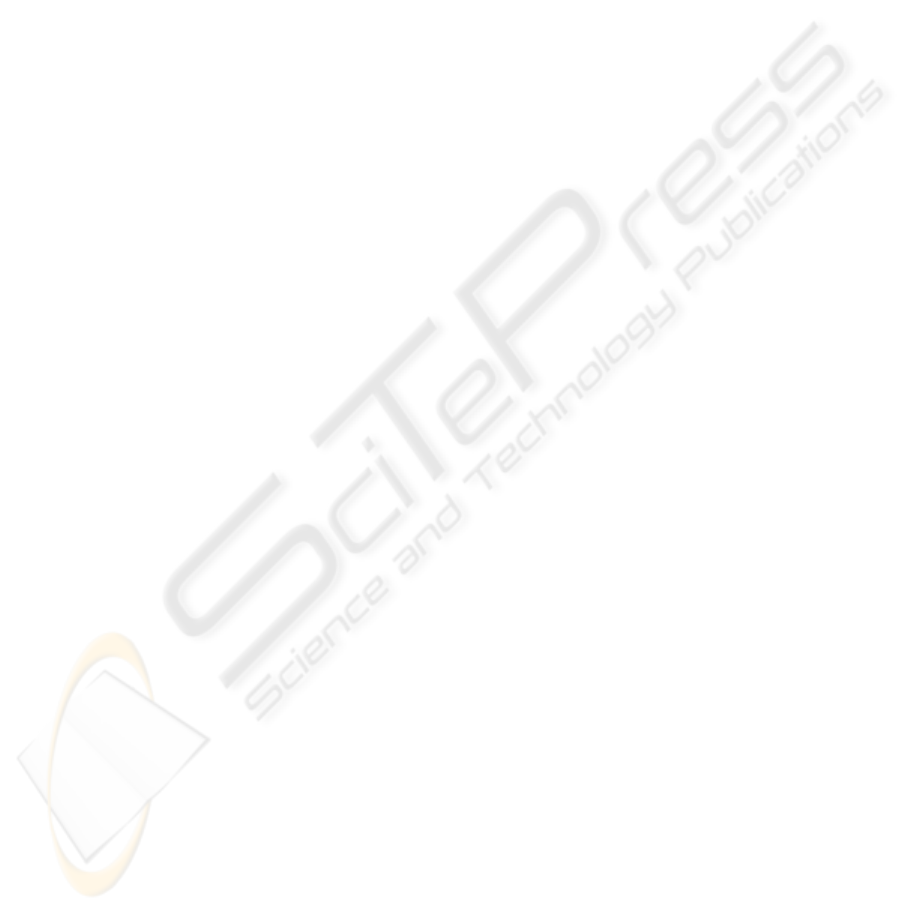
one hand pervasiveness of the underlying technology,
e.g. UMTS and WLAN networks of the radio sig-
nal approach, and on the other hand the obtainable
accuracy for the derived user context. Todays mo-
bile environments are characterized by highly avail-
able, pervasive mobile communication networks, mo-
bile calendar based job itineraries, and mobile devices
with high computational power. Under these precon-
ditions it is recommendable to combine it with radio
based approaches in UMTS/GSM networks for the
detection of the user’s current geographical region.
A rough context of the mobile user can be deduced
therefrom in combination with the event and activ-
ity list from his calendar. This rough context can be
refined with the help of the radio based approach in
WLAN infrastructures that the user will enter, cross,
or leave. To determine the context of a mobile user
in an automated way it is necessery to process knowl-
edge from several sources and services. Thus, there
is need for common ontologies for the description of
the context concept, knowledge description tags char-
acterizing a defined context, and even the events pro-
vided by the signal layer. The next implementation
steps for the proposed context detection architecture
are the definition of a suitable context ontology and
the interaction design of the discussed components of
a Context Detections Service.
REFERENCES
Berchtold, M. and Beigl, M. (2009). Increased robustness
in context detection and reasoning using uncertainty
measures - concept and application. In Proceedings
of the European Conference on Ambient Intelligence
(AmI’09), Salzburg, Austria. Springer.
Brdiczka, O., Crowley, J. L., and Reignier, P. (2007). Learn-
ing situation models for providing context-aware ser-
vices. In HCI (6), pages 23–32.
da Silva, B. C., Basso, E. W., Perotto, F. S., C. Bazzan,
A. L., and Engel, P. M. (2006). Improving reinforce-
ment learning with context detection. In AAMAS ’06:
Proceedings of the fifth international joint conference
on Autonomous agents and multiagent systems, pages
810–812, New York, NY, USA. ACM.
Dey, A. K. (2001). Understanding and using context. Per-
sonal Ubiquitous Comput., 5(1):4–7.
Henricksen, K. and Indulska, J. (2005). Develop-
ing context-aware pervasive computing applications:
Models and approach. Pervasive and Mobile Comput-
ing, In, 2:2005.
Jan, R.-H. and Lee, Y. R. (6-9 Oct. 2003). An Indoor Ge-
olocation System for Wireless LANs. Parallel Pro-
cessing Workshops, 2003. Proceedings. 2003 Interna-
tional Conference on, pages 29–34.
Karantonis, D. M., Narayanan, M. R., Mathie, M., Lovell,
N. H., and Celler, B. G. (2006). Implementation of a
real-time human movement classifier using a triaxial
accelerometer for ambulatory monitoring. Informa-
tion Technology in Biomedicine, IEEE Transactions
on, 10(1):156–167.
Kern, N., Schiele, B., and Schmidt, A. (2003). Multi-sensor
activity context detection for wearable computing. In
In Proc. EUSAI, LNCS, pages 220–232.
Kos, T., Grgic, M., and Sisul, G. (June 2006). Mobile
User Positioning in GSM/UMTS Cellular Networks.
Multimedia Signal Processing and Communications,
48th International Symposium ELMAR-2006 focused
on, pages 185–188.
Krempels, K.-H. and Krebs, M. (2008). Directory-less
WLAN Indoor Positioning. In Proceedings of the
IEEE International Symposium on Consumer Elec-
tronics 2008, Vilamoura Portugal.
Kunczier, H. and Anegg, H. (5-8 Jan. 2004). Enhanced Cell
ID Based Terminal Location for Urban Area Loca-
tion Based Applications. Consumer Communications
and Networking Conference, 2004. CCNC 2004. First
IEEE, pages 595–599.
Luley, P. M., Paletta, L., and Almer, A. (2005). Visual ob-
ject detection from mobile phone imagery for context
awareness. In MobileHCI ’05: Proceedings of the 7th
international conference on Human computer interac-
tion with mobile devices & services, pages 385–386,
New York, NY, USA. ACM.
Ma, L., Smith, D., and Milner, B. (2003). Environmen-
tal noise classification for context-aware applications.
In Mar
´
ık, V., Retschitzegger, W., and Step
´
ankov
´
a, O.,
editors, DEXA, volume 2736 of Lecture Notes in Com-
puter Science, pages 360–370. Springer.
Ranganathan, A., Al-Muhtadi, J., and Campbell, R. H.
(2004). Reasoning about uncertain contexts in perva-
sive computing environments. IEEE Pervasive Com-
puting, 3(2):62–70.
Sama, M., Rosenblum, D. S., Wang, Z., and Elbaum, S.
(2008). Model-based fault detection in context-aware
adaptive applications. In SIGSOFT ’08/FSE-16: Pro-
ceedings of the 16th ACM SIGSOFT International
Symposium on Foundations of software engineering,
pages 261–271, New York, NY, USA. ACM.
Strang, T. and Linnhoff-Popien, C. (2004). A context mod-
eling survey. In In: Workshop on Advanced Context
Modelling, Reasoning and Management, UbiComp
2004 - The Sixth International Conference on Ubiq-
uitous Computing, Nottingham/England.
WINSYS 2010 - International Conference on Wireless Information Networks and Systems
194