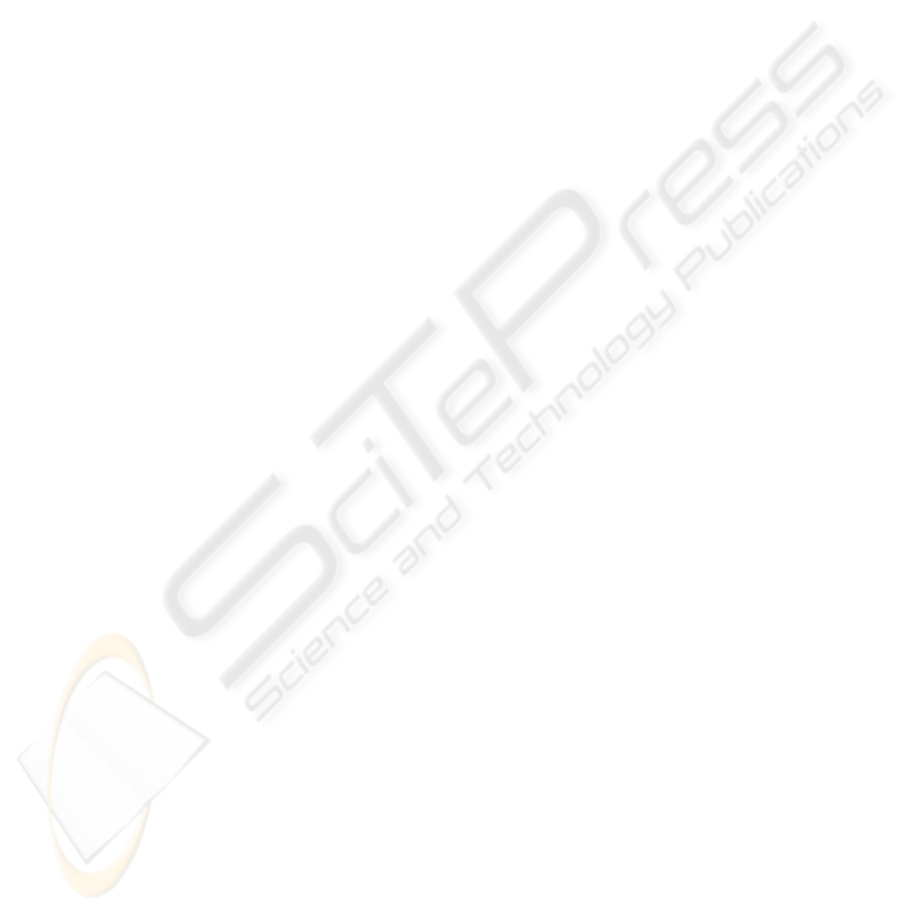
5 EVALUATION
AND CONCLUSIONS
First tests were performed with epidemiologists and
physicians at the CR, who are experienced with mul-
tidimensional concepts. Given the graphical DSL and
new tools the people were able to model and create
new data integration scenarios as well as to import
single and bulk data. The acceptance and comprehen-
sibility of the graphical DSL is a result of the close co-
operation between our institute and CR with its users.
Using the DSL, unemployment figures could be
integrated into the data warehouse to analyze correla-
tions between unemployment and cancer incidence.
Unemployment figures made available by a statis-
tics office, were imported as single data records of
47 rural districts. After discovering a correlation at
this level the cube model of the unemployment fig-
ures was more detailed by the unemployment figures
of boroughs. Eventually, the unemployment figures
combined with gender data of about 1000 boroughs
were integrated for further analysis as bulk data start-
ing in 2003 by using the web service interface.
There are more evaluation scenarios that require
ad hoc data integration but in addition also need new
dimensions to be defined. One important task of
the CR is to answer requests by rural health author-
ities and citizens’ groups. That analysis handles with
small-scale clusters of cancer. That analysis also re-
quired ad hoc integration of special data. For example
population figures on basis of boroughs provided by
the statistics office are integrated yearly. However,
the requests by boroughs require more detailed fig-
ures, e.g. based on street sections. On the one hand
those data requires research of the residents registra-
tion office and on the other hand the spatial dimension
needs to be extended by, for example, street sections.
In addition to modeling new data cubes the creation
or extension of existing dimensions by suitable DSLs
is another important challenge and field of research.
REFERENCES
Batzler, W. U., Giersiepen, K., Hentschel, S., Husmann,
G., Kaatsch, P., Katalinic, A., Kieschke, J., Kray-
winkel, K., Meyer, M., Stabenow, R., and Stegmaier,
C. (2008). Cancer in Germany 2003-2004 Incidence
and Trends. Robert Koch-Institut, Berlin.
Bulos, D. (1996). Olap database design: A new dimension.
Database Programming&Design, Vol. 9(6).
Cook, S., Jones, G., and Kent, S. (2007). Domain Specific
Development with Visual Studio DSL Tools (Microsoft
.net Development). Addison-Wesley Longman, Ams-
terdam.
Dombrowski, E. and Lechtenb
¨
orger, J. (2005). Evalua-
tion objektorientierter Ans
¨
atze zur Data-Warehouse-
Modellierung. Datenbank-Spektrum, 5(15):18–25.
Fowler, M. (2002). Patterns of Enterprise Application Ar-
chitecture. Addison-Wesley Longman.
Gluchowski, P., Kunze, C., and Schneider, C. (2009). A
modeling tool for multidimensional data using the
adapt notation. In 42nd Hawaii International Con-
ference on System Sciences (HICSS-42).
Golfarelli, M., Maio, D., and Rizzi, S. (1998). The dimen-
sional fact model: A conceptual model for data ware-
houses. International Journal of Cooperative Infor-
mation Systems, 7:215–247.
Hahne, M. (2005). Das common warehouse metamodel
als referenzmodell f
¨
ur metadaten im data warehouse
und dessen erweiterung im sap business information
warehouse. In Vossen, G., Leymann, F., Lockemann,
P. C., and Stucky, W., editors, BTW, volume 65 of LNI,
pages 578–595. GI.
Hartmann, S. (2008). berwindung semantischer Hetero-
genitt bei multiplen Data-Warehouse-Systemen. PhD
thesis, University of Bamberg.
Herden, O. (2000). A design methodology for data ware-
houses. In Proc. of the 7th IEEE Intl. Baltic Workshop
(Baltic DB&IS 2000), pages 292–293. IEEE.
Kelly, S. and Tolvanen, J.-P. (2008). Domain-Specific Mod-
eling: Enabling Full Code Generation. John Wiley &
Sons.
Kimball, R., Reeves, L., Ross, M., and Thornthwaite, W.
(1998). The Data Warehouse Lifecycle Toolkit: Expert
Methods for Designing, Developing, and Deploying
Data Warehouses. John Wiley & Sons.
Koch, S., Meister, J., and Rohde, M. (2003). Mustang – a
framework for statistical analyses of multidimensional
data in public health. In Gnauck, A. and Heinrich, R.,
editors, 17th International Conference Informatics for
Environment Protection, pages 635–642.
Mazon, J.-N., Trujillo, J., Serrano, M., and Piattini, M.
(2005). Applying mda to the development of data
warehouses. In DOLAP ’05: Proceedings of the 8th
ACM international workshop on Data warehousing
and OLAP, pages 57–66, New York, NY, USA. ACM.
OMG (2001). Common warehouse metamodel (cwm) spec-
ification. Internet.
Sapia, C., Blaschka, M., H
¨
ofling, G., and Dinter, B. (1999).
Extending the e/r model for the multidimensional
paradigm. In ER ’98: Proceedings of the Workshops
on Data Warehousing and Data Mining, pages 105–
116, London, UK. Springer-Verlag.
V
¨
olter, M. and Stahl, T. (2006). Model-Driven Software
Development. Wiley & Sons.
Wietek, F. (1999). Modelling Multidimensional Data in
a Dataflow-Based Visual Data Analysis Environment,
volume 1626 of Lecture Notes in Computer Science.
Springer.
MODEL-DRIVEN AD HOC DATA INTEGRATION IN THE CONTEXT OF A POPULATION-BASED CANCER
REGISTRY
343