
icles with numerical time constraints from alarm logs
for monitoring dynamic systems. In IJCAI : Proceed-
ings of the 16th international joint conference on Ar-
tifical intelligence, pages 620–626.
Han, J. and Kamber, M. (2006). Data Mining: Concepts
and Techniques. Morgan Kaufmann.
Le Goc, M. (2006). Notion d’observation pour le diagnostic
des processus dynamiques: Application `a Sachem et `a
la d´ecouverte de connaissances temporelles. HDR,
Facult´e des Sciences et Techniques de Saint J´erˆome.
Le Goc, M., Bouch´e, P., and Giambiasi, N. (2006). Devs, a
formalism to operationnalize chronicle models in the
elp laboratory, usa. In DEVS’06, DEVS Integrative
M&S Symposium, pages 143–150.
Mannila, H. (2002). Local and global methods in data min-
ing: Basic techniques and open problems. 29th In-
ternational Colloquium on Automata, Languages and
Programming.
Mannila, H. and Toivonen, H. (1996). Discovering general-
ized episodes using minimal occurrences. In Knowl-
edge Discovery and Data Mining, pages 146–151.
Mannila, H., Toivonen, H., and Verkamo, A. I. (1995). Dis-
covering frequent episodes in sequences. In Fayyad,
U. M. and Uthurusamy, R., editors, Proceedings of the
First International Conference on Knowledge Discov-
ery and Data Mining (KDD-95), Montreal, Canada.
AAAI Press.
Roddick, F. and Spiliopoulou, M. (2002). A survey of tem-
poral knowledge discovery paradigms and methods.
IEEE Transactions on Knowledge and Data Engineer-
ing, 14(4):750–767.
Shannon, C. E. (1949). Communication in the presence of
noise. Institute of Radio Engineers, 37.
Smyth, P. and Goodman, R. M. (1992). An information
theoretic approach to rule induction from databases.
IEEE Transactions on Knowledge and Data Engineer-
ing 4, pages 301–316.
Vilalta, R. and Ma, S. (2002). Predicting rare events in
temporal domains. In ICDM02: Proceedings of the
2002 IEEE International Conference on Data Mining
(ICDM02), page 474. IEEE Computer Society.
Weiss, G. M. and Hirsh, H. (1998). Learning to predict rare
events in categorical time-series data. In Proceedings
of the Fourth International Conference on Knowledge
Discovery and Data Mining, AAAI Press, Menlo Park,
CA.
Zaki, M. J. (2001). Spade: An efficient algorithm for min-
ing frequent sequences. Machine Learning, 42:31–60.
ICEIS 2010 - 12th International Conference on Enterprise Information Systems
120