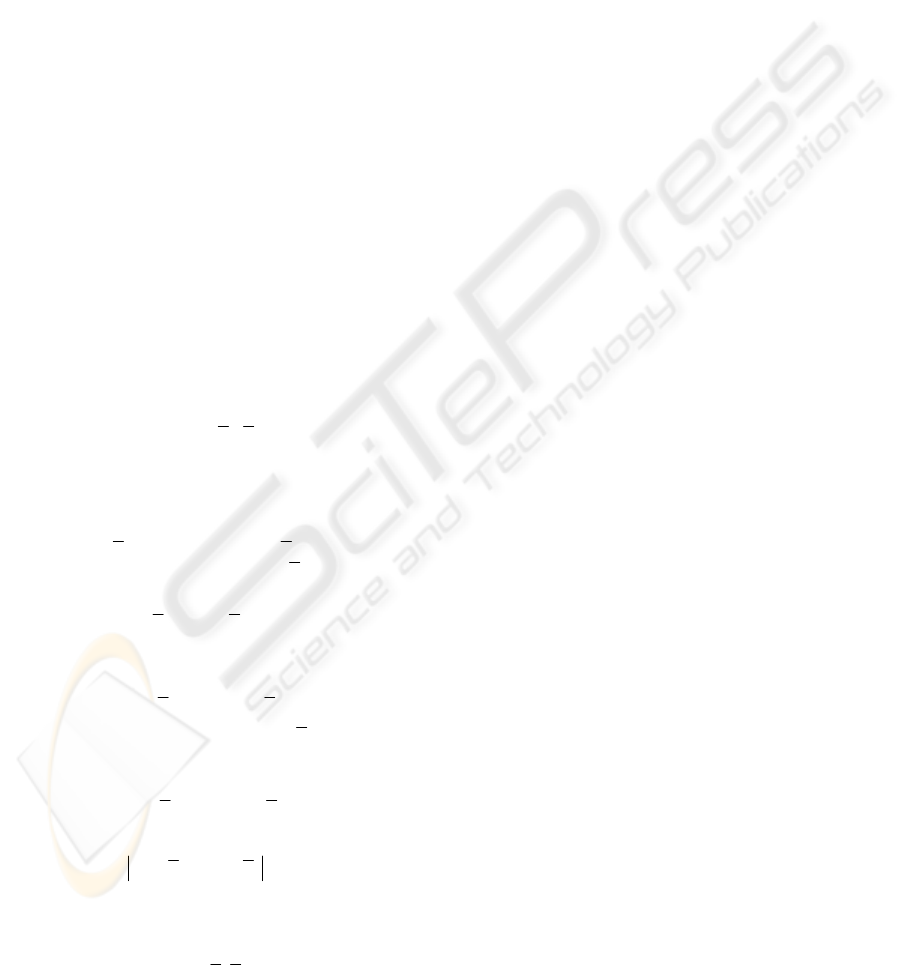
3 THE PROPSED METHOD
The basis of our method is to improve the
performance of SAX by combining it with an
exclusion condition that increases its pruning power,
and by using different reduced spaces, and not only
one.
We divide each time series into
N
segments.
Each segment is approximated by a polynomial. In
this paper we use a polynomial of the first-degree for
its simplicity, but other approximating functions can
be used as well.
Since this approximating function is the optimal
approximation of the corresponding segment, the
distance between this segment and this
approximating function is minimal. The time series
is also represented using SAX in the way indicated
in section 2.2. A polynomial of the same degree is
used to approximate all the segments of all the time
series in the database. So now each time series has
two representations: the first is a
n-dimensional
one, by using the approximating function, and the
second is a
-dimensional one, by using SAX. We
also have two similarity distances; the first one is the
Euclidean distance, which is the distance between a
time series and its approximating function, and the
other one is MINDIST, which is the distance in the
reduced space.
Given a query
),(
q , let u , q be the projections
of the
u , q , respectively, on their approximating
functions, where
u
is a time series in the database.
By applying the triangular inequality we get:
),(),(),( qqduqduqd +≤ (5)
Taking into consideration that
u is the best
approximation of u , we get:
),(),( quduud ≤ (6)
By substituting the above relation in (5), we can
safely exclude all the times series that satisfy:
),(),( qqduud +>
(7)
In a similar manner, and since
q
is the best
approximation of
q , we can safely exclude all the
time series that satisfy:
),(),( uudqqd +>
(8)
Both (7), (8) can be expressed in one relation:
ε
>− ),(),( qqduud (9)
In addition to the exclusion condition in (9), since
MINDIST is lower bounding of the original
Euclidean distance, all the time series that satisfy:
>),( uqMINDIST (10)
Should also be excluded, Relation (10) defines the
other exclusion condition.
The Offline Phase. The application of our method
starts by choosing the lengths of segments. We
associate each length with a level of representation.
The shortest lengths correspond to the lowest level,
and the longest lengths with the highest levels. Each
series in the database is represented by a first-degree
polynomial, which is the same approximating
function for all the time series in the databases. The
distances between the time series and their
approximating function are computed and stored. In
order to represent the time series, we choose the
alphabet size to be used. SAX appeared in two
versions; in the first one the alphabet size varied in
the interval (3:10), and in the second one the
alphabet size varied in the interval (3:20). We
choose the appropriate alphabet size for this
datasets. The time series in the database are
represented using SAX on every representation level
The Online Phase. The range query is represented
using the same scheme that was used to represent the
time series in the database. We start with the lowest
level and try to exclude the first time series using (9)
if this time series is excluded, we move to the next
time series, if not, we try to exclude this time series
using relation (10). If all the time series in the
database have been excluded the algorithm
terminates, if not, we move to a higher level. Finally,
after all levels have been exploited, we get a
potential answer set, which is linearly scanned to
filter out all the false alarms and get the true answer
set.
4 EXPERIMENTS
We conducted experiments on different datasets
available at UCR (UCR Time Series datasets) and
for all alphabet sizes, which vary between 3 (the
least possible size that was used to test the original
SAX) to 20 (the largest possible alphabet size). We
compared the speed of our method FAST_SAX,
with that of SAX as a standalone method. The
comparison was based on the number of operations
that each method uses to perform the similarity
search query. Since different operations take
different execution times, we used the concept of
latency time (Schulte et al. 2005). We report in
Tables 1 and in Figure 2 the results of (wafer). We
chose to present the results of this dataset because it
is the largest dataset in the repository. Also it is
shown in (Muhammad Fuad and Marteau 2008) that
the best results obtained with SAX were with this
dataset.
ICSOFT 2010 - 5th International Conference on Software and Data Technologies
308