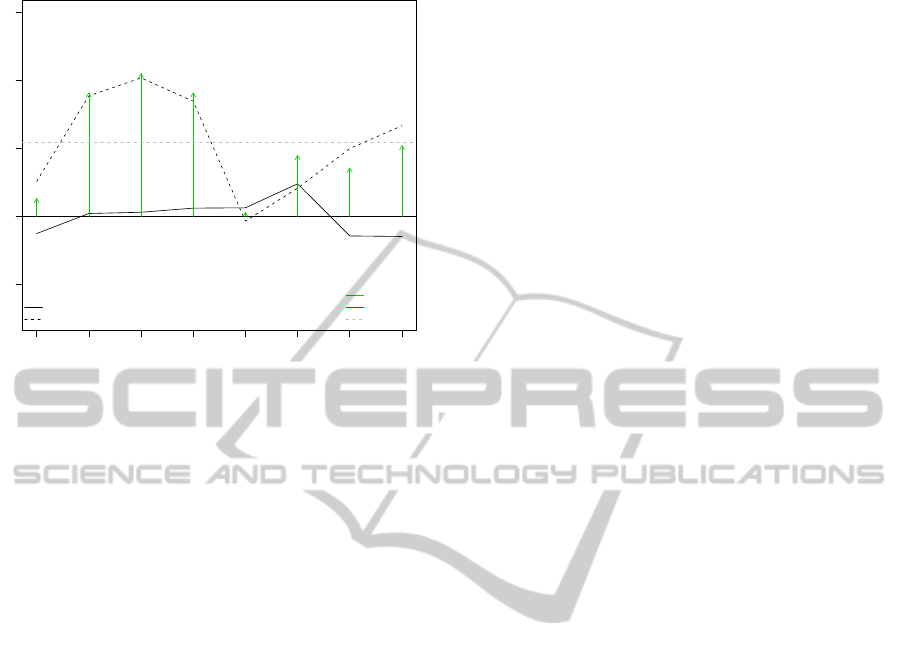
−0.2 0.0 0.2 0.4 0.6
∆(PrecisionOrPMI4kLSA4k, PrecisionAnd)
∆(RecallOrPMI4kLSA4k, RecallAnd)
OR + LSA 4000 + PMI 4000
Hotel pos
Products pos
Camera pos
Election pos
Hotel neg
Products neg
Camera neg
Election neg
Data Sets per Class
●
●
●
●
●
●
●
●
●
●
●
●
●
●
●
●
avg.gain = 0.217
●
●
precision
recall
pos. gain
neg. gain
avg. gain
Figure 3: Evaluation results of the different merging strate-
gies. The arrows indicate a gain or loss in PRG.
5 CONCLUSIONS
The evaluation shows small improvements using only
LSA or a combination of LSA and PMI-IR. Yet, we
believe that their application to smaller dictionaries
would have more effect. Using a combined measure-
ment like the proposed Precision-Recall-Gain helps
highlighting small improvements. As a future work
we see the exploration of different levels of document
granularity. Using sentences or paragraphs as the unit
for indexing could improve the proposed extension
strategies.
ACKNOWLEDGEMENTS
The RAVEN Research Project (Relation Anal-
ysis and Visualization for Evolving Networks;
www.modul.ac.at/nmt/raven) is funded by the Aus-
trian Ministry of Transport, Innovation & Technol-
ogy (BMVIT) and the Austrian Research Promotion
Agency (FFG) within the strategic objective FIT-IT
Semantic Systems (www.fit-it.at). We would like to
thank Albert Weichselbraun for providing the data
sets for the evaluation and Gerhard Wohlgenannt for
the PMI-IR Analysis. We also thank Jode Ziegen-
fuß for proof reading the manuscript.
REFERENCES
Deerwester, S. C., Dumais, S. T., Landauer, T. K., Furnas,
G. W., and Harshman, R. A. (1990). Indexing by latent
semantic analysis. Journal of the American Society of
Information Science, 41(6):391–407.
Gindl, S. and Liegl, J. (2008). Evaluation of different sen-
timent detection methods for polarity classification on
web-based reviews. In Proceedings of the ECAI Work-
shop on Computational Aspects of Affectual and Emo-
tional Interaction.
Landauer, T. K. and Dumais, S. T. (1997). A solution to
plato’s problem: The latent semantic analysis theory
of the acquisition, induction, and representation of
knowledge. Psychological Review, 104:211–240.
Mullen, T. and Collier, N. (2004). Sentiment analysis us-
ing support vector machines with diverse information
sources. In Lin, D. and Wu, D., editors, Proceedings
of EMNLP 2004, pages 412–418, Barcelona, Spain.
Association for Computational Linguistics.
Pang, B., Lee, L., and Vaithyanathan, S. (2002). Thumbs
up? Sentiment classification using machine learning
techniques. In Proceedings of the 2002 Conference on
Empirical Methods in Natural Language Processing
(EMNLP).
Rafelsberger, W. and Scharl, A. (2009). Games with a pur-
pose for social networking platforms. In Proceedings
of the 21st ACM Conference on Hypertext and Hyper-
media.
Read, J. and Carroll, J. (2009). Weakly supervised
techniques for domain-independent sentiment clas-
sification. In Proceedings of the 1st International
CIKM Workshop on Topic-Sentiment Analysis for
Mass Opinion Measurement.
Stone, P. J., Dunphy, D. C., and Smith, M. S. (1966). The
General Inquirer : A Computer Approach to Content
Analysis. MIT. Press, Cambridge, Mass. [u.a.].
Turney, P. (2002). Thumbs up or thumbs down? Seman-
tic orientation applied to unsupervised classification
of reviews. In Proceedings of the 40th Annual Meet-
ing of the Association for Computational Linguistics.
Turney, P. D. (2001). Mining the web for synonyms: PMI–
IR versus LSA on TOEFL. In Proceedings of the 12th
European Conference on Machine Learning.
Wilson, T., Wiebe, J., and Hoffmann, P. (2005). Recogniz-
ing contextual polarity in phrase-level sentiment anal-
ysis. In Proceedings of Human Language Technolo-
gies Conference/Conference on Empirical Methods in
Natural Language Processing (HLT/EMNLP 2005),
Vancouver, CA.
Yu, H. and Hatzivassiloglou, V. (2003). Towards answering
opinion questions: separating facts from opinions and
identifying the polarity of opinion sentences. In Pro-
ceedings of the 2003 conference on Empirical meth-
ods in natural language processing, pages 129–136,
Morristown, NJ, USA. Association for Computational
Linguistics.
DICTIONARY EXTENSION FOR IMPROVING AUTOMATED SENTIMENT DETECTION
407