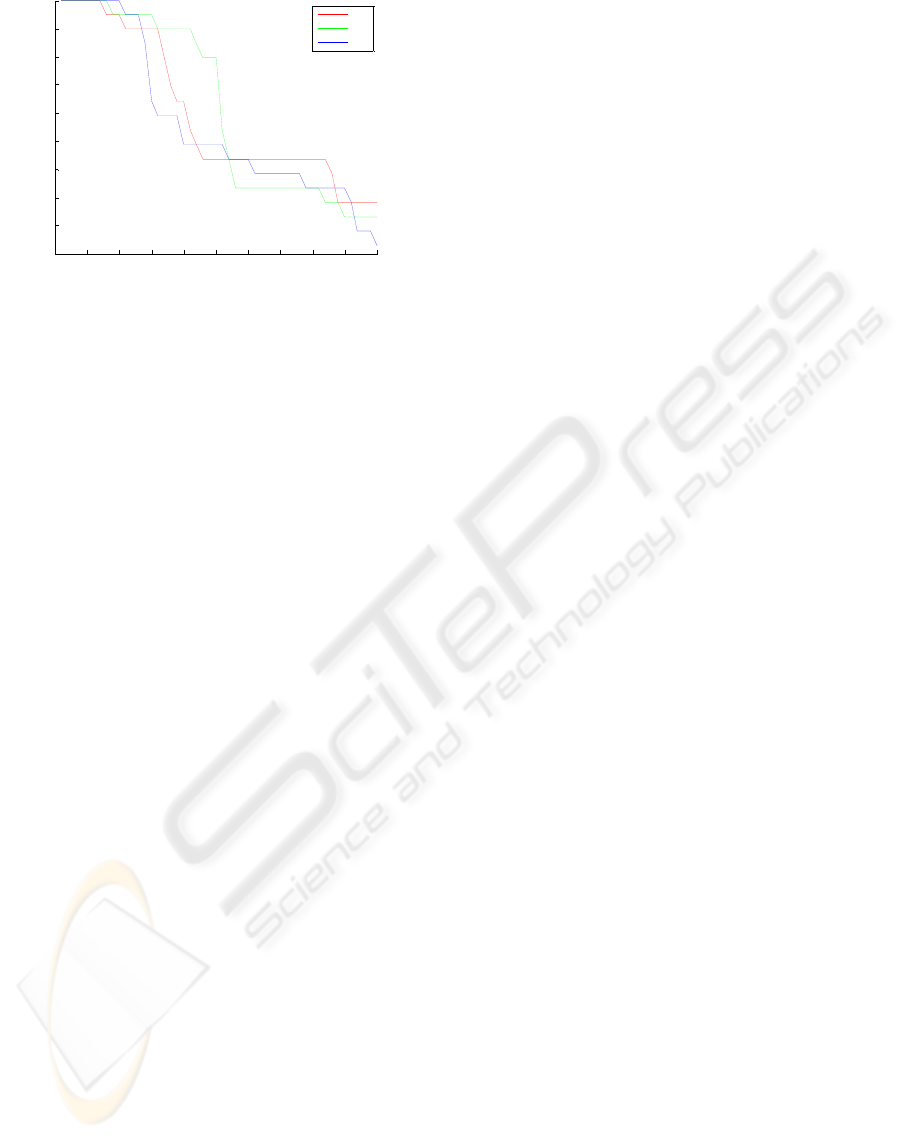
0 0.5 1 1.5 2 2.5 3 3.5 4 4.5 5
0.55
0.6
0.65
0.7
0.75
0.8
0.85
0.9
0.95
1
Sigma values of RBF Kernel
Specificity Value
CV1
CV2
CV3
Figure 3: The specificity values of SVM.
4 CONCLUSIONS
In this study, the statistical data of HIV subtype
genes were obtained by accessible residues and
modeled by AR model to reduce the size of HIV
sequences. The SVM structure was used to classify
HIV sub-type viruses successfully. Thus, the
optimal parameter
in radial basis kernel of SVM
was searched by using the pre-processed data.
The training and test dataset were obtained by
using 3-fold cross-validation and these datasets were
used for training and testing the SVM.
The best classification accuracy was obtained
while the parameter
was 0.1 for all CVs.
Moreover, as the parameter
was increasing, the
accuracy levels were decreasing.
Since the classification accuracy is not enough to
analyze the performance of SVM, ROC analysis was
applied to these results. The sensitivity and
specificity were obtained as 1, when the parameter
was 0.1 for all CVs.
In future work, SVM structure and an
incremental Multilayer Perceptron implementation
will be compared and the results will be discussed.
REFERENCES
Berger, E. A., Murphy, P. M., and Farber, J. M. (1999)
Chemokine Receptors as HIV-1 Coreceptors: Roles in
Viral Entry, Tropism, and Disease. Ann. Rev.
Immunology. 17, 675-700.
Resch, W., Hoffman, N., and Swanstrom, R. (2001).
Improved Success of Phenotype Prediction of the
Human Immunodeficiency Virus Type 1 from
Envelope Variable Loop 3 Sequence Using Neural
Networks. Journal of Virology. 76, 3852-3864.
Wang, D., and Larder, B. (2003). Enhanced Prediction of
Lopinavir Resistance from Genotype by Use of
Artificial Neural Networks. J. Infectious Diseases.
188, 653-660.
Brumme, Z. L., Dong, W. W. Y., Yip, B., Wynhoven, B.,
Hoffman, N. G., Swanstrom, R., Jensen, M. A.,
Mullins, J. I., Hogg, R. S., Montaner, J. S. G., and
Harrigan, P. R. (2004). Clinical and Immunological
Impact of HIV Envelope V3 Sequence Variation after
Starting Initial Triple Antiretroviral Therapy. AIDS.
18, F1-F9.
Milich, L., Margolin, B., and Swanstrom, R. (1993). V3
Loop of the Human Immunodeficiency Virus Type 1
Env Protein: Interpreting Sequence Variability. J.
Virology. 67(9), 5623-5634.
Lamers, S., Susanna, L., Salemi, M., McGrath, M. S., and
Fogel, G. B. (2008). Prediction of R5, X4, and R5X4
HIV-1 Coreceptor Usage with Evolved Neural
Networks. Trans. On 1HComputational Biology and
Bioinformatics. 5, 291-300.
Zhou, H., and Yan, H. (2006). Autoregressive Models for
Spectral Analysis of Short Tandem Repeats in DNA
Sequences. IEEE Int. Conf. on Systems, Man and
Cybernetics. 2, 1286-1290.
Kong, R., Wang, C. X., Ma, X. H., Liu, J. H., and Chen,
W. Z. (2005). Peptides Design Based on the Interfacial
Helix of Integrase Dimer. 27th Annual Int. Conf. of the
Engineering in Medicine and Biology Society. 4743-
4746.
Akhtar, M., Ambikairajah, E., and Epps, J. (2007).
Detection of period-3 behavior in genomic sequences
using singular value decomposition. Proc. of
International Conference on Emerging Technologies.
13-17.
Rosen, G. (2007). Comparison of Autoregressive
Measures for DNA Sequence Similarity. IEEE
Genomic Signal Processing and Statistics Workshop
(GENSIPS). 13-17.
Haykin, S. (2002). Adaptive Filter Theory, New Jersey:
Prentice-Hall.
Kohavi, R. (1995). A study of cross-validation and
bootstrap for accuracy estimation and model selection.
Proceedings of the Fourteenth International Joint
Conference on Artificial Intelligence. 2, 1137–1143.
Vapnik, V. N. (1999). An overview of statistical learning
theory. IEEE Transactions on Neural Networks. 10(5),
988-999.
SIGMAP 2010 - International Conference on Signal Processing and Multimedia Applications
166