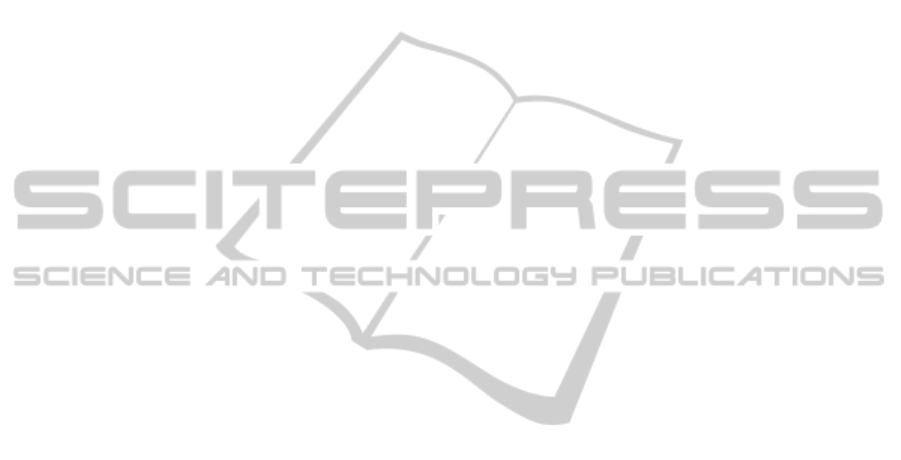
coming from social network analysis. Third, critical
situations during opinion formation are spotted by a
fuzzy perceptron on the basis of the opinions of the
network members, the influence of the opinion lead-
ers as well as the structure of the network. Choosing
a neuro fuzzy approach allows the learning of lin-
guistic rules which can be easily interpreted by mar-
keting managers. These rules are learned from past
situations and can be employed to judge future situa-
tions.
There are a lot of advantages in discovering crit-
ical situations. Being alerted at an early stage, mar-
keting managers can influence the process of opi-
nion formation. For instance, they can address opi-
nion leaders who have a negative opinion and ask
their advice about product improvements. This ac-
tion might not only reveal valuable information for
product development but might also lead to a change
in the leaders’ opinions as they have the impression
that their complaint is being taken seriously. All in
all, this approach attempts to improve a company’s
image and to increase its sales volume.
Scheduled work is to implement a decision sup-
port system that not only identifies critical situations
but also generates recommendations on appropriate
actions for marketing mangers. For example, the
system should advise marketing managers how to
communicate with network members in critical
situations.
REFERENCES
Bodendorf, F., Kaiser, C., (2009). Detecting Opinion
Leaders and Trends in Online Social Networks. In
Proceedings of the 2nd Workshop on Social Web
Search and Mining. Hong Kong.
Borgelt, C., Klawonn, F., Kruse, R., Nauck, D., 2003.
Neuro-Fuzzy-Systeme: Von den Grundlagen
künstlicher Neuronaler Netze zur Kopplung mit Fuzzy
Systemen. [engl.: Neuro-Fuzzy-Systems: Foundations
of the combination of neural networks and fuzzy-
systems.] (3th ed.). Wiesbaden: Vieweg.
Chang, C. L., Chen, D. Y., and Chuang, T. R., (2002).
Browsing Newsgroups with a Social Network Analyz-
er. In Proceedings of the Sixth International Confe-
rence on Information Visualization, London.
Choudhury, M. D., Sundaram, H., John, A., Seligmann, D.
D. (2007): Contextual Prediction of Communication
Flow in Social Networks. In Proceedings of the
IEEE/WIC/ACM international Conference on Web in-
telligence (Silicon Valley, California, USA). Web In-
telligence. IEEE Computer Society, Washington, DC,
pp. 57-65.
Choudhury, M. D. (2009). Modelling and Predicting
Group Activity over Time in Online Social Media. In
Proceedings of the Twentieth ACM Conference on
Hypertext and Hypermedia. Torino, Italy.
Choudhury, M. D., Sundaram, H.,John, A., Seligmann, D.
D. (2009). Which are the Representatative Groups in a
Community? Extracting and Characterizing Key
Groups in Blogs. ACM Student Research Competition,
HyperText '09.
Cortes C., Vapnik V. N., 1995. Support Vector Networks.
In Machine Learning, Vol. 20, pp. 273-297.
Dave, K., Lawrence, S., Pennock, D., M. (2003). Mining
the peanut gallery: Opinion extraction and semantic
classification of product reviews. In Proceedings of
the 12th international conference on World Wide Web.
Dhar, V., Chang, E. (2007). Does Chatter Matter? The
Impact of User-Generated Content on Music Sales.
Technical Report, Leonard N. Stern School of Busi-
ness, New York University.
Glance, N., Hurst, M., Nigam, K., Siegler, M., Stockton,
R., Tomokiyo, T. (2005). Deriving Marketing Intelli-
gence from Online Discussion. In Proceedings of the
eleventh ACM SIGKDD international conference on
Knowledge discovery in data mining. Chicago, Illi-
nois, USA, pp. 419 – 428.
Gomez, V., Kaltenbrunner, A., and Lopez, V. (2008).
Statistical Analysis of the Social Network and Discus-
sion Threads in Slashdot, In Proceedings of the Inter-
national World Wide Web Conference, Beijing: ACM
Press.
Gruhl, D., Guha, R., Kumar, R., Novak, J., Tomkins, A.
(2005). The Predictive Power of Online Chatter. In
Proceedings of the eleventh ACM SIGKDD interna-
tional conference on Knowledge discovery in data
mining. Chicago, Illinois, USA, pp. 78 - 87.
Huang, Y., Liu, S., Wang, Y. (2007). Online Detecting
and Tracking of the Evolution of User Communities.
In Third International Conference on Natural Compu-
tation, pp.681-685.
Kaiser, C. (2009): Combining Text Mining and Data
Mining For Gaining Valuable Knowledge from Online
Reviews. In Pedro Isaías (Ed.). IADIS International
Journal on WWW/Internet 6 (2), pp. 63-78, 2009.
Kaiser, C., Bodendorf, F. (2009). Opinion and Relation-
ship Mining in Online Forums. In Proceedings of the
2009 IEEE/WIC/ACM International Joint Conference
on Web Intelligence and Intelligent Agent Technology.
Milan, pp. 128-131.
Katz E., Lazarsfeld P. F, (1955). Personal influence, the
part played by people in the flow of mass communica-
tion, Glencoe: Free Press.
Keller E. B., Berry J., (2003). The influentials, New York:
Free Press.
Kim, S.-M., Hovy, E. (2007). Crystal: Analysing Predic-
tive Opinions on the Web. In proceedings of the 2007
Joint Conference on the Empirical Methods in Natural
Language Processing and Computational Natural
Language Learning, Prague, pp. 1056-1064.
Nauck, D., Kruse, R., (1994). A Fuzzy Perceptron as a
Generic Model for Neuro-Fuzzy Approaches. In
Fuzzy Systeme '94.
DISCOVERING CRITICAL SITUATIONS IN ONLINE SOCIAL NETWORKS - A Neuro Fuzzy Approach to Alert
Marketing Managers
63