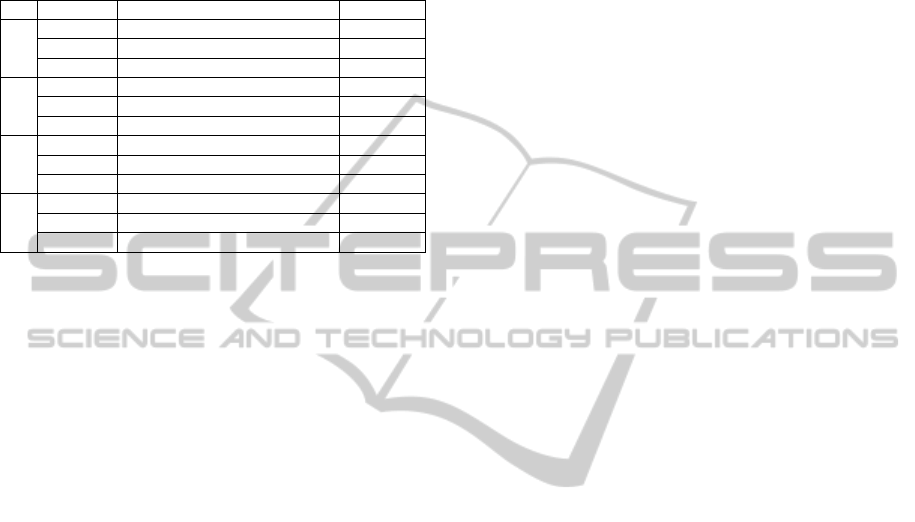
of the population. As the new material is completely
untested, mutation operator often ends up decreasing
the fitness of an individual and increasing the
convergence time.
Table 1: Results from parameter identification – criteria
values and convergence time.
GA Indicator J
J
S
J
J T, s
SMPGA
average 2.3831 1.2583 0.0004 3.6418 316.0752
min time 2.4876 1.2554 0.0004 3.7434 288.1806
max time 2.4363 1.1442 0.0005 3.5810 359.8007
Modif. 1
average 2.4198 1.3892 0.0012 3.8102 277.8347
min time 2.3131 1.5951 0.0008 3.9090 259.2737
max time 2.2126 1.2794 0.0019 3.4939 297.6343
Modif. 2
average 2.4615 1.3631 0.0021 3.8267 203.0400
min time 2.4631 1.6115 0.0003 4.0749 195.3601
max time 2.3878 1.6648 0.0004 4.0530 214.7198
Modif. 3
average 2.4276 1.3830 0.0023 3.8130 240.6050
min time 2.5629 1.5171 0.0043 4.0844 208.8073
max time 2.4597 1.0401 0.0008 3.5005 264.4685
When the operator mutation is eliminated in the
proposed in (Roeva, 2006) modification of GA –
MPGA Modification 3, the convergence time is
decreased compared to MPGA Modification 1 –
from 259.27 s to 208.80 s. The proposed MPGA
Modifications 2 and 3 considerably decrease the
convergence time of GA, and in the same time the
increase of the error is slightly smaller.
5 CONCLUSIONS
Based on performed numerical experiments the
following conclusions for the performance of the
examined MPGA could be generalized:
1. Applying MPGA Modification 1, the estimates of
the considered model parameters with highest
accuracy are obtained. The value of the optimization
criterion J is 3.4939 obtained for a time of 297.6343
s.
2. By the MPGA Modification 2 the best
convergence time is achieved. The average results
are: J = 3.8267 and T = 203.04 s. The obtained
minimal time for solution finding is 195.36 s with an
optimization criterion value of 4.0749.
As a result from the conducted experiments and
analysis of the received data the multi-population
genetic algorithm without mutation (MPGA
Modification 2) is defined as suitable for on-line
application for optimization and control of
bioprocesses. This is the algorithm with the best
convergence time and in the same time the accuracy
of the model is comparable with the higher accuracy
achieved by MPGA Modification 1.
ACKNOWLEDGEMENTS
This work is partially supported by the National
Science Fund Grants DMU 02/4 and DID-02-29.
REFERENCES
Alsumait, J. S., Sykulski, J. K., Al-Othman, A. K., 2010.
A hybrid GA–PS–SQP method to solve power system
valve-point economic dispatch problems, Applied
Energy, 87, 1773-1781.
Arndt, M., Kleist, S., Miksch, G., Friehs, K., Flaschel, E.,
Trierweiler, J., Hitzmann, B., 2004. A feedforward-
feedback substrate controller based on a kalman filter
for a fed-batch cultivation of Escherichia coli
producing phytase, Computers and Chemical
Engineering, 29(5), 1113-1120.
Crueger, W., Grueger, D., 1984. Biotechnology. A
textbook of industrial microbiology. Sci. Tech. Inc.
Goldberg, D., 1989. Genetic algorithms in search,
optimization and machine learning. Addison-Weslcy
Publishing Company, Reading, Massachusetts.
Kim, D. H., Abraham, A., Cho, J. H., 2007. A hybrid
genetic algorithm and bacterial foraging approach for
global optimization, Information Sci., 177, 3918-3937.
Na, J.-G., Chang, Y. K., Chung, B. H., Lim, H. C., 2002.
Adaptive Optimization of fed-batch culture of yeast by
using genetic algorithms, Bioprocess and Biosystems
Engineering, 24, 299-308.
Roeva, O., 2006. A modified genetic algorithm for a
parameter identification of fermentation processes,
Biotech. & Biotechnol. Equipm. 20(1), 202-209.
Roeva, O., 2007. Multipopulation genetic algorithm: A
tool for parameter optimization of cultivation
processes models, LNCS, Springer, 4310, 255-262.
Roeva, O., Tzonkov, St., 2009. A genetic algorithm for
feeding trajectory optimization of fed-batch
fermentation processes, Int. J. Bioautomation, 12, 1-
12.
Rowe, J., East, I., 1995. Direct replacement: A genetic
algorithm without mutation which avoids deception,
Lecture notes in Artificial Intel., Springer, 956, 41-48.
Louis, S. J., Rawlins, G. J. E., 1993. Predicting
convergence time for genetic algorithms, Technical
Report TR370, Department of Computer Science,
Indiana University, https://www.cs.indiana.edu/cgi-
bin/techreports/TRNNN.cgi?trnum=TR370.
A MODIFIED MULTI-POPULATION GENETIC ALGORITHM FOR PARAMETER IDENTIFICATION OF
CULTIVATION PROCESS MODELS
351