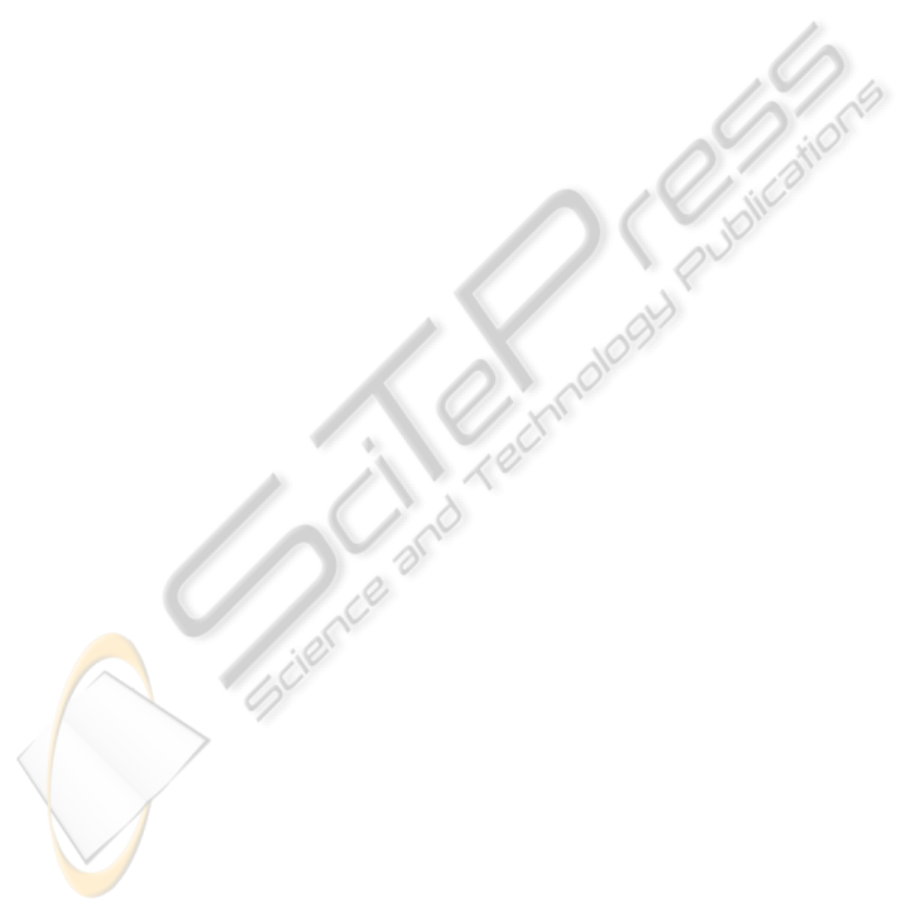
5 EXPERIMENTAL TESTING
OF CONTROL SYSTEM
The stability and robustness of the proposed control
strategy is verified by experiments on a CNC milling
machine for Ck 45 and 16MnCrSi5 XM steel
prismatic workpieces with variation of axial cutting
depth. Details of the experimental conditions and the
dimensions of the workpiece are shown in Figure 2.
Feedrates for each cut are first optimized off-line,
and then machining runs are made with controller
action. The first test is conventional cutting with the
constant feedrate (Test_A). In the second test, the
proposed control system was applied to demonstrate
its performance (Test_B). The parameters for
adaptive control are the same as for the experiments
in the conventional milling (Zuperl, 2003).
Figure 3 is the response of the cutting force and
the feedrate when the cutting depth is changed. It
shows the experimental result where the feedrate is
adjusted on-line to maintain the maximal cutting
force at the desired value.
6 RESULTS AND DISCUSSION
As compared to most of the existing end milling
control systems (Chen, 2006), the proposed adaptive
system has the following advantages: 1. the
computational complexity of UNKS does not
increase much with the complexity of process; 2. the
learning ability of UNKS is more powerful than that
of conventional adaptive controller; 3. UNKS has a
generalisation capability; 4. insensitive to changes in
workpiece geometry, cutter geometry, and
workpiece material; 5. cost-efficient and easy to
implement; and 6. mathematically modeling-free.
Comparing the Figure 3a to Figure 3b, the
cutting force for the neural control milling system is
maintained at about 250N, and the feedrate of the
adaptive milling system is close to that of the
conventional milling from point C to point D. From
point A to point C the feedrate of the adaptive
milling system is higher than for the classical CNC
system, so the milling efficiency of the adaptive
milling is improved.
The time analysis for conventional and adaptive
control system has been curried out. By adaptive
control system of time saving of 40% with one cut
was reached. The complete machining requires 15
cuts; thus machining of a simple workpiece is
shortened for 155 seconds.
The system remains stable in all experiments,
with little degradation in performance. The results
reached are in accordance with the objectives of
researches, according to which the controlled cutting
force must not deviate from the desired value for
more than 10% (Zuperl, 2005).
7 CONCLUSIONS
In this paper, an inteligent control algorithm that
controls feedrate is proposed to regulate the cutting
force. On the basis of the cutting process modeling,
off-line optimization and neural control scheme
(UNKS) the combined system for off-line
optimization and adaptive adjustment of cutting
parameters is built. This is an adaptive control
system controlling the cutting force and maintaining
constant roughness of the surface being milled by
digital adaptation of cutting parameters.
In order to check the applicability of the
adaptive control algorithm, cutting experiments were
carried out under various cutting conditions,
different tool diameters and different work materials.
Experiments have confirmed efficiency of the
adaptive control system, which is reflected in
improved surface quality and decreased tool wear.
REFERENCES
Balic, J., 2000. A new NC machine tool controller for
step-by-step milling. Int. j. adv. manuf. technol. 8, pp.
399-403.
Chen, C., Zhibin, M., (2006). An intelligent approach to
non-constant feed rate determination for high-
performance 2D CNC milling. International Journal
of Manufacturing Technology and Management. pp.
219-236.
Cus, F., Balic, J., 2003. Optimization of cutting process by
GA approach. Robot. comput. integr. manuf., 19 , pp.
113-121.
Kopac, J., 2002. Rezalne sile in njihov vpliv na
gospodarnost obdelave = Cutting forces and their
influence on the economics of machining. Stroj. vestn.
3, pp. 121-132.
Mursec, B., Cus, F., Balic, J., 2000. Organization of tool
supply and determination of cutting conditions. J.
mater. process. Technol., 100, pp. 241-249.
Zuperl, U., Cus, F., 2004. A determination of the
characteristic technological and economic parameters
during metal cutting. Stroj. vestn. pp. 252-266.
Zuperl, U., Cus, F., 2003. Optimization of cutting
conditions during cutting by using neural networks.
Robot. comput. integr. Manuf., 19 ,pp. 189-199.
Zuperl, U., Cus F., 2005. Tool cutting force modeling in
ball-end milling using multilevel perceptron. J. mater.
process. technol.
ICINCO 2010 - 7th International Conference on Informatics in Control, Automation and Robotics
268