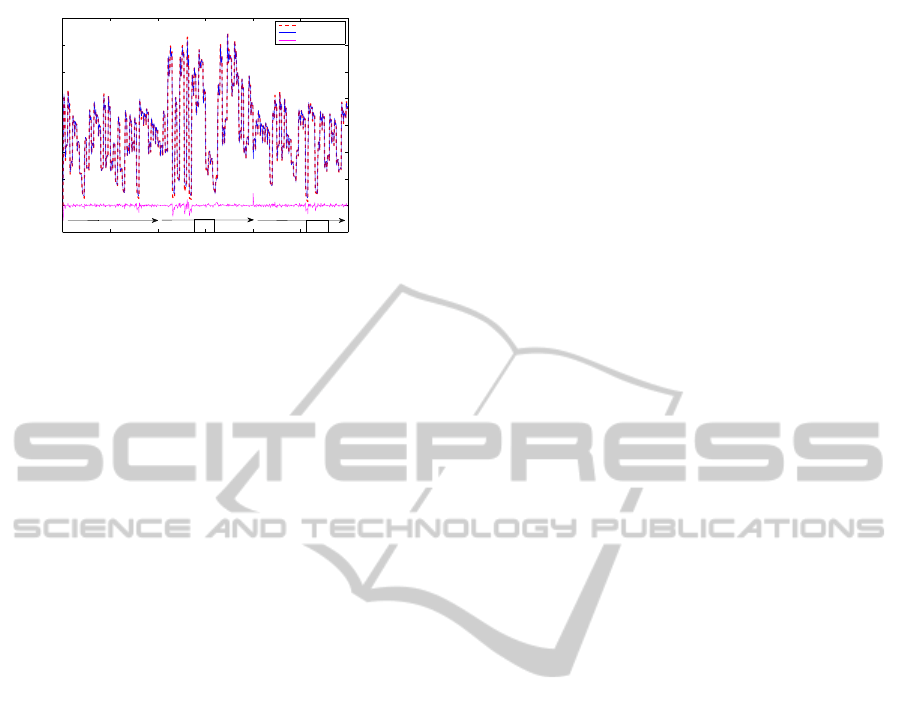
0 200 400 600 800 1000 1200
−0.5
0
0.5
1
1.5
2
2.5
3
3.5
System output
Model output
Identification error
S2
S1
S1
Figure 12: Multi-kernel LS-SVM identification of the
global system.
which is an acceptable performance.
4 CONCLUSIONS
A multi kernel LS-SVM identification method is pro-
posed in this paper. The suggested idea consists on
training an LS-SVM algorithm with multiple kernel
functions. A linear kernel combined with the Gaus-
sian one have been chosen. These two weighted com-
bined kernels were demonstrate a good performances
in the identification context compared with the tra-
dionnal LS-SVM method which uses unique kernel
function.
REFERENCES
Bemporad, A., A. Garulli, S. P., and Vicino, A. (2005).
A bounded-error approach to piecewise affine system
identification. In IEEE Transactions on Automatic
Control, 50: 1567-1580.
Delmotte, F. (1997). Analyse multimod`ele. In Th`ese, UST
de Lille, France.
Diosan, L., Oltean, M., Rogozan, A., and Pecuchet, J.
(2007). Improving svm performance using a linear
combination of kernels. In B. Beliczynski et al. (Eds.):
ICANNGA 2007, Part II, LNCS 4432, pp. 218-227,
2007. Springer–Verlag Berlin Heidelberg 2007.
ElFerchichi, S., Laabidi, K., Zidi, S., and Maouche, S.
(2009). Feature selection using an svm learning ma-
chine. In Signals, Circuits and Systems (SCS).
Goethals, I., Pelckmans, K., Suykens, J., and Moor, B. D.
(2005). Identfication of mimo hammerstein models
using least squares support vector machines. In Sci-
ence direct, automatica 41 (2005) 1263 –1272.
Lanckriet, G. R., Cristianini, N., Bartlett, P., Ghaoui, L. E.,
and Jordan, M. (2004). Learning the kernel matrix
with semidefinite programming. In Journal of Ma-
chine Learning Research, 5, 27-72.
Lauer, F. (2008). From support vector machines to hybrid
system identification. In th`ese doctorat de l’Universit´e
Henri Poincar´e-Nancy1. sp´ecialit´e automatique.
Lei, B., Wang, W., and Li, Z. (2008). On line predictive
control based on ls svm. In Proceedings of the 7th
World Congress on Intelligent Control and Automa-
tion. Chongqing, China.
Messaoud, A., Ltaief, M., and Abdennour, R. B. (2009).
Supervision based on partial predicctors for a mul-
timodel generalised predictive control : exprimental
validation on a semi-batch reactor. In J. Modelling
Identification and control, vol 6 no 4.
Sonnenburg, S., R ¨atsch, G., Schfer, C., and Schlkopf,
B. (2006). Large scale multiple kernel learning.
In Journal of Machine Learning Research 7 (2006)
15311565.
Suykens, J. and J.Vandewalle (1999). Least squares support
vector machine classifier. In Neural Processing Let-
ters 9: 293–300,1999. Kluwer Academic Publishers.
Printed in the Netherlands.
Tao, S., Chen, D., and Zhao, W. (2009). Fast pruning al-
gorithm for multi-output ls-svm and its application in
chemical pattern classification. In Chemometrics and
Intelligent Laboratory Systems 96, 63-69.
Tarhouni, M., Laabidi, K., Zidi, S., and Ksouri, M. L.
(2010). Surveillance des systemes complexes par les
s´eparateurs `a vaste marge. In 8eme Conf´erence Inter-
nationale de MOd´elisation et SIMulation - MOSIM’10
- 10 au 12 mai 2010 - Hammamet - Tunisie.
Valyon, J. and Horvath, G. (2005). A robust ls–svm re-
gression. In Proceeding of word academy of science,
engeneering and technology, Volume 7, 148–53.
Verdult, V., Ljung, L., and Verhaegen, M. (2002). Iden-
tification of composite local linear state-space mod-
els using a projected gradient search. In International
Journal Control, 75 :1385-1398, 2002.
Zhejing, B., Daoying, P., and Youxian, S. (2004). Multi-
ple kernel learning, conic duality, and the smo algo-
rithm machine with multi–kernel. In Proceedings of
the 21
st
International Conference on Machine Learn-
ing, Banff, Canada.
Zhejing, B., Daoying, P., and Youxian, S. (2007). Nonlin-
ear model predictive control based on support vector
machine with multi–kernel. In Chin. J. Chern. Eng.,
15(5) 691–697.
Zidi, S., Maouche, S., and Hammadi, S. (2006). Nou-
velle approche pour la regulation des r´eseaux de trans-
port multimodal. 6eme Conf´erence Francophone de
MOd´elisation et SIMulation , MOSIM’06 ,du 3 au 5
avril 2006, Rabat, Maroc.
Zouari, T., Laabidi, K., and ksouri, M. (2008). Multimodel
approach applied for failures diagnostic. In Interna-
tional Journal of sciences and Techniques of Auto-
matic controle and computer engineeering, IJ–STA,
Volume 2, N1, pp. 500–515.
SYSTEM IDENTIFICATION BASED ON MULTI-KERNEL LEAST SQUARES SUPPORT VECTOR MACHINES
(MULTI-KERNEL LS-SVM)
315