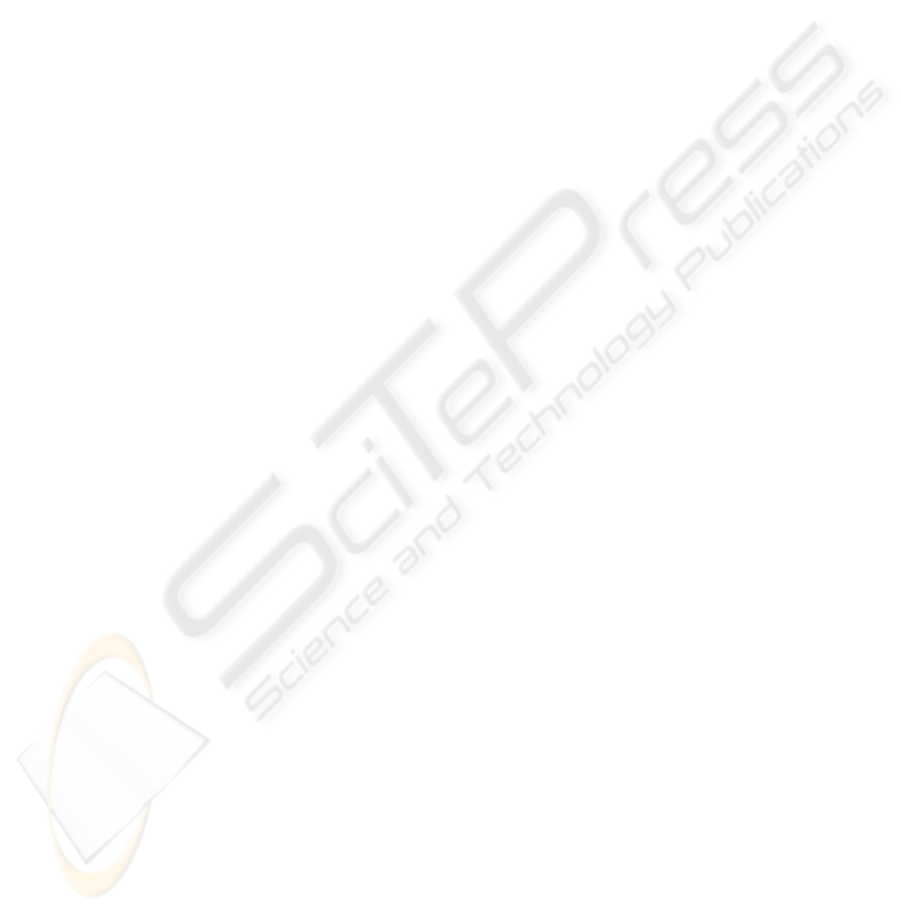
the sample are similar to those of the entire
population within the segment.
This assumption was proven to be right by the fact
that the historical sales trends produced using our
data and methodology matched closely the trends
available from other publicly available sources.
3.6 Validation
We validate our results by various means. First of
all, we compared our long term trends against the
publicly available consumer spending statistics
(Statistics Canada, retail associations, professional
publications, etc.) to calibrate and re-calibrate our
methodology and the business logic implemented in
our SQL scripts. Secondly, after each analysis we
conduct thorough testing of the execution of our
scripts and we analyse and test in detail a few
merchants to ensure the growth rates are reasonable.
Furthermore, we assess the reasonability of the
overall findings based on our professional
experience and industry knowledge. Any trends or
numbers that seem to be significantly different from
our expectations are subject to further analysis to
determine if we have sufficient reasons to trust the
results to be reflecting the market forces, and are not
caused by anomalies in the data or programming
issues.
Parts of our validation process were automated,
however, most of it requires human intervention and
attention, and is performed by highly skilled and
experienced analyst.
3.7 Dissemination of Results
The results are delivered using various media and
formats to different audiences. Our numbers were
packaged as summaries in merchant facing web-
based reports, subscription based printed and web-
based detailed reports for merchants, Excel reports
for internal users, data summaries transferred into
data marts used for internal analysis, and last but not
least, are used for media briefings for printed press,
news agencies and interviews with commercial TV
channels.
4 COMPARATIVE EVALUATION
In this paragraph we will to provide a high-level
comparison of the costs involved in producing retail
sales statistics via the traditional methods using
survey data vs. the Moneris model using POS data.
As Moneris Solutions Corp. is a privately held
company we do have strict confidentiality policies in
place that present limitations regarding the cost data
that we are allowed to disclose in this study. Also,
we do not have access to the cost structure and data
of the organisations and companies that produce
similar retail sales statistics using survey-data.
Nevertheless, as we will show in the coming
paragraphs, the difference in cost structure is so
obvious and order of magnitude so significant that it
leaves no doubt about the cost advantage of the
Moneris POS model. Estimates can be easily
performed based on country specific salary ranges to
quantify the cost advantage in monetary terms.
From Moneris’ point of view - or for a matter of fact
any other similar card processing organisation’s
point of view - the extraction and storage of
transaction level POS data is a must for a variety of
business reasons, such as fraud detection, business
performance reporting, cost monitoring, audit,
charge back processing, etc.
Actually Moneris started collecting this data long
before we started using it for retail sales statistics.
Therefore, the costs associated to the extraction and
storage of the POS data itself are not occurred as a
result of the existence of the retail sales statistics.
The costs directly associated with the production and
validation of the retail sales statistics in a POS
model similar to the one presented in this paper can
be estimated as follows: ~50% time of one database
administrator FTE (full time employee), ~50% time
of one data analyst FTE and ~50% time of one
business analyst FTE, with corresponding
management overhead. Based on our experience
these are conservative estimates.
An initial 3-4 month one-time development effort
for 2-3 FTEs is required to build out the analytical
infrastructure (aggregate dataset structure design,
databases, SQL scripts, Excel templates, etc.) in
addition to the hardware and software costs. This
can take more or less depending on the peculiarities
of the POS data structure and the technologies used.
In comparison, the survey-based methodology in a
market of the size of the Canadian retail market
involves collecting and validating data from
questionnaires sent out to approximately 10,000
retail establishments.
The success of the data collection is highly
dependent on the reduction of non-responses. Data
editing at collection is extremely important.
Replacement values must be calculated for missing
ICEIS 2010 - 12th International Conference on Enterprise Information Systems
450