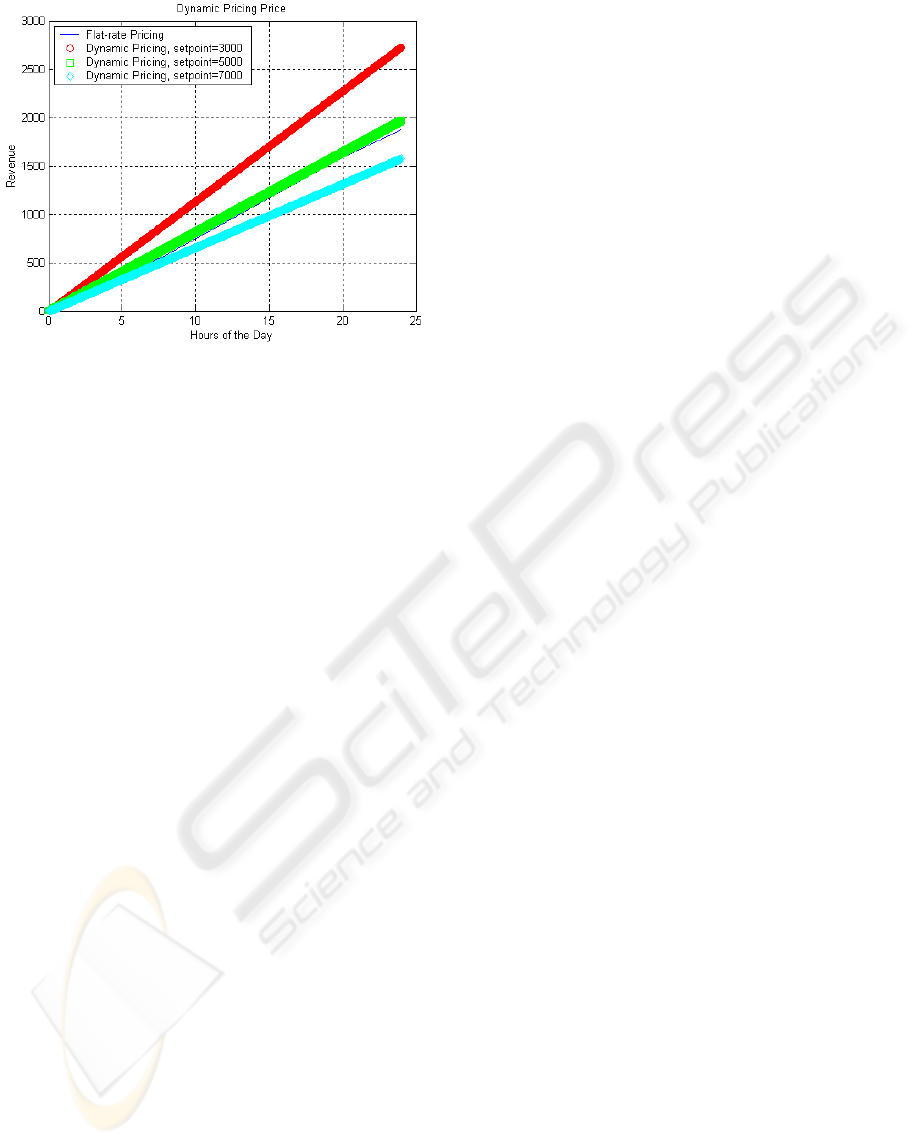
Figure 11: Revenue under Flat-rate and Dynamic Pricing.
Under the dynamic pricing scheme, new users are
always being admitted into the network as opposed
to the flat rate scheme thus, the resulting cumulative
revenue collected is higher in dynamic pricing
scheme as compared to flat rate scheme.
6 CONCLUSIONS AND FUTURE
WORK
The simulation results in Figure 9 show that an
optimal usage of the network resources is achieved
under dynamic pricing as compared to flat rate
pricing scheme. Regulating the price charged for the
network services under dynamic pricing scheme as
indicated in Figure 10, provides a mechanism to
maintain the status of the network resources at an
optimum level. In the flat rate pricing, when
congestion (due to scarcity of network resources) is
experienced in the network, new calls are blocked or
dropped resulting in high blocking probability.
While in the dynamic pricing scheme, when
congestion is experienced, the network service price
is increased thus only the users willing to pay the
new network service price will use the network. This
results in a lower blocking probability or fewer
dropped calls. By dynamically varying the network
price, the service provider provides the network
services to at a price the users are willing to pay.
The resulting better usage of the network resource
and the fewer blocked/dropped calls enables the
service provider to guarantee the quality of service
to the user, as the system ensures system availability
and reliability. The results obtained in this research
have shown that the fuzzy-based dynamic pricing
scheme can be used by the network service provider
as a mechanism to support network QoS to the users.
When the results in Figure 11 for dynamic and flat
rate pricing schemes is compared, it is observed that
total cumulative revenue collected is higher under
dynamic pricing scheme. The conclusion drawn
from these results is that implementing dynamic
scheme would result in more revenue for the
network service providers if the service provider
chooses the optimal operating point appropriately.
Dynamic pricing strategy converts congestion,
delays and queue costs into monetary values for the
service provider.
Future work includes considering that each network
service has a unique traffic and how this type of
dynamic pricing scheme can be implemented in the
real network.
REFERENCES
L. A. Zadeh, “Fuzzy Logic, Neural Networks, and Soft
Computing”, Communication of ACM, Vol. 37, No.3,
pages 77-84, March 1994.
R. Abiri, “Optimizing service Quality in GSM/GPRS
Networks,” In Focus, September 2001.
M. Bouroche, “Meeting QoS Requirements in Dynamic
Priced Commercial Cellular Network,” Masters
Thesis, University of Dublin, September 2003.
K. Ahmad, E. Fitkov-Norris, “Evaluation of Dynamic
Pricing in Mobile Communication Systems,”
University College London, 1999.
Q. Wang, J. M. Peha, M. A. Sirbu, “Optimal Pricing for
Integrated-Services Networks with Guaranteed Quality
of Service,” Carnegie Mellon University, Chapters in
Internet Economics, MIT Press, 1996.
I. C. Paschalidis, J. N. Tsitsiklis, “Congestion-dependent
Pricing of Network Services,” IEEE/ACM
Transactions on Networking, vol.8, No.2, pp.171-84,
April 2003.
J. M. Peha, “Dynamic Pricing and Congestion Control for
Best –Effort ATM services,” Computer Networks,
Vol.32, pp. 333-345, March 2000.
E. D Fitkov-Norris, A. Khanifar, “Dynamic Pricing In
Mobile Communication Systems,” In First
International Conference on 3G Mobile
communication Technologies, pp 416-420, 2000.
J. Hou, J. Yang, P. Symeon, “Integration of pricing and
call admission for wireless networks,” In IEEE 54
th
Vehicular Technology Conference, Vol. 3, pp 1344-
1348, 2001.
E. Viterbo, C. F. Chiasserini, “Dynamic Pricing for
Connection Oriented Services in Wireless Networks,”
In 12
th
IEEE International Symposium on Personal,
Indoor and Mobile Radio Communications, Vol.1, pp.
A-68-72, September 2001.
FUZZY LOGIC BASED DYNAMIC PRICING SCHEME FOR PROVISION OF QOS IN CELLULAR NETWORKS
73