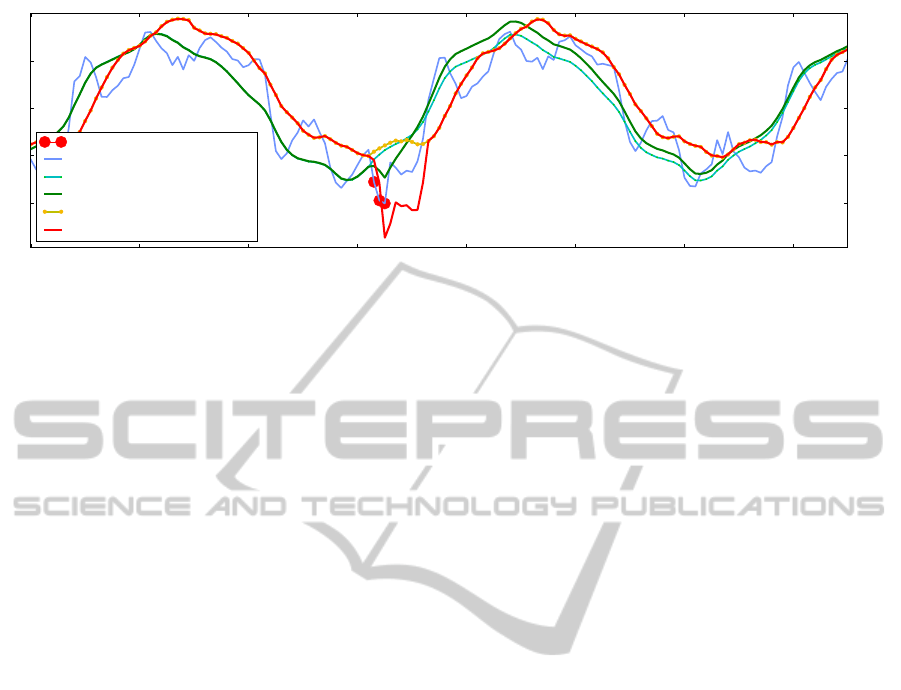
300 320 340 360 380 400 420 440
−2
−1
0
1
2
IIR vs. SFA: Simulated short-term disturbances
Simulated disturbance
SLx_fr/SRx_fr mix
IIR filter mix
IIR filter mix with disturbance
SFA y
1
SFA y
1
with disturbance
Figure 5: Comparing the response to a short disturbance of the IIR filtered signal and the slowest component extracted by
SFA.
of undesirable configurations of the robot.
Future work will focus on how the achieved in-
crease in reactivity can be efficiently used for the im-
provement of the safety of the gait pattern. Several ap-
proaches are conceivable, e.g., the reduction of motor
activity as soon as the SFA signal leaves its allowed
range. Also one could imagine to use predictors that
are trained on the SFA component; a high prediction
error would then indicate upcoming problems.
Another promising investigation is the online
adaption of the calculated SFA component by an
adaptive LMS algorithm as mentioned in 4.2. This
would prove helpful in cases when the robot’s sensors
are exchanged and therefore slight decalibration may
occur.
In addition, further investigation will be carried
out on the applicability of SFA to other use cases for
humanoid robotics. The newly available successor of
the A-series platform, the Myon robot, is equipped
with a higher amount and additional modalities of
sensors, like pressure sensors located in the feet, etc.
Considering the results hitherto, SFA can prove useful
for the extraction of robust high level abstract features
that meaningfully describe the robot’s states on one
hand, and stabilise robot control on the other hand.
ACKNOWLEDGEMENTS
This work has been supported by the European re-
search project ALEAR (FP7, ICT-214856).
REFERENCES
Aizerman, A., Braverman, E. M., and Rozoner, L. I.
(1964). Theoretical foundations of the potential func-
tion method in pattern recognition learning. Automa-
tion and Remote Control, 25:821–837.
Berkes, P. (2006). Temporal slowness as an unsupervised
learning principle. PhD thesis, Humboldt-Universit
¨
at
zu Berlin.
Berkes, P. and Wiskott, L. (2002). Applying Slow Feature
Analysis to Image Sequences Yields a Rich Reper-
toire of Complex Cell Properties. In Dorronsoro,
J. R., editor, Proc. Intl. Conf. on Artificial Neural Net-
works - ICANN’02, Lecture Notes in Computer Sci-
ence, pages 81–86. Springer.
Berkes, P. and Wiskott, L. (2006). On the analysis and inter-
pretation of inhomogeneous quadratic forms as recep-
tive fields. Neural Computation, 18(8):1868–1895.
Franzius, M., Sprekeler, H., and Wiskott, L. (2007). Slow-
ness and sparseness lead to place, head-direction,
and spatial-view cells. PLoS Computational Biology,
3(8):e166.
Lau, S., Leung, S., and Chan, B. (1992). A reduced rank
second-order adaptive volterra filter. In ISSPA 92, Sig-
nal Processing and its Applications, pages 561–563,
Gold Coast, Australia.
Mathews, J. (1991). Adaptive polynomial filters. IEEE Sig-
nal Processing Magazine, 8(3):10–26.
Pfeifer, R. and Bongard, J. C. (2006). How the Body Shapes
the Way We Think: A New View of Intelligence (Brad-
ford Books). The MIT Press.
Spranger, M., H
¨
ofer, S., and Hild, M. (2009). Biologically
inspired posture recognition and posture change de-
tection for humanoid robots. In Proc. IEEE Interna-
tional Conference on Robotics and Biomimetics (RO-
BIO), pages 562–567, Guilin, China.
Wiskott, L. (1998). Learning Invariance Manifolds. In Proc.
of the 5th Joint Symp. on Neural Computation, May
16, San Diego, CA, volume 8, pages 196–203, San
Diego, CA. Univ. of California.
Wiskott, L. (2003). Estimating Driving Forces of Nonsta-
tionary Time Series with Slow Feature Analysis.
Wiskott, L. and Sejnowski, T. (2002). Slow Feature Anal-
ysis: Unsupervised Learning of Invariances. Neural
Computation, 14(4):715–770.
Wyss, R., K
¨
onig, P., and Verschure, P. F. M. J. (2006). A
model of the ventral visual system based on temporal
stability and local memory. PLoS Biol, 4(5).
Zaknich, A. (2005). Principles of adaptive filters and self-
learning systems. Springer London.
Zito, T., Wilbert, N., Wiskott, L., and Berkes, P. (2009).
Modular toolkit for Data Processing (MDP): a Python
data processing frame work.
USING SLOW FEATURE ANALYSIS TO IMPROVE THE REACTIVITY OF A HUMANOID ROBOT'S
SENSORIMOTOR GAIT PATTERN
219