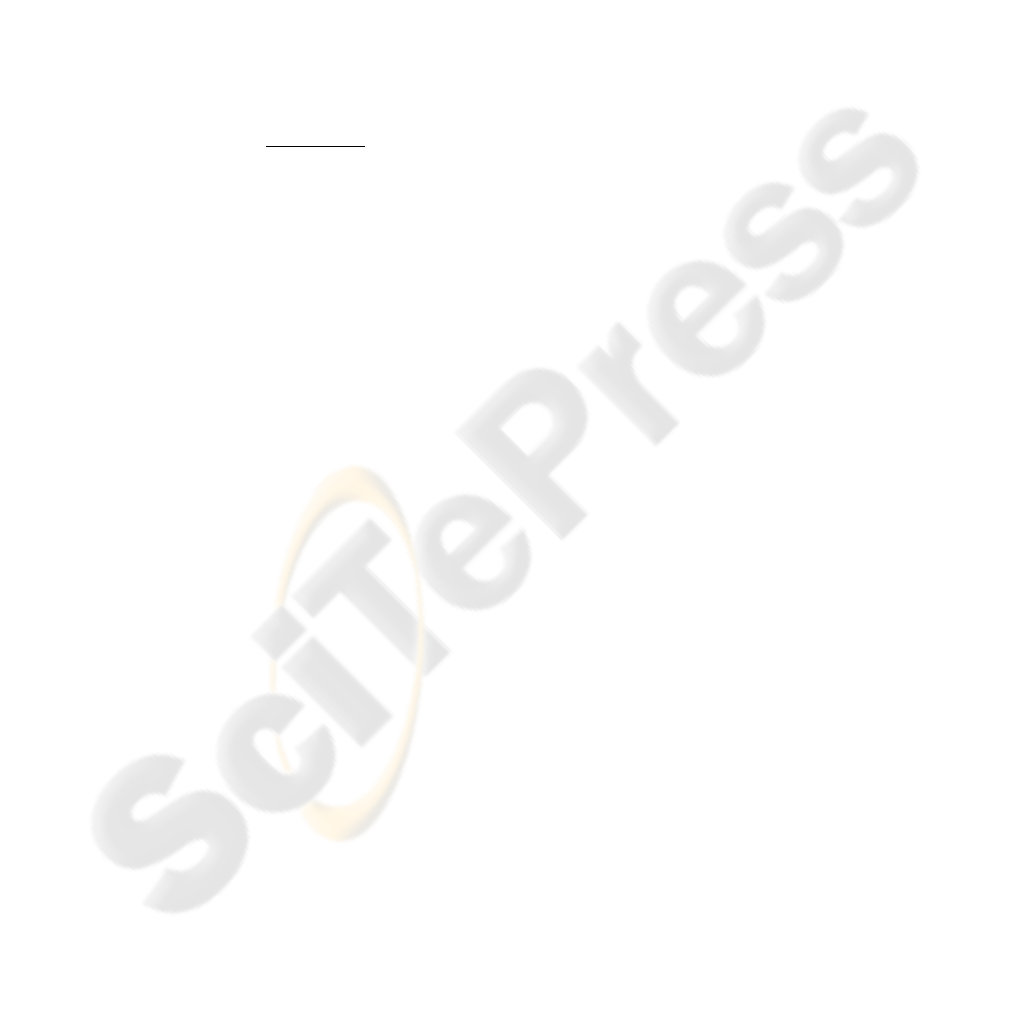
From Eq. 2 and 3, when the eye closeness is less than
or equal to 20% of the maximum distance between
eyelids is considered that the eyes are closed. Accord-
ing to the work developed in (Dong and Wu, 2005) if
the eyes are closed for 5 consecutive frames it can be
considered that the driver is falling asleep.
In order to compute the rate of mouth opening, it
is necessary to know the degree of the mouth open-
ness, which is represented by the relation between the
mouth’s height and width. The graphical represen-
tation of the mouth openness in a period of time is
know as YawnFrec. To compute the mouth openness
the vertices (right, left, upper, lower), of the mouth
in the model Candide 3 are used. In this sense, the
mouth openness is computed as follows:
OpenMouth =
d (v
7
− v
8
)
d (v
64
− v
31
)
(4)
5 EXPERIMENTAL
FRAMEWORK
Two different videos were acquired with 320x340 res-
olution. The subjects were instructed to blink and
yawn in different head positions range from 45
◦
y −
45
◦
. The subject 1 blinked 35 times and yawned 5
times. The subject 2 blinked 24 and yawned 4 times.
The system was able to identified the total numbers
of yawns and blinks for each subject. This means
a 100% accuracy in detection of eye closeness and
mouth openness. Indeed the parameters PERCLOS,
AECS, and Yawnfrec could be determined every time.
In order to validate the presented methodology, an ex-
perimental framework is proposed. First, a commer-
cial driving simulator is going to be used and three
diferent physiological test will be set up (the PVT test,
the Stroop test, and the RT test). These tests are em-
bedded in the software PEBL (Open source Psychol-
ogy Software), available at “http://pebl.sf.net”. From
these tests some basic measures will be made, in sim-
ilar ambiental and physical conditions to those pre-
sented in the computer-vision-based methodology in
order to compare results and validate the parameters.
6 CONCLUSIONS
In this paper was proposed a characterization method-
ology based on models to assess fatigue. Two vari-
ables are measured by means of computer vision. The
eye closening range and the mouth opening range.
These measures are the base to calculate the param-
eters PERCLOS, AECS y YawnFrec. It has been
found that the proposed methodology is practical and
reliable whithin the previously described conditions.
Also an experimental framework based on psycholog-
ical measures was defined in order to validate the pro-
posed methodology. As future work, it is proposed to
complement the above methodology with estimation
of head’s position in order to compute additional pa-
rameters. This will provide more information to the
assessment of the driver fatigue.
REFERENCES
Ahlberg, J. (2001.). Candide-3 an updated parameterized
face. Report No. LiTH-ISY-R-2326, Dept. of Electri-
cal Engineering, Linkping University, Sweden.
Cootes, T. F., Taylor, C. J., Cooper, D. H., and Graham, J.
(1995). Active shape models-their training and appli-
cation. Comput. Vis. Image Underst., 61(1):38–59.
Dinges, D. F., Mallis, M., Maislin, G., and Powell, J. W.
(1998). Evaluation of techniques for ocular measure-
ment as an index of fatigue and the basis for alertness
management.
Dong, W. and Wu, X. (2005). Driver fatigue detection based
on the distance of eyelid. IEEE Int. Workshop VLSI
Design and Video Tech Suzhou, pp 365-368.
Ji, Q. and Yang, X. (2002). Real-time eye, gaze, and face
pose tracking for monitoring driver vigilance. Real-
Time Imaging, 8(5):357–377.
Saeed, I., Wang, A., Senaratne, R., and Halgamuge, S.
(2007). Using the active appearance model to detect
driver fatigue. In Information and Automation for Sus-
tainability, 2007. ICIAFS 2007. Third International
Conference on, pages 124 –128.
Saroj, K. and Lal, A. (2001.). A critical review of the psy-
chophysiology of driver fatigue. Biological Psychol-
ogy 55 173194.
Smith, P., Shah, M., and Vitoria, N. (2003.). Determining
driver visual attention with one camera. Trans. on In-
telligent Transportation Systems, 4(4):205–218.
Ting, P., Hwang, J., Doong, J., and Jeng, M. (2008.). Driver
fatigue and highway driving: A simulator study. Phys-
iology and Behavior 94 448-453.
Vural, E., Cetin, M., Ercil, A., Littlewort, G., Bartlett, M.,
and Movellan, J. (2007). Drowsy driver detection
through facial movement analysis. pages 6–18.
Wang, Q., Yang, J., Ren, M., and Zheng, Y. (2006). Driver
fatigue detection: A survey. Proceedings 6th World
Congr. Intell. Control Autom., Dalian, China, pp.
8587-8591.
Zhang, Z. and Zhang, J. (2006). Driver fatigue detection
based intelligent vehicle control. In ICPR ’06: Pro-
ceedings of the 18th International Conference on Pat-
tern Recognition, pages 1262–1265.
Zhu, Z., Ji, Q., and Lan, P. (2004). Real time non-intrusive
monitoring and prediction of driver fatigue. IEEE
Trans. Veh. Technol, 53:1052–1068.
ICINCO 2010 - 7th International Conference on Informatics in Control, Automation and Robotics
298