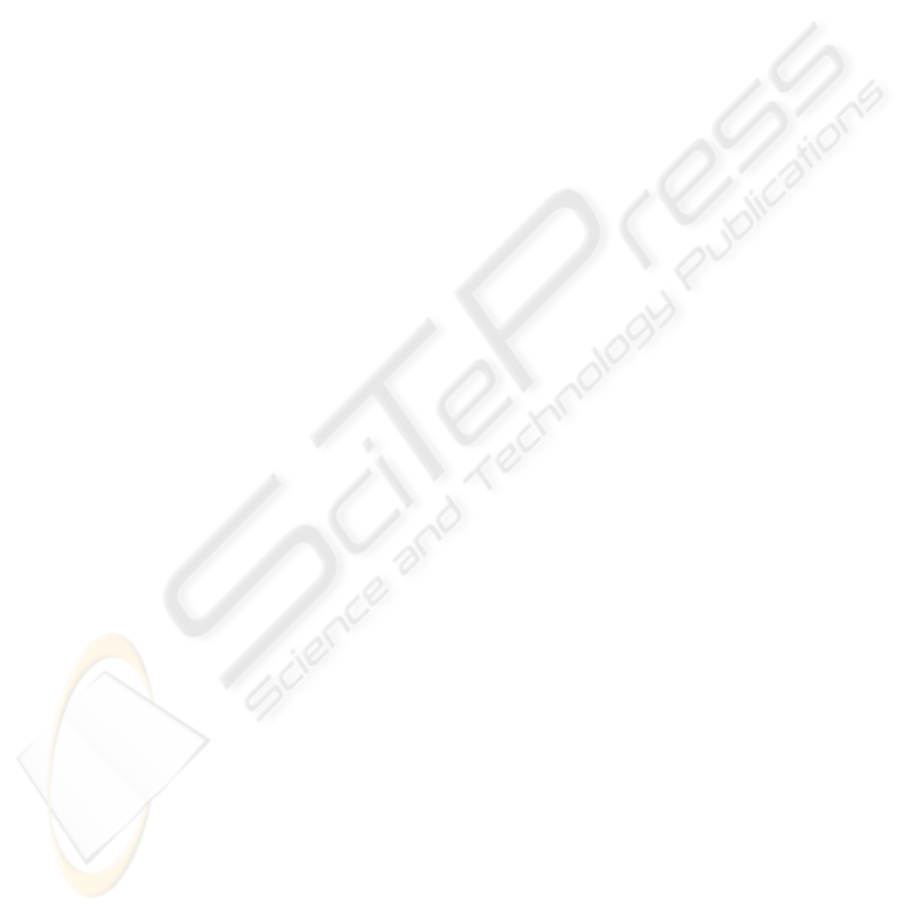
REFERENCES
Beyer, K. and Ramakrishnan, R. Bottom-up computation
of sparse and Iceberg CUBEs. SIGMOD, 28(2):359–
371, 1999.
Chen, Y., Dehne, F., Eavis, T. and Rau-Chaplin, A.
Parallel ROLAP data cube construction on shared-
nothing multiprocessors. Distributed and Parallel
Databases, 15:219-236, 2004.
Chen, Y., Dehne, F., Eavis, T. and Rau-Chaplin, A. PnP:
sequential, external memory, and parallel iceberg
cube computation. Distributed and Parallel Databases,
23(2):99-126, 2008.
Dehne, F., Eavis, T., and Rau-Chaplin, A. Parallelizing
the data cube. Distributed and Parallel Databases,
11(2):181-201, 2002.
Dehne, F., Eavis, T., Hambrush, S. and Rau-Chaplin, A.
Parallelizing the data cube. International Conference
on Database Theory, 2001.
DeWitt, D. and Gray, J. Parallel database systems: the
future of high performance database systems.
Communications of the ACM, 35(6):85-98, 1992.
Dongarra, J., Foster, I., Fox, G., Gropp, W., Kennedy, K.,
Torczon, L. and White, A. Source Book of Parallel
Computing. Morgan Kaufman, 2003.
Goil, S. and Choudhary, A. High performance OLAP and
data mining on parallel computers. Journal of Data
Mining and Knowledge Discovery, (4), 1997.
Goil, S. and Choudhary, A. High performance
multidimensional analysis of large datasets. First
ACM International Workshop on Data Warehousing
and OLAP, pages 34-39, 1998.
Goil, S. and Choudhary, A. A parallel scalable
infrastructure for OLAP and data mining.
International Database Engineering and Application
Symposium, pages 178-186, 1999.
Gray, J., Chaudhuri, S., Bosworth, A., Layman, A.,
Reichart, D., Venkatrao, M., Pellow, F. and Pirahesh,
H. Data cube: A relational aggregation operator
generalizing group-by, cross-tab, and sub totals. Data
Mining and Knowledge Discover, 1(1):29–53, 1997.
Han, J., Pei, J., Dong, G. and Wang, K. Efficient
computation of iceberg cubes with complex measures.
SIGMOD, pages 1–12. ACM, 2001.
Han, J., Kamber, M. Data Mining Concepts and
Techniques. Morgan Kaufman, 2006.
Lakshmanan, L.V.S., Pei, J. and Han, J. Quotient cube:
How to summarize the semantics of a data cube.
VLDB’02, pages 778–789. Morgan Kaufmann, 2002.
Lima, J.C. and Hirata, C.M. MDAG-Cubing: A Reduced
Star-Cubing Approach. SBBD, 362-376, October
2007.
Lu, H., Yu, J., Feng, L. and Li, X. Fully dynamic
partitioning: Handling data skew in parallel data cube
computation. Distributed and Parallel Databases,
13:181-202, 2003.
Li, X., Han, J. and Gonzalez, H. High-dimensional OLAP:
A minimal cubing approach. In VLDB’04, pages 528–
539. Morgan Kaufmann, 2004.
Muto, S. and Kitsuregawa, M. A dynamic load balancing
strategy for parallel data cube computation. ACM 2
nd
Annual Workshop on Data Warehousing and OLAP,
pages 67-72, 1999.
Olken, F., and Rotem, D. Random sampling from database
files - a survey. In 5th International Conference on
Statistical and Scientific Database Management, 1990.
Xin, D., Han, J., Li, X. and Wah, B.W. Star-Cubing:
Computing Iceberg Cubes by Top-Down and Bottom-
Up Integration. VLDB’03, pages 476-487. Morgan
Kaufmann, 2003.
Xin, D., Han, J., Li, X., Shao, Z. and Wah, B.W.
Computing Iceberg Cubes by Top-Down and Bottom-
Up Integration: The StarCubing Approach. IEEE
Transactions on Knowledge and Data Engineering,
19(1): 111-126, 2007.
Xin, D., Shao, Z., Han, J., and Liu, H. C-cubing: Efficient
computation of closed cubes by aggregation-based
checking. ICDE’06, page 4. IEEE Computer Society,
2006.
Zhao, Y., Deshpande, P., and Naughton, J. F. An array-
based algorithm for simultaneous multidimensional
aggregates. SIGMOD, pages 159–170. ACM, 1997.
p-MDAG - A Parallel MDAG Approach
331