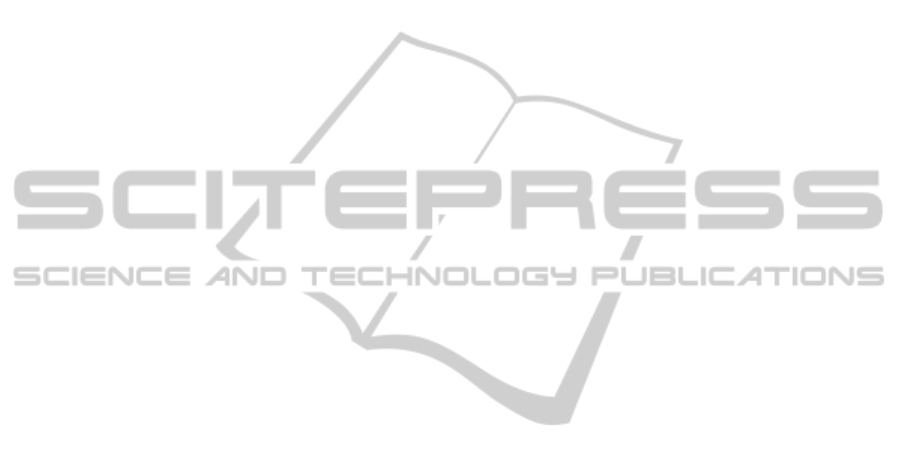
interest models is to identify a VIG, which is an
ordered set of other system users who exhibit
interests similar to those of the target user. As a
result, we can evaluate our user modeling algorithm
through a VIG identification task. In a formative
NIST evaluation using intelligence analysts, we
achieved 95% VIG identification precision and
recall.
In the evaluation, we have explored a couple of
other information object modelers especially those
based on topic distributions such as Latent Dirichlet
Allocation (LDA) (Wang et al. 2007). The results
were not conclusive due to insufficient time and
resource for tuning. In the future we would like to
study them further. It would also be interesting to
acquire the relevance assignments for different types
of ALEs automatically by restricting inputs to VIG
algorithm to single ALE types and compare the
impacts on VIG identification performance. Lastly,
we would like to apply clustering algorithms to the
task identification problem using the generated
segment models.
ACKNOWLEDGEMENTS
This study was supported and monitored by the
Intelligence Advanced Research Projects Activity
(IARPA) under the CASE MASTER project with
contract number FA8750-06-C-0193. The views,
opinions, and findings contained in this report are
those of the authors and should not be construed as
an official IARPA position, policy, or decision,
unless so designated by other official
documentation.
We would like to thank the NIST team headed
by Emile Morse for conducting the formative
evaluation of our work. We are also indebted to New
Vectors and Oculus teams for their collaboration and
software support. We also owe our gratitude to
FairIsaac and BAE teams for providing the object
modeler support and software.
REFERENCES
Alonso, R., Bloom, J.A., Li, H. and Basu, C., 2003. An
adaptive nearest neighbor search for a parts acquisition
ePortal Proceedings of the ninth ACM SIGKDD
international conference on Knowledge discovery and
data mining, ACM, Washington, D.C.
Alonso, R. and Li, H., 2005a. Combating Cognitive Biases
in Information Retrieval. in First International
Conference on Intelligence Analysis Methods and
Tools, McLean, VA, USA.
Alonso, R. and Li, H., 2005b. Model-guided information
discovery for intelligence analysis Proceedings of the
14th ACM international conference on Information
and knowledge management, ACM, Bremen,
Germany.
Hijikata, Y., 2004. Implicit user profiling for on demand
relevance feedback Proceedings of the 9th
international conference on Intelligent user interfaces,
ACM, Funchal, Madeira, Portugal.
Guy, I., Ronen, I. and Wilcox, E., 2009. Do you know?:
recommending people to invite into your social
network Proceedings of the 13th international
conference on Intelligent user interfaces, ACM,
Sanibel Island, Florida, USA.
Kelly, D. and Teevan, J., 2003. Implicit feedback for
inferring user preference: a bibliography. SIGIR
Forum, 37 (2). 18-28.
Li, Y., Algarni, A., Wu, S.-T. and Xue, Y., 2009. Mining
Negative Relevance Feedback for Information
Filtering Proceedings of the 2009 IEEE/WIC/ACM
International Joint Conference on Web Intelligence
and Intelligent Agent Technology - Volume 01, IEEE
Computer Society.
McDonald, D.W., 2003. Recommending collaboration
with social networks: a comparative evaluation
Proceedings of the SIGCHI conference on Human
factors in computing systems, ACM, Ft. Lauderdale,
Florida, USA.
Mostafa, J., Mukhopadhyay, S., Palakal, M. and Lam, W.,
1997. A multilevel approach to intelligent
information filtering: model, system, and evaluation.
ACM Trans. Inf. Syst., 15 (4). 368-399.
Oard, D.W. and Kim, J., 2001. Modeling information
content using observable behavior. in Proceedings of
the 64th Annual Meeting of the American Society for
Information Science and Technology, 38-45.
Pazzani, M.J. and Billsus, D., 1997. Learning and
Revising User Profiles: The Identification of
Interesting Web Sites. Machine Learning, 27. 313-
331.
Schroh, D., Bozowsky, N., Savigny, M. and Wright, W.,
2009. nCompass Service Oriented Architecture for
Tacit Collaboration Services Proceedings of the 2009
13th International Conference Information
Visualisation - Volume 00, IEEE Computer Society.
Shen, X., Tan, B. and Zhai, C., 2005. Implicit user
modeling for personalized search Proceedings of the
14th ACM international conference on Information
and knowledge management, ACM, Bremen,
Germany.
Teevan, J., Dumais, S.T. and Horvitz, E., 2005.
Personalizing search via automated analysis of
interests and activities Proceedings of the 28th
annual international ACM SIGIR conference on
Research and development in information retrieval,
ACM, Salvador, Brazil.
KMIS 2010 - International Conference on Knowledge Management and Information Sharing
134