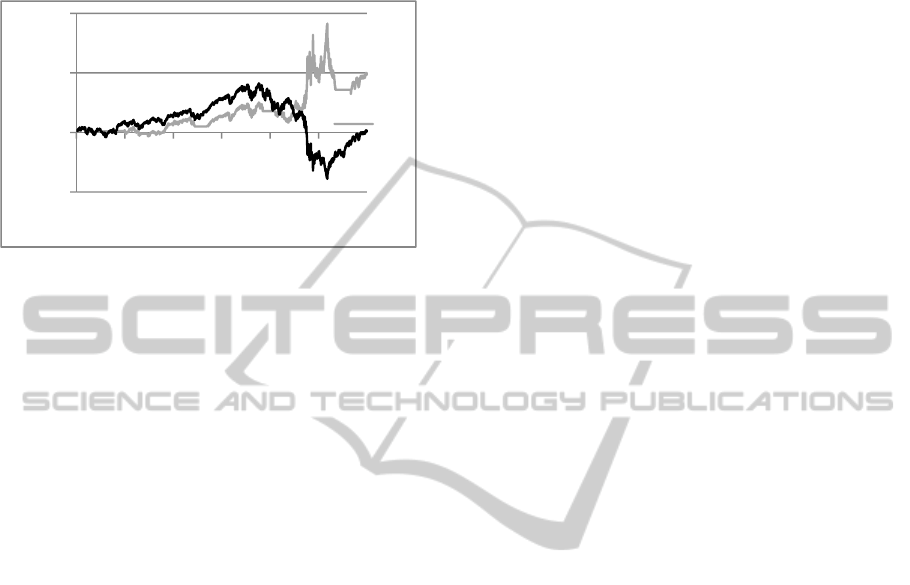
is the investor profile risk. The investor can choose
between a strategy with better returns but more
volatility (the “SMAC & MAD”) and the SMAC
with more regular but less attractive results.
Figure 3: Evolution of the return of the Buy and Hold, and
the “MAD (108, 20) & SMAC(206, 195)” strategy, on
S&P500 from 2004 to 2009.
In Figure 3 we can see the evolution of the return of
the strategy with the best results in the training
period, during the test period, compared with the
evolution of the Buy and Hold.
The proposed strategy is best suited for medium and
long term investment since it only takes a decision
after the confirmation of a trend is clear, it has the
great advantage of avoiding long periods of
downtrends. The classical stategy of Buy and Hold
that is only good in markets that do not exibited bear
markets like the 80s and 90s in the S&P500 does not
perform well in markets characterized by long bear
markets.
5 CONCLUSIONS
This document presented the use of Genetic
Algorithms to optimize the parameters of various
Technical Indicators and with them create various
trading strategies. The results obtain showed that
this strategies beat significantly the Buy and Hold
(the “MAD & SMAC” strategy had an average of
9.0% against the 2.6% of the Buy and Hold), once
more proving the validity of Technical Analysis.
Finally the optimized “MAD & SMAC” strategy is
compared with the random strategy, with excellent
results: the optimized has an average of return of
9.0% against the -1.01% of the random strategy. The
use of the “MAD & SMAC” has also shown better
results than the use of any of the indicators
individually.
REFERENCES
Bodas-Sagi, et al. (2009). Multiobjective optimization of
technical market indicators. In Proceedings of the
GECCO '09, Montreal, Canada .
Brock, et al. (1992). Simple Technical Trading Rules and
the Stochastic Properties of Stock Returns. Journal of
Finance, American Finance Association, vol. 47(5) ,
1731-6.
Canegrati, E. (2008). A Non-Random Walk down Canary
Wharf. MPRA Paper, University Library of Munich .
Chan, E. P. (2009). Quantitative Trading. New Jersey:
John Wiley and Sons.
Ellis, Craig A. & Parbery, Simon, A. (2005). Is smarter
better? A comparison of adaptive, and simple moving
average trading strategies. Research in International
Business and Finance, Elsevier, vol. 19(3) , 399-411.
Fama, Eugene F. (1998). Market efficiency, long-
term returns, and behavioral finance. Journal of
Financial Economics, Elsevier, vol. 49(3) , 283-306.
Fernández-Blanco, P., et al. (2008). Technical market
indicators optimization using evolutionary algorithms.
Proceedings of the 2008 GECCO conference
companion on Genetic and evolutionary computation ,
1851-1857.
Gorgulho, A., Neves, R. and Horta N. (2009). Using GAs
to Balance Technical Indicators on StockPicking for
Financial Portfolio Composition. Proceedings of the
GECCO, 2009, Montreal, Canada. , 2041-2046.
Jegadeesh, N., and Titman, S. (1993). Returns to buying
winners and selling losers: Implica-tions for stock
market efficiency. Journal of Finance 48 , 65-91.
Kaufman, P. J. (2005). New Trading Systems And
Methods. San Francisco, CA: John Wiley & Sons Inc.
Kimoto, T. and Asakawa, K. (1990). Stock Market
Prediction System with Modular Neural Networks.
IJCNN International Joint Conference on Neural
Networks, vol. 1 , 1-6.
Kirkpatrick, C. D. and Dahlquist R. D. (2009). Technical
Analysis. FT press.
Sharpe, W. F,. (1994). The Sharpe Ratio. Journal of
Portfolio Management 21 (1) , 49–58.
Yan, W. and Clack, C. (2007). Evolving Robust GP
Solutions for Hedge Fund Stock Selection in
Emerging Markets. Proceedings of the GECCO 2007 ,
London, UK, 2234-224.
‐50%
0%
50%
100%
2004 2005 2006 2007 2008 2009
MA
ICEC 2010 - International Conference on Evolutionary Computation
310