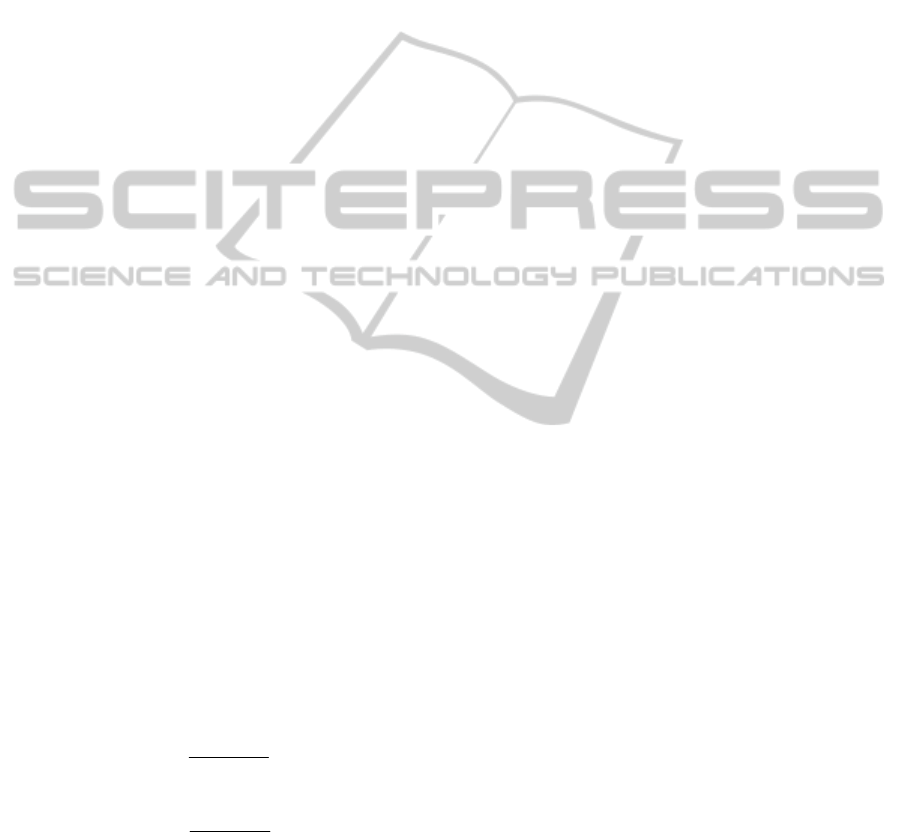
Intuitively, a general and important relation in a
domain would appear frequently in the documents of
this domain, and this corresponds to a frequent sub-
structure of the graphs representing the documents.
Therefore, relation extraction for domain ontology
construction can be converted into discovering
frequent subgraphs within graphs describing the
domain-related corpus. Semantics should be
considered during the mining procedure since
relations discovered should have practical meanings,
rather than just combinations of terms. Thus, relation
extraction in this research is formulated as: given a
dataset of labelled-graph representations (each graph
representation corresponds to a document), a
taxonomy of concepts (concepts are outcomes of the
concept extraction step), a mapping from the labels
to the concepts, and a minimum support threshold,
find all the frequent informative subgraphs and
interpret them as relations.
The gSpan algorithm is used to discover the
frequent subgraphs (Yan and Han, 2002). An
information function defined in Equation (3) to
estimate the information contained in the subgraphs
is integrated into the gSpan algorithm to determine
the importance of the subgraphs.
r
is the frequency
that the subgraph g appears in the graph database.
The factor
()
g of the information related to the
subgraph g is the sum of the information carried by
the vertex
()vVg of weight
()
v
wv
and the edge
()eEg of weight ()
e
we:
:( , ) ( )
ir r
sg Ig
(3)
() ()
() () ()
ve
vV g eEg
givie
(4)
Information associated with a vertex or edge
weight are given in Equations (5) and (6)
respectively.
{| ,()()}
vv
dDvdlv lv
and
{| ,()()}
ee
d D e dle le
are the subsets of graph
database D respectively.
2
()
() log
()
v
v
dD
v
vd
wv
iv
wv
(5)
2
()
() log
()
e
e
dD
e
ed
we
ie
we
(6)
Besides the information function, three
constraints are considered in relation extraction,
namely, (1) the concepts obtained from the prior step
are used to determine whether the current vertex
should be discovered as an element of one relation
when mining the frequent subgraphs; (2) for relation
extraction, the part-of-speech attribute of a term is
considered, i.e., each subgraph discovered must
contain at least one noun and one verb or adjective;
and (3) if the subgraphs mined contain vertices that
are not in the list of concepts, these vertices can be
added as concepts with respect to their term weights,
which can be regarded as the feedback from the
relations to the concepts.
After the frequent subgraph mining, the
subgraphs obtained will be interpreted as relations
between the concepts. A relation is described as a
triple {concept1, relation, concept2}. A general way
is to first locate the node labelled with a verb, and
find the adjacent nodes. If both adjacent nodes are
labelled with a noun, a relation between these three
terms is established. If one or both nodes are
labelled with a verb, these nodes are connected to
form a new node labelled with the union of the verb
labels. The new node will be used as the middle
word for the next iteration. This process is iterated
until two nodes labelled with a noun are connected
by a node labelled with a verb. Next, the two nouns
and the verb are interpreted as concept1 and
concept2 and the relation, respectively. This process
is applicable to a few nouns that are adjacent. A few
typical situations encountered in subgraph
interpretation are illustrated in Figure 2. It should be
noted that more than one relation may be extracted
from one subgraph; the number of relations
extracted from one subgraph depends on the number
of verb concepts in this subgraph as the core of a
relation is a verb term. Figure 3 shows an example
of frequent subgraph mining and the interpretation
of a subgraph as relations.
3 CONSTRUCTION OF FIXTURE
DESIGN ONTOLOGY
This section presents the construction of fixture
design ontology using the proposed approach. A
snippet of the ontology on “fixture design” is shown
in Figure 4. The new ontology created is compared
with a fixture design ontology FIXON to
demonstrate the validity of the proposed method.
Since the sources from which the two ontologies are
constructed are different, only the common parts of
the two ontologies are compared. From Table 1, the
performance of the proposed method as compared
with FIXON is as follows: the concept precision is
70.8%, the concept-location precision is 71.4%, and
the concept recall is 76.8%.
AUTOMATIC ONTOLOGY CONSTRUCTION FOR MANUFACTURING KNOWLEDGE AND INFORMATION
MANAGEMENT
333