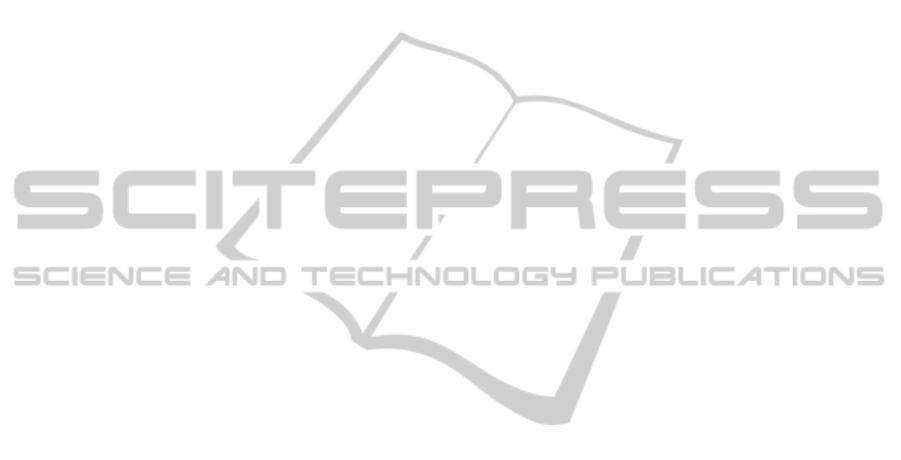
for US companies and municipalities. The assessed
objects were labelled by rating classes from rating
agencies. The selection of input variables was
realized as a two-step procedure. First, the original
sets of input variables were proposed based on
previous studies. Then correlation based approach
together with GA was employed with the aim of
reducing the original sets. The PNNs showed best
results for both the corporate and the municipal
credit rating problem. The results conform to prior
research results (Brennan and Brabazon, 2004;
Huang et al., 2004) indicating that the models of
NNs based on publicly available financial and
nonfinancial information could provide accurate
classifications of credit ratings. The sets of variables
identified in this study captured the most relevant
information for the credit rating decision.
In future research, the sets of input variables can
be extended in order to involve also the qualitative
factors of credit rating process. So far, these
variables have been either ignored or replaced with
alternative quantitative input variables.
REFERENCES
Brabazon, A. and O’Neill, M. (2006). Credit Classification
using Grammatical Evolution. Informatica, 30, 325-
335.
Brennan, D. and Brabazon, A. (2004). Corporate Bond
Rating using Neural Networks, In Arabnia, H.R. (Ed.),
Proceedings of the Conference on Artificial
Intelligence (pp. 161-167).
Delahunty, A. and O’Callaghan, D. (2004). Artificial
Immune Systems for the Prediction of Corporate
Failure and Classification of Corporate Bond Ratings.
Dublin: University College Dublin.
Farnham, P. G. and Cluff, G. S. (1982). Municipal Bond
Ratings: New Directions, New Results. Public
Finance Quarterly, 26, 427-455.
Gaganis, Ch., Pasiouras, F. and Doumpos, M. (2007).
Probabilistic Neural Networks for the Identification of
Qualified Audit Opinions. Expert Systems with
Applications, 32, 114-124.
Gaillard, N. (2009). The Determinants of Moody’s Sub-
Sovereign Ratings. International Research Journal of
Finance and Economics, 31, 194-209.
Hajek, P. and Olej, V. (2008). Municipal Creditworthiness
Modelling by Kohonen’s Self-Organizing Feature
Maps and Fuzzy Logic Neural Networks. In Kurkova,
V. (Ed.), Lecture Notes in Artificial Intelligence (pp.
533-542).
Hajek, P. and Olej, V. (2009). Municipal Creditworthiness
Modelling by Kernel Based Approaches with
Supervised and Semi-Supervised Learning. In Palmer-
Brown, D., Draganova, Ch., Pimenidis, E. and
Mouratidis, H. (Eds.), Communications in Computer
and Information Science (pp. 35-44).
Hall, M. A. (1998). Correlation-Based Feature Subset
Selection for Machine Learning. Hamilton: University
of Waikato.
Huang, Z., Chen, H., Hsu, Ch. J., Chen, W. H. and Wu, S.
(2004). Credit Rating Analysis with Support Vector
Machines and Neural Networks: A Market
Comparative Study. Decision Support Systems, 37,
543-558.
Hwang, R. Ch. and Cheng, K. F. (2008). On Multiple-
Class Prediction of Issuer Credit Ratings. Applied
Stochastic Models in Business and Industry, 5, 535-
550.
Lee, Y. Ch. (2007). Application of Support Vector
Machines to Corporate Credit Rating Prediction.
Expert Systems with Applications, 33, 67-74.
Li, S. T., Shue, L. Y. and Shiue, W. (2000). The
Development of a Decision Model for Liquidity
Analysis. Expert Systems with Applications, 19, 271-
278.
Loviscek, L. A. and Crowley, F. D. (2003). Municipal
Bond Ratings and Municipal Debt Management. New
York: Marcel Dekker.
Maher, J. J. and Sen, T. K. (1997). Predicting Bond
Ratings using Neural Networks: A Comparison with
Logistic Regression. Intelligent Systems in
Accounting, Finance and Management, 6, 59-72.
Moody, J. and Utans, J. (1995). Architecture Selection
Strategies for Neural Networks Application to
Corporate Bond Rating. In Refenes, A.N. (Ed.),
Neural Networks in the Capital Markets (pp. 277-
300).
Olej, V. and Hajek
, P. (2007). Hierarchical Structure of
Fuzzy Inference Systems Design for Municipal
Creditworthiness Modelling. WSEAS Transactions on
Systems and Control, 2, 162-169.
Serve, S. (2001). Assessment of Local Financial Risk: The
Determinants of the Rating of European Local
Authorities. Lugano: EFMA.
Singleton, J. C. and Surkan, A. J. (1995). Bond Rating
with Neural Networks. In Refenes, A.N. (Ed.), Neural
Networks in the Capital Markets (pp. 301-307).
Specht, D. F. (1990). Probabilistic Neural Networks.
Neural Networks, 3, 109-118.
Wasserman, P. D. (1993). Advanced Methods in Neural
Computing. John Wiley & Sons: VNR Press.
ICFC 2010 - International Conference on Fuzzy Computation
294