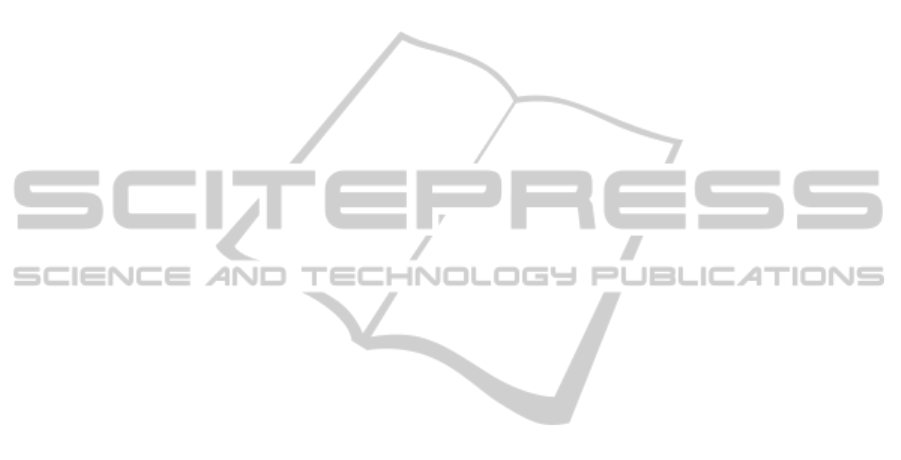
automated annotation. Secondly, the merging of the
manual annotation and its later validation is useful
for obtaining a set of well-annotated documents for
further evaluation of automatic annotations.
The findings of this work can be used in other
domains of knowledge where unstructured data has
to be annotated using a domain-specific ontology. In
this context, it has to be considered, where the
needed knowledge is stored. If using only instances
for the annotation, the ontology could become huge.
For example, if every function owner should be part
of the ontology, huge classifications or standards
have to be integrated. For instance, transferring the
products and services categorization standards
eCl@ss in OWL yielded 75,000 ontology classes
plus more than 5,000 properties (Hepp, 2006).
Alternatively, you may use a combination of
ontological knowledge and linguistic patterns (or
rules) for annotation. For example, modelling only
on the (technical) operations in the ontology and
defining patterns to annotate a technical function in
combination with an identified noun in the sentence
would decrease the size of the ontology, as the
number of technical operations is limited. However,
the number of rules to be defined will increase.
What works best has to be judged considering the
relevant domain and the complexity of the modelled
knowledge.
6 CONCLUSIONS AND
OUTLOOK
The analysis of solution documents done in this
research permits an insight into the content of
solution documents in the field of automation
technology. With the help of the proposed ranking
numbers, important instances can be identified
according to the manual annotations made by
different persons. This ranking numbers can be
subsequently used for the evaluation of an
automated annotation. The evaluation of the used
prototype showed need for improvement concerning
the annotation of related instances in the ontology.
To improve this annotation, further work will
focus on the interpretation of the made analyses for
identifying patterns in the syntax or layout of
solution documents. Furthermore, the personal
background of the manual annotations will be
considered for the purpose of identify individual
requirements on the annotation. This will improve
the automatic annotation and may also be
instrumental to identifying the “core functions” of a
technical solution.
ACKNOWLEDGEMENTS
This work has been funded by the German Federal
Ministry of Economy and Technology (BMWi)
through THESEUS. The authors wish to
acknowledge gratitude and appreciation to all the
PROCESSUS project partners for their contribution
during the development of various ideas and
concepts presented in this paper.
REFERENCES
Blumberg, R., and Atre, S. (2003). The Problem with
Unstructured Data. In DM Review, 13(2).
Corcho, O. (2006). Ontology based document annotation:
trends and open research problems. Int. J. of Metadata,
Semantics and Ontologies, 1(1), 47–57.
Dylla, N. (1990). Denk- und Handlungsabläufe beim
Konstruieren, PhD thesis, Technische Universität
München.
Gaag, A., Kohn, A., and Lindemann, U., (2009). Function-
based Solution Retrieval and Semantic Search in
Mechanical Engineering. In ICED’09, 17th
International Conference on Engineering Design,
Stanford, California, USA.
Hepp, M. (2003). Güterklassifikation als semantisches
Standardisierungsproblem. Wiesbaden: Deutscher
Universitäts-Verlag.
Hepp, M. (2006). Products and Services Ontologies: A
Methodology for Deriving OWL Ontologies from
Industrial Categorization Standards. Int. J. on
Semantic Web & Information Systems, 2 (1), 72-99.
Ponn, J., and Lindemann, U. (2008). Konzeptentwicklung
und Gestaltung technischer Produkte. Berlin:
Springer.
Pocsai, Z. (2000). Ontologiebasiertes Wissensmanagement
für die Produktentwicklung. PhD thesis, Technische
Universität Karlsruhe.
Ponn, J., Deubzer, F., and Lindemann, U., (2006).
Intelligent Search for Product Development
Information - an Ontology-based Approach. In:
DESIGN’06, 9th International Design Conference,
Dubrovnik, Croatia.
Salton, G. and McGill, M. J. (1986). Introduction to
Modern Information Retrieval. New York: McGraw-
Hill.
Uren, V., Cimiano, P., Iria, J. e., Handschuh, S., Vargas-
Vera, M., Motta, E., and Ciravegna, F. (2006).
Semantic annotation for knowledge management:
Requirements and a survey of the state of the art.
Journal of Web Semantics, 4(1), 14-28.
Vallet, D., Fernández, M., and Castells, P., (2005). An
Ontology-Based Information Retrieval Model. In
ESWC’05, The Semantic Web: Research and
Applications, Second European Semantic Web
Conference. Heraklion, Crete, Greece.
KEOD 2010 - International Conference on Knowledge Engineering and Ontology Development
158