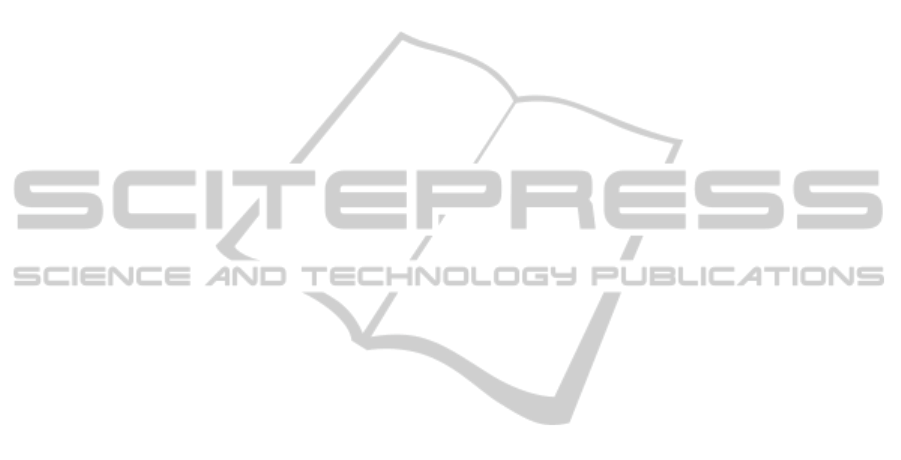
(high beta and gamma). This is probably a
consequence of their fast dynamics. A similar
conclusion was achieved, via a sensitivity analysis,
in another modeling paper (Ursino et al., 2010).
Although the results attained in the present work
are quite satisfactory, the study also exhibits several
limitations, which may become the target of future
improvements or extensions. First, there is no
warranty that the parameter values obtained in this
study are unique. Probably, different combinations
of parameters do exist which provide similar results.
The problem of the uniqueness of parameter
estimates is very complex in all non-linear fitting
procedures. The solution will require the inclusion
of additional knowledge, to constrain parameter
estimates (for instance, the use of additional
anatomical or neurophysiological knowledge, and
the use of Bayesian estimation techniques).
In the present study we used a manual fitting
procedure. An improved fitting may be achieved, in
future works, using an automatic algorithm. This,
however, must deal with the problem of multiple
solutions and with a smart choice of an initial guess.
The experiment was performed on 7 subjects, but
in this pilot study we used data recorded just from
one of them. Fitting to all available data will be
attempted in future works, once an automated
estimation algorithm is available. Comparison
between the connectivity patterns obtained on
different subjects will be of the greatest value to
check the repeatability of the obtained results, and to
understand which connectivity parameters are more
subject dependent.
In the present study, we did not consider
connections between cortical regions and the
thalamus. Conversely, cortico-thalamic connections
are known to play a pivotal role in generating brain
oscillations (Steriade, 2006) as well as in the
transmission of information among cortical regions.
The choice of not including thalamic regions in the
model was motivated by a parsimony reason: we
wished to realize a parsimonious model of a TMS
stimulation experiment, with a reduced number of
regions and of connectivity parameters.
Consequently, a single ROI in the model can be
considered representative not only of cortical
dynamics, but more generally of an entire cortico-
thalamic circuit. Inclusion of an explicit description
of the thalamus may represent a possible model
extension. However, we expect that this enlarged
model would require more data to fit individual
parameters in both cortical and thalamic regions
altogether.
REFERENCES
Brignani, D., Manganotti, P., Rossini, P. M. and Miniussi,
C., (2008). Modulation of cortical oscillatory activity
during transcranial magnetic stimulation. Human
Brain Mapping, 29(5), 603-612.
Esser, S. K., Hill, S. L. and Tononi, G., (2005). Modeling
the effects of transcranial magnetic stimulation on
cortical circuits. Journal of Neurophysiology, 94(1),
622-639.
Fries, P., Nikolic, D. and Singer, W., (2007). The gamma
cycle. Trends in Neurosciences, 30(7), 309-316.
Friston, K., Henson, R., Phillips, C. and Mattout, J.,
(2006). Bayesian estimation of evoked and induced
responses. Human Brain Mapping, 27(9), 722-735.
Fuggetta, G., Pavone, E. F., Fiaschi, A. and Manganotti,
P., (2008). Acute modulation of cortical oscillatory
activities during short trains of high-frequency
repetitive transcranial magnetic stimulation of the
human motor cortex: a combined EEG and TMS
study. Human Brain Mapping, 29(1), 1-13.
Hallett, M., (2007). Transcranial magnetic stimulation: a
primer. Neuron, 55(2), 187-199.
Jansen, B.H. and Rit, V.G., (1995). Electroencephalogram
and visual evoked potential generation in a
mathematical model of coupled cortical columns.
Biological Cybernetics, 73(4), 357-366.
Kaiser, J. and Lutzenberger, W., (2005). Human gamma-
band activity: a window to cognitive processing.
Neuroreport, 16(3), 207-211.
Miniussi, C. and Thut, G., (2010). Combining TMS and
EEG offers new prospects in cognitive neuroscience.
Brain Topography, 22(4), 249-256.
Rosanova, M., Casali A., Bellina V., Resta F., Mariotti M.
and Massimini M., (2009). Natural frequencies of
human corticothalamic circuits. Journal of
Neuroscience, 29(24), 7679-7685.
Steriade, M., (2006). Grouping of brain rhythms in
corticothalamic systems. Neuroscience, 137(4), 1087-
1106.
Tamraz, J. and Comair, Y. (2000). Atlas of regional
anatomy of the brain using MRI. Berlin: Springer.
Thut, G. and Miniussi, C., (2009). New insights into
rhythmic brain activity from TMS-EEG studies.
Trends in cognitive sciences, 13(4), 182-189.
Ursino, M., Cona, F. and Zavaglia, M., (2010). The
generation of rhythms within a cortical region:
Analysis of a neural mass model. Neuroimage, 52(3),
1080-1094.
Virtanen, J., Ruohonen, J., Naatanen, R. and Ilmoniemi,
R.J., (1999). Instrumentation for the measurement of
electric brain responses to transcranial magnetic
stimulation. Med.Biol.Eng Comput., 37(3), 322-326.
Wendling, F., Bartolomei, F., Bellanger, J. J. and Chauvel,
P., (2002). Epileptic fast activity can be explained by a
model of impaired GABAergic dendritic inhibition.
European Journal of Neuroscience, 15(9), 1499-1508.
CORTICAL RHYTHMS INDUCED BY TMS STIMULATION - Analysis with a Neural Mass Model
211