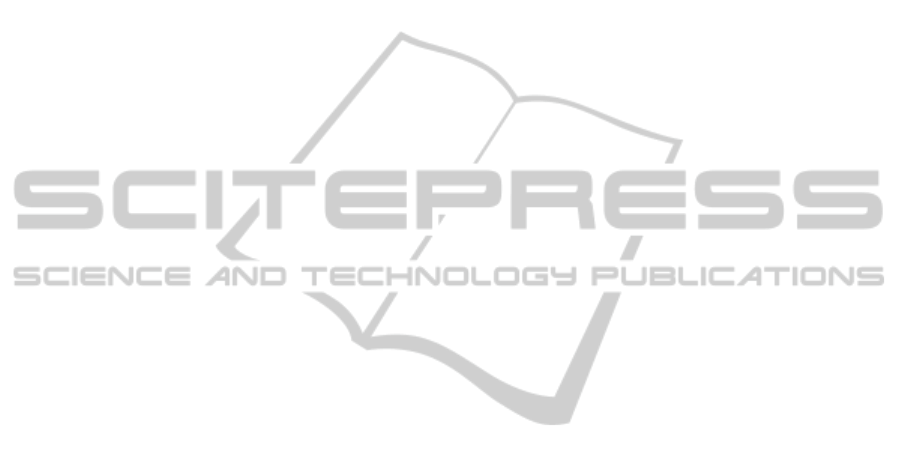
the complete set of features we obtained a satisfactory
result with a value of F
1
= 0.86. The precision value
of P = 0.88 determines the ability to embrace the pro-
posed solution in real-world scenarios. The addition
of anchortext features is able to improve both P and R
passing from an overall F
1
of 0.72 to 0.86. This latter
observation deserves particular attention since justi-
fies the definition of the proposed novel ML model.
Moreover experimental results show that the use
of the f
fsize
and f
fbold
visual features enable a further
improvement of the recognition performance, in par-
ticular the Recall value that goes from 0.81 to 0.84.
5 CONCLUSIONS AND FUTURE
WORKS
In this paper we have shown that ML techniques can
be used in conjunction with LA to achieve automatic
recognition of the main entity from pages with a given
topic and well-known web-usability-driven structure.
Experimental results show encouraging results on the
proposed dataset and highlight the advantage of com-
bining the two sources of information, text blocks
with their visual formatting styles and incoming an-
chor texts. Future works include the experimentation
on other website domains and the extension of the
current set general purpose features with additional
domain specific features.
REFERENCES
Cai, D., Yu, S., Wen, J.-R., and Ma, W.-Y. (2003). Ex-
tracting content structure for web pages based on vi-
sual representation. In Web Technologies and Applica-
tions: 5th Asia-Pacific Web Conference, APWeb 2003,
Xian, China, April 23-25, 2003. Proceedings, page
596.
Carullo, M., Binaghi, E., and Gallo, I. (2009). Soft cate-
gorization and annotation of images with radial basis
function networks. In VISSAPP, International Con-
ference on Computer Vision Theory and Applications,
volume 2, pages 309–314.
Chakrabarti, S., Dom, B., and Indyk, P. (1998). En-
hanced hypertext categorization using hyperlinks. In
SIGMOD ’98: Proceedings of the 1998 ACM SIG-
MOD international conference on Management of
data, pages 307–318, New York, NY, USA. ACM.
Congalton, R. (1991). A review of assessing the accuracy of
classifications of remotely sensed data. Remote sens-
ing of environment, 37(1):35–46.
Frakes, W. B. and Baeza-Yates, R. A., editors (1992). In-
formation Retrieval: Data Structures & Algorithms.
Prentice-Hall.
F¨urnkranz, J. (2002). Web structure mining - exploiting the
graph structure of the world-wide web.
¨
OGAI Journal,
21(2):17–26.
Joachims, T., De, T. J., Cristianini, N., and Uk, N. R. A.
(2001). Composite kernels for hypertext categorisa-
tion. In In Proceedings of the International Confer-
ence on Machine Learning (ICML, pages 250–257.
Morgan Kaufmann Publishers.
Kosala, R. and Blockeel, H. (2000). Web mining research:
a survey. SIGKDD Explor. Newsl., 2(1):1–15.
Michalski, R. S., Carbonell, J. G., and Mitchell, T. M.
(1983). Machine Learning, An Artificial Intelligence
Approach. McGraw-Hill.
Mitchell, T. M. (1997). Machine Learning. McGraw-Hill,
New York.
Moody, J. E. and Darken, C. (1989). Fast learning in net-
works of locally-tuned processing units. Neural Com-
putation, 1:281–294.
Oh, H.-J., Myaeng, S. H., and Lee, M.-H. (2000). A prac-
tical hypertext catergorization method using links and
incrementally available class information. In SIGIR
’00: Proceedings of the 23rd annual international
ACM SIGIR conference on Research and development
in information retrieval, pages 264–271, New York,
NY, USA. ACM.
Rubner, Y., Tomasi, C., and Guibas, L. J. (2000). The earth
mover’s distance as a metric for image retrieval. Int.
J. Comput. Vision, 40(2):99–121.
Spertus, E. (1997). Parasite: mining structural informa-
tion on the web. Comput. Netw. ISDN Syst., 29(8-
13):1205–1215.
Zhang, M.-L. and Zhou, Z.-H. (2006). Adapting rbf neural
networks to multi-instance learning. Neural Process.
Lett., 23(1):1–26.
MACHINE LEARNING AND LINK ANALYSIS FOR WEB CONTENT MINING
161