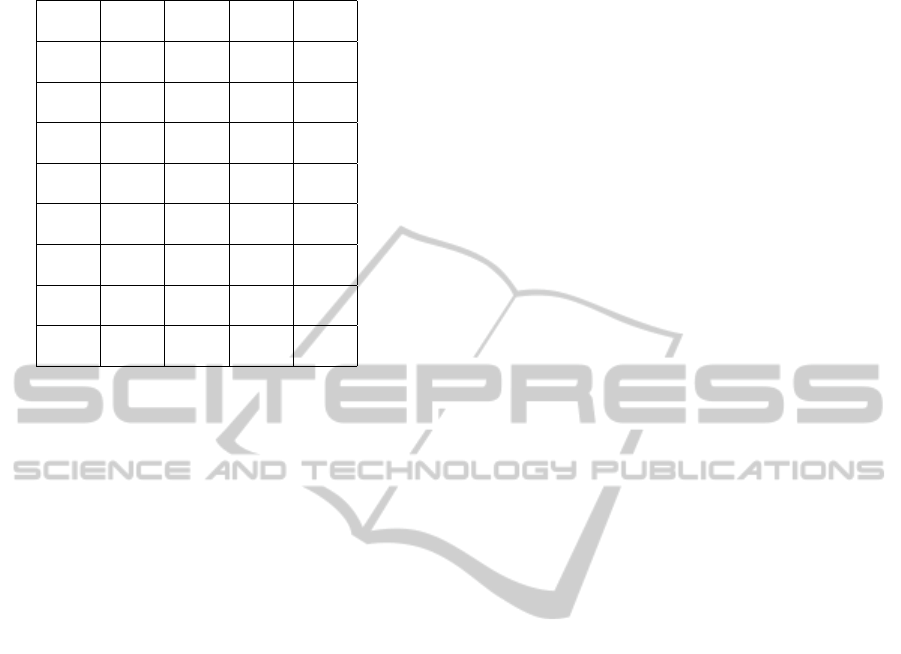
Table 2: Relative frequency of non-null data.
id 01 id 02 id 03 id 04 id 05
0,115 0,036 0,024 0,308 0,179
id 06 id 07 id 08 id 09 id 10
0,164 0,052 0,129 0,057 0,176
id 11 id 12 id 13 id 14 id 15
0,030 0,128 0,021 0,061 0,013
id 16 id 17 id 18 id 19 id 20
0,083 0,004 0,093 0,049 0,014
id 21 id 22 id 23 id 24 id 25
0,017 0,145 0,015 0,012 0,018
id 26 id 27 id 28 id 29 id 30
0,015 0,024 0,006 0,066 0,097
id 31 id 32 id 33 id 34 id 35
0,166 0,174 0,046 0,003 0,004
id 36 id 37 id 38 id 39 id 40
0,002 0,006 0,001 0,091 0,048
id 41 id 42 id 43 id 44 id 45
0,116 0,583 0,049 0,011 0,006
quent substitution using satellite data can be success-
fully achieved, as proved by the experience in the
classification of vegetation types using the LandSat
data.
In addition, at least in the study zone, there is
no noticeable interaction between various vegetation
covers to complicate the training of the networks
which, in other situations, would have to be studied.
This situation may be due in part to the values ob-
tained: for each quadrat of land, the values indicate a
dominant vegetation type, so that 90% of the samples
have a vegetation cover of more than 41.9, while 50%
of cases have an vegetation cover exceeding 68.8. In
such a situation, the information obtained from the
satellite data is representing, in the majority of cases,
the dominant characteristic and for this reason, there
are no undesired interactions. In addition, the matrix
contains a large number of nil or very low data values:
92.3% of data are zero and only 55,800 data of a total
of 985,725 in the matrix, have values above 5.
5 CONCLUSIONS
As a final point, the approximation functions of the
ecological variables developed here using radial basis
function networks could be used in subsequent years
to study changes in vegetation cover. Although the
vegetation cover changes seasonally, it is also true that
the experiment could be repeated for different seasons
of the year, so long as this cover existed.
The use of Landsat data in this case reduces field
studies in at least 30%. Neural networks can recog-
nize geographical locations with similar vegetation
characteristics at any given time. This situation will
allow work teams to to study the Landsat information
previously available and improve the work on a sur-
face, saving costs.
From a technical point of view, the study also co-
rroborates the need for a precise study of the training
data set in order to achieve a precise training so
that the results are consistent with the environmen-
tal model simulated. The results confirm our working
hypothesis that supports the viability of a computation
process of ecological variables that uses satellite data
that could substitute for the traditional field studies.
ACKNOWLEDGEMENTS
This work has been supported by the EU (FEDER)
and the Spanish MICINN under grant of TIN2007-
61497 and TIN2010-1558 projects.
REFERENCES
Loveland, T. R. and Merchant, J. M. (2004). Ecoregions and
ecoregionalization: geographical and ecological pers-
pectives. In Environmental Management 34. Springer
New York.
Moreira, J. (2000). Reconocimiento biof
´
ısico de espa-
cios naturales protegidos. Parque natural Sierras
Subb
´
eticas. Junta de Andaluc
´
ıa, Sevilla, 1st edition.
Naiman, R., Loranrich, D., Beechie, T., and Ralph, S.
(1992). General principles of classification and the
assessment of conservation potential in rivers. In
River Conservation and Management. John Wiley and
Sons.
Pablo, C. D. (2000). Cartograf
´
ıa ecol
´
ogica: conceptos y
procedimientos para la representaci
´
on espacial de eco-
sistemas. In Bolet
´
ın de la Real Sociedad Espaola de
Historia Natural. Real Sociedad Espaola de Historia
Natural.
Poggio, T. and Girosi, F. (1990). A theory of networks for
approximation and learning. In Proceedings of the
IEEE 78. Massachusetts Institute of Technology.
Revenga, C. (2005). Developing indicators of ecosystem
condition using geographic information systems and
remote sensing. In Regional Environmental Change
5. Springer Berlin and Heidelberg.
Richards, J. (1993). Remote sensing digital image analysis.
An introduction. Springer-Verlag, Berlin, 2nd edition.
Snelder, T., Leathwick, J., and Dey, K. (2007). A proce-
dure for making optimal selection of input variables
for multivariate environmental classifications. In Con-
servation Biology 21. National Institute of Water and
Atmospheric Research.
Snelder, T., Lehmann, A., Lamouroux, N., Leathwick, J.,
and Allenbach, K. (2010). Effect of classification
procedure on the performance of numerically defined
ecological regions. In Environmental Management 45.
Springer New York.
ICFC 2010 - International Conference on Fuzzy Computation
300