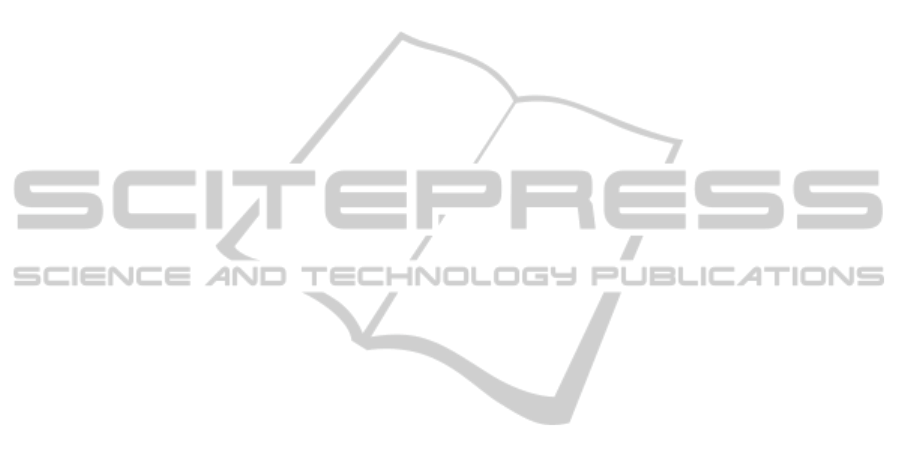
tions once a pattern of utility scores has been recog-
nized. (For other issues related to reducing the com-
plexity of knowledge acquisition of influence dia-
grams see Bielza, Gomez and Shenoy (2010).) Fol-
lowing these tenets, we successfully configured our
first demonstration system of CLAD.
As regards incorporating aspects of influence
diagram creation into OntoElicit, our current think-
ing is that experts could, in fact, be led through the
process of decomposing the problem into the main
variables in the decision vs. the variables in local
decisions (cf. point (1) above), but we have yet to
experiment with this methodology.
4 CONCLUSIONS
In this paper, we have provided a sweeping introduc-
tion to some of the different kinds of modeling
strategies used within the OntoAgent environment.
We have shown how our problem space design has
facilitated the creation of a mixed-initiative KE sys-
tem for encoding clinical knowledge in the metalan-
guage of the OntoAgent environment. One of the
advantages of our modeling strategies is that the
knowledge is formulated such that it can understood
not only by the expert him- or herself but also by the
wider community, as illustrated in Jarrell et al.
(2008). (Cf. the KADS principle that collected data
and analysis should be documented.) Although we
are well aware that such general strategies will not
be sufficient to overcome all modeling challenges,
we believe that they are beneficial in helping experts
to conceptualize domains quickly, independently
and in the most practical way. In this sense we be-
lieve that this work makes a contribution to over-
coming the knowledge bottleneck in constructing
practical knowledge-based systems.
REFERENCES
Beale, S., Lavoie, B., McShane, M., Nirenburg, S.,
Korelsky, T., 2004. Question answering using Onto-
logical Semantics. In Proceedings of ACL-2004 Work-
shop on Text Meaning and Interpretation.
Bielza, C., Gomez, M., Shenoy, P. P., 2010. Modeling
challenges with influence diagrams: Constructing
probability and utility models. In Decision Support
Systems (in press).
Breuker, J. (Ed.), 1987. Model-driven knowledge acquisi-
tion interpretation models. Deliverable task AI, Esprit
Project 1098 (University of Amsterdam).
Cooke, N.J. (no date), Knowledge elicitation. Available at
http://www.cerici.org/documents/Publications/Durso%
20chapter%20on%20KE.pdf
Ford, D. N., Sterman, J. D., 1998. Expert knowledge elici-
tation to improve formal and mental models. In Syst.
Dyn. Rev. 14: 309-340.
Hoffman, R. A., Lintern, G., 2006. Eliciting and
representing the knowledge of experts. In Ericsson, K.
A., Charness, N., Feltovich, P., Hoffman, R. (Eds.),
Cambridge Handbook of Expertise and Expert Per-
formance, pp. 203-222. New York, Cambridge Uni-
versity Press.
Howard, R. A., Matheson, J. E., 2005. Influence diagrams.
In Decision Anal. 2(3): 127–143.
Jarrell, B., Nirenburg, S., McShane, M., Fantry, G.,
Beale, S., 2008. Revealing the conceptual substrate of
biomedical cognitive models to the wider community.
In Medicine Meets Virtual Reality 16.
Lucas, P., 1996. Knowledge acquisition for decision-
theoretic expert systems. In AISB Quaterly, 94: 23-33.
McShane, M., Nirenburg, S., 2003. Parameterizing and
eliciting text elements across languages. In Machine
Translation 18(2): 129-165.
McShane, M., Nirenburg, S., Beale, S., 2005. Semantics-
based resolution of fragments and underspecified
structures. In Traitement Automatique des Langues
46(1): 163-184.
McShane, M., Fantry, G., Beale, S., Nirenburg, S, Jarrell,
B., 2007a. Disease interactions in cognitive simula-
tions for medical training. In Proceedings of MODSIM
World Conference, Medical Track.
McShane, M., Nirenburg, S., Beale, S., Jarrell, B., Fantry,
G., 2007b. Knowledge-based modeling and simulation
of diseases with highly differentiated clinical manife-
stations. In Proceedings of the 11th Conference on Ar-
tificial Intelligence in Medicine.
McShane, M., Nirenburg, S., Beale, S., Catizone, R.,
Submitted. A cognitive architecture for simulating bo-
dies and minds. Submitted to ICAART-2011.
Nirenburg, S., McShane, M., Beale, S., 2008a. A simu-
lated physiological/cognitive “double agent”. In Pro-
ceedings of the Workshop on Naturally Inspired Cog-
nitive Architectures at AAAI 2008 Fall Symposium.
Nirenburg, S., McShane, M., Beale, S., Jarrell, B., 2008b.
Adaptivity in a multi-agent clinical simulation system.
In Proceedings of AKRR'08 - International and Inter-
disciplinary Conference on Adaptive Knowledge Re-
presentation and Reasoning.
Nirenburg, S., Raskin, V., 2004. Ontological semantics.
Cambridge, Mass., The MIT Press.
Schank, R., Abelson, R., 1977. Scripts, plans, goals and
understanding. Hillsdale, NJ, Erlbaum.
Shadbolt, N., Burton, M., 1995. Knowledge elicitation. In
Corlett, E.N., Wilson, J.R., (Eds.), Evaluation of hu-
man work. CRC Press.
KEOD 2010 - International Conference on Knowledge Engineering and Ontology Development
182