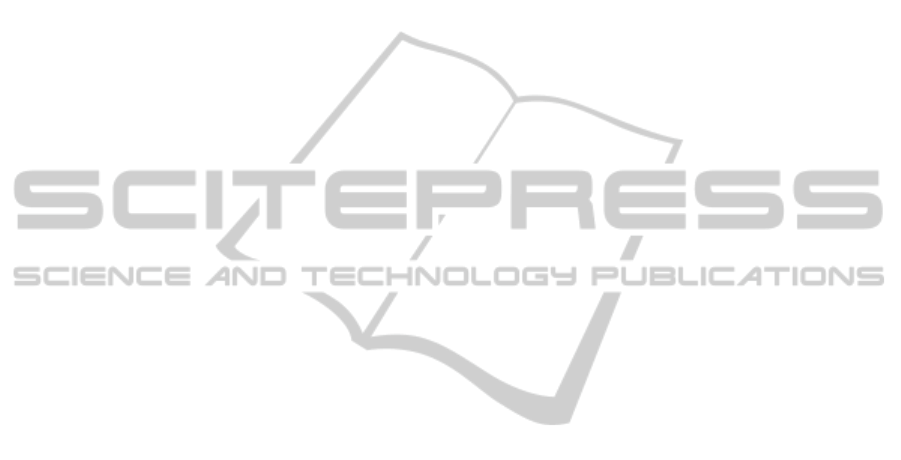
between neglect patients and non neglect CVA
patients lies at the very beginning of the motion
where the classification between them is quite high
(89%). Such pointers may aid researchers in
hypothesizing models of brain functions and in
designing the experiments to validate them.
The zero-class approach suggested, especially in
the 3D study, how the rigid distinction between
various conditions may be misleading. It was shown
how sometimes neglect patients behave in a similar
way to non-neglect CVA patients or how certain
CVA patients belong in the same cluster. This
approach can point the rehabilitation professionals to
better understanding and organization of
heterogeneous or wide spectrum disorders.
4.2 Future Directions
One immediate goal is to apply one-class algorithms
on these data. One-class filters are those that are
trained and produced using only data from one-class,
yet it produces a classification on new data that says
the data point is in the class or not.
Building one class filters may assist the field of
rehabilitation science in one of its severe
shortcomings, which is the lack of large samples of
clinical populations. One can only imagine the
benefits of building one-class filters from different
VR platforms and applications. We propose that
upon finding relevant data segments we can
accumulate data from different settings. After
sufficient training of a set of one-class filters, one
can bring a novel data vector and test it on these
filters to see whether this patient tested positive for
the "mild neglect" or "severe neglect" or "mild
upper-left hemi-field but otherwise OK" etc. This
may focus the clinicians in treating only the
impaired faculties of the patients.
Preliminary results indicate that in our 3D data a
one-class filter achieved 97% success rate in
labeling "severe neglect". Thus this may be a
promising direction.
Another direction would be to test our approach
in current frontiers of neurological rehabilitation.
One example would be finding a way to easily
diagnose between spatial neglect and hemianopsia,
which is related to different brain mechanisms, but
leads to a behavior similar to that of neglect patients.
Similarly, it would be of significant value if
zero-class clustering may aid in separating the wide
spectrum of attention deficit disorders (ADD) into
meaningful sub-categories.
Finally, in our vision for the long range, we see
the possibility of "closing the loop" and using the
classification and clustering methodology as keys
for making rehabilitation protocols both adaptive
and individualized. This is especially tempting in the
context of rehabilitation in the virtual reality
environment. What is needed is the development of
a virtual model of the individual which we would
want to extract automatically from data based on his
performance in the VR sessions. Then an individual
rehabilitative protocol can be obtained by simulating
the behavior of an avatar in the VR; and simply
testing how the avatar improves under a large
variety of protocols. Once a good one is established,
it can be immediately applied to the patient who is
being treated in the same VR environment.
ACKNOWLEDGEMENTS
Thanks to Assaf Dvorkin, Jim Patton, Eugene
Mednikov, Debbie Rand, Rachel Kizony, Neta Erez,
Meir Shahar, Patrice L. Weiss and the Caesarea
Rothschild Institute. Authors are listed
alphabetically. This work appears as part of the
M.Sc. thesis of Natan Silnitsky.
REFERENCES
Deouell LY, Sacher Y, Soroker N. Assessment of spatial
attention after brain damage with a dynamic reaction
time test. J Intern Neuropsychol Society 2005; 11: 697-
707.
Dvorkin AY, Rymer WZ, Harvey RL, Bogey RA, Patton JL
(2008) Assessment and monitoring of recovery of
spatial neglect within a virtual environment. In: IEEE
Virtual Rehabilitation. p. 88-92, Vancouver, Canada.
Katz, N., Ring H., Naveh, Y., Kizony, R., Feintuch, U. and
Weiss, P.L. (2005). Interactive virtual environment
training for safe street crossing of right hemisphere
stroke patients with Unilateral Spatial Neglect.
Disability and Rehabilitation, 29(2), 177-181.
Patton, J. L., Dawe, G., Scharver, C., Mussa-Ivaldi, F.A.,
and Kenyon, R. (2006) Robotics and virtual reality: A
perfect marriage for motor control research and
rehabilitation. Assistive Technology 18: 181-195.
Rand, D., Katz, N., Shahar, M., Kizony, R., and Weiss, P.
L.: The virtual mall: development of a functional virtual
environment for stroke rehabilitation. Abstracts of the
55th Annual Conference of the Israeli Association of
Physical and Rehabilitation Medicine. Tel Aviv 2004.
Robertson, I. H. and Halligan, P. W. Spatial neglect: a
clinical handbook for diagnosis and treatment. Hove,
UK: Psychology Press, 1999.
Weiss, P.L., Kizony, R., Feintuch, U., & Katz, N. Virtual
reality in neurorehabilitation. In M.E. Selzer, S. Clarke,
L.G. Cohen, P. Duncan, & F. Gage (Eds.). Textbook of
Neural Repair and Rehabilitation - Medical
Rehabilitation. pp 182-197. Cambridge:
Cambridge University Press. Cambridge. 2006.
ICFC 2010 - International Conference on Fuzzy Computation
252