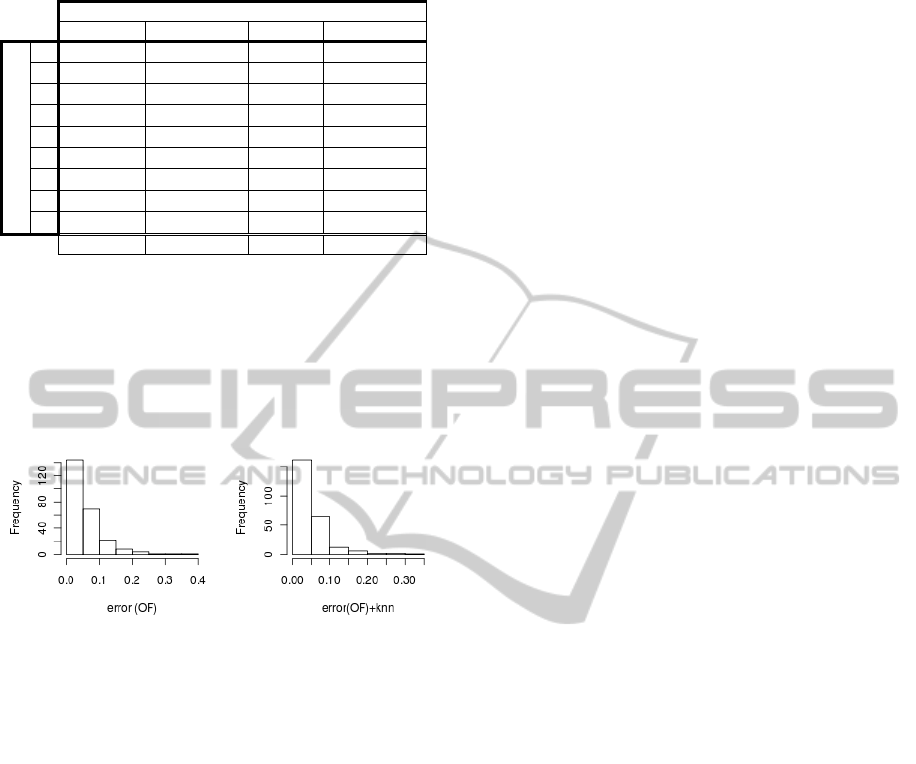
Table 2: Distribution of selected ontology for each user.
Clustering method
agglom. agglom-knn part. part-knn
Criteria function
1 13 16 12 0
2 6 8 4 4
3 4 9 3 2
4 9 3 0 11
5 11 14 9 5
6 3 8 2 2
7 22 12 NA NA
8 8 25 NA NA
9 10 15 NA NA
Total
86 110 30 24
In relative terms this means an improvement of
14.1%.
To determine if this difference between the
average errors of both methods is significant we
have used the Wilcoxon test for paired samples,
since there is no normal distribution of errors, as can
be seen in Figure 3.
Figure 3: Wilcoxon test.
The p-value Wilcoxon test is less than 10
-6
which
indicates that the difference between the magnitudes
of the errors is significant and cannot be attributed to
chance.
5 CONCLUSIONS
In this work we have presented the recommendation
engine developed for the customization and
advertisement managers within an audiovisual
content delivery platform. The main novelty of our
work is the reusable approach of the
recommendation engine for different contexts. In
this sense, we can add new different filtering
techniques and different content types easily. In this
work we have used the ontology filtering technique.
This work has enhanced the traditional ontology
filtering technique by means of an imputation of
ratings in the user-item matrix.
6 FUTURE WORK
The extended use of social networks introduces an
important element to extend user profile as the user
interacts with other users in the network. Moreover,
some geolocalization aspects must be considered for
recommending advertisements.
At the moment we are updating the ontology
filtering technique in order to improve the quality of
the recommendations even more.
REFERENCES
Herlocker, J., Konstan, J.A., Borchers, A, Riedl, J., 1999.
An Algorithmic FrameWork for Performing
Collaborative Filtering. In 22nd Annual International
ACM SIGIR Conference on Research and
Development in Information Retrieval, Berkeley, CA,
pp. 230-237.
Sarwar, B., Karypis, G., Konstan, J., Riedl, J. 2001. Item-
based collaborative filtering recommendation
algorithms. In 10th Conference on World Wide Web,
Hong Kong, pp. 285-295.
Vozalis, E., Margaritis, K.G. 2003. Analysis of
Recommender Systems’ Algorithms. In 6
th
Hellenic
European Conference on Computer Mathematics and
its Applications (HERCMA), Athens, Greece.
O´Connor, M., Herlocker, J. 1999. Clustering Items for
Collaborative Filtering. In Proceedings of the ACM
SIGIR Workshop on Recommender Systems:
Algorithms and Evaluation, Berkeley, CA.
Pazzani, M.J., Billsus, D. 2007. Content-Based
Recommendation Systems. Lectures Notes in
Computer Science, vol. 4321, Springer, pp. 325-341.
Schickel-Zuber, V. 2007. Ontology Filtering. Thesis,
Lausanne, EPFL.
Troyanskaya, O., Cantor, M., Sherlock, G., Brown, P.,
Hastie, T., Tibshirani, R., Botstein, D., Altman, R.B.
2001. Missing value estimation methods for DNA
microarrays. Bioinformatics, vol. 17, n. 6, pp. 520-
525.
Dataset Movielens. http://www.cs.umn.edu/
Research/GroupLens/data (visited March 23, 2010).
Herlocker, J.L., Konstan, J.A., Terveen, L.G., Riedl, J.T.
2004. Evaluating Collaborative Filtering
Recommender Systems. In ACM Transactions on
Information Systems, vol. 22, n. 1, pp. 5-53.
KDIR 2010 - International Conference on Knowledge Discovery and Information Retrieval
382