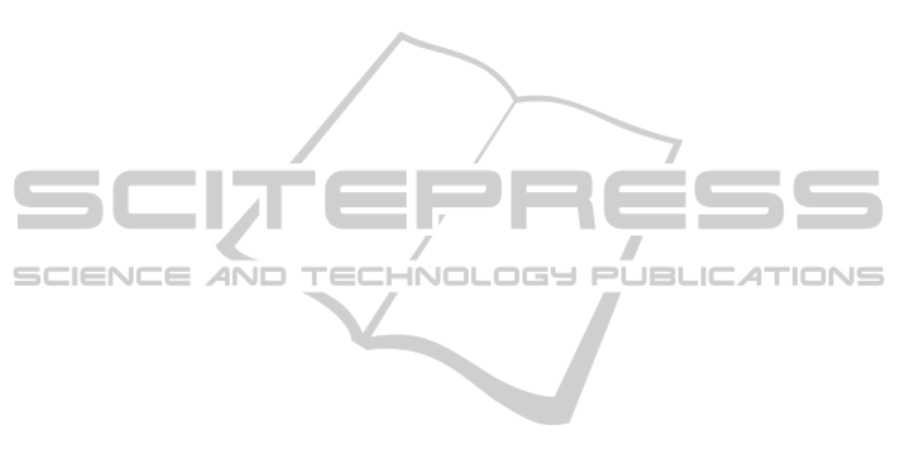
short learning rate. That is, the results obtained when
representing the time in months differ from those in
which the time is represented in years, despite the fact
this is just a simple scaling.
6 FUTURE WORK
In the future, we aim to investigate how to restrict the
constant value of the singleton output of the rules pro-
ducing by the ANFIS to be all positive, so that we can
obtain a smooth curve of conditional probability with
non-negative values in any of the time intervals.
Further investigations into the effects of scaling
the inputs to the ANFIS model will also be under-
taken, to see whether there are any significant effects
on learning rate and/or final membership functions.
We also aim to create ANFIS models for other
clinical data sets — we have recently obtained data for
a cohort of over 400 colorectal cancer patients with
ten year follow-up survival data.
ACKNOWLEDGEMENTS
The authors thank all members of the Nottingham
Breast Cancer Pathology Research Group, and par-
ticularly Prof. Ian Ellis, Dr Andy Green and Dr Des
Powe, for their help in preparing and providing the
data set used in this study.
This study was supported by the Ministry of
Higher Learning, Malaysia and Universiti Putra
Malaysia (UPM).
REFERENCES
Biganzoli, E., Boracchi, P., Mariani, L., and Marubini, E.
(1998). Feed forward neural networks for the analysis
of censored survival data: a partial logistic regression
approach. Statistics in Medicine, 17(10):1169–1186.
Bishop, C. M. (2007). Neural Networks for Pattern Recog-
nition. Oxford University Press, UK.
Burke, H., Goodman, P., Rosen, D., Henson, D., Weinstein,
J., Harrell, F., Marks, J., Winchester, D., and Bost-
wick, D. (1997). Artificial neural network improve
the accuracy of cancer survival prediction. Cancer,
79(4):857–862.
Cancer Research UK (2010). Uk breast cancer incidence
statistics. Date last accessed: 18/05/2010.
Galea, M., Blamey, R., Elston, C., and Ellis, I. (1992). The
nottingham prognostic index in primary breast cancer.
Breast Cancer Research and Treatment, 22(3):207–
219.
Hamdan, H. and Garibaldi, J. M. (2010). Adaptive neuro-
fuzzy inference system (ANFIS) in modelling breast
cancer survival. In 2010 IEEE International Confer-
ence on Fuzzy Systems (FUZZ-IEEE), pages 573–580.
Jang, J.-S. (1993). Anfis adaptive-network-based fuzzy in-
ference system. IEEE Transactions on Systems, Man
and Cybernetics, 23(3):665–685.
Joseph, A. C. and David, S. W. (2006). Applications of
machine learning in cancer prediction and prognosis.
Cancer Informatics, 2:59–78.
Lisboa, P. J. G. (2002). A review of evidence of health bene-
fit from artificial neural networks in medical interven-
tion. Neural Networks, 15(1):11–39.
Mamdani, E. H. and Assilian, S. (1975). An experiment
in linguistic synthesis with a fuzzy logic controller.
International Journal of Man-Machine Studies,, 7:1–
13.
Negnevitsky, M. (2005). Artificial Intelligence: a guide to
intelligent systems. Pearson Education Limited, Es-
sex, England.
Ramesh, A. N., Kambhampati, C., Monson, J. R. T., and
Drew, P. J. (2004). Artificial intelligence in medicine.
Annals of The Royal College of Surgeons of England,
86:334–338.
Sugeno, M. (1985). Industrial applications of fuzzy control.
Elsevier Science Pub. Co.
WHO (2010). Cancer. Date last accessed: 18/05/2010.
Yardimci, A. (2009). Soft computing in medicine. Applied
Soft Computing, 9(3):1029 – 1043.
Zadeh, L. A. (1969). Biological application of the theory
of fuzzy sets and systems. In Proceeding of the Inter-
national Symposium of Biocybernetics of the Central
Nervous System, pages 199–212.
ICFC 2010 - International Conference on Fuzzy Computation
104