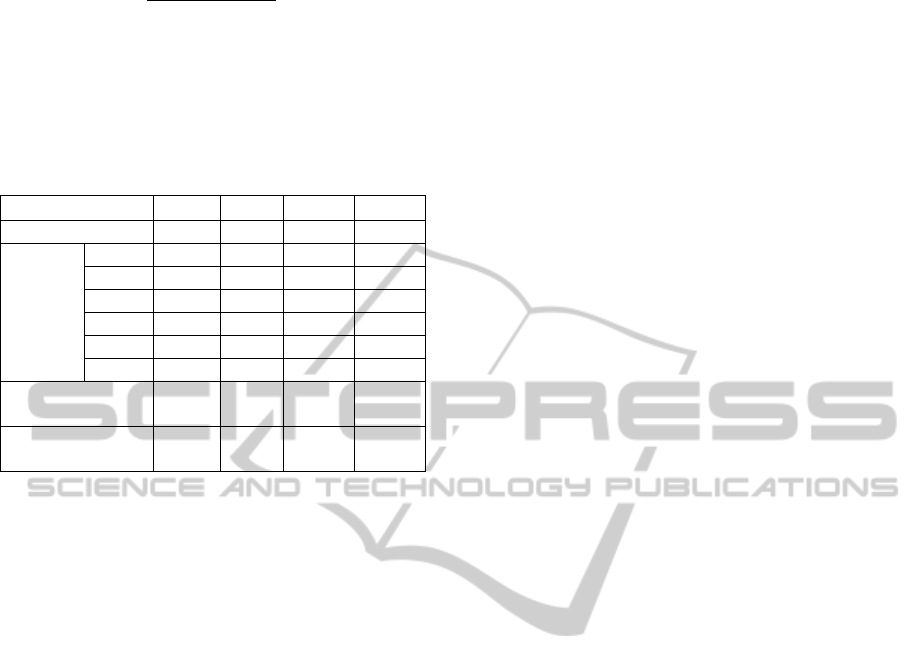
()
()
()
2
3
1366
4.5cos25.12.3
,
−+
+
=
x
y
yxS
(17)
()
()
()
22
4
,sin5Sxy x y x=−
(18)
Table 2: Comparison between the MSE of CWN,
MMWNN and MMWNN-GA in term of 2D functions
approximation.
Function S1 S2 S3 S4
Nb of wavelets 17 19 14 9
CWNN
(100
iterations)
Mexhat 2.58e-3 1.00e-2 4.93e-2 1.05e-2
Pwog1 4.06e-3 1.73e-2 4.94e-2 1.08e-3
Slog1 2.31e-3 2.60e-3 4.88e-2 6.37e-3
Beta1 4.22e-3 1.44e-2 4.63e-2 2.25e-4
Beta2 2.80e-3 6.19e-3 4.56e-2 3.94e-4
Beta3 4.23e-3 6.19e-3 4.65e-2 4.85e-4
MLWNN
(100 iterations)
3.49e-7 1.50e-5 2.54e-4 8.4e-3
MMWNN-GA
(40iterations )
8.48e-7 5.78e-7 7.89e-4 4.86e-3
From table 2, we can see that MLWNN-GA are
more suitable for 2D function approximation then
the others wavelets neural networks.
For example we have an MSE equal to 8.4877e-7
to approximate the surface S1 using MMWNN-GA
after 40 iterations over 2.5803e-3 if we use the
Mexican hat wavelet after 100 iterations.
The MMWNN approximates S2 with an MSE
equal to 1.50e-5 where the MSE using the
MLWNNGA is 5.78e-7.
For S3, the MSE is equal to 4.65e-2 for Beta3
WNN comparing to 7.89e-4 for MLWWN-GA.
Finally, Slog1 approximates S4 with MSE equal
to 6.37e-3 comparing to 4.86e-3 with MMWNN-
GA.
5 CONCLUSIONS
In this paper, we presented a genetic algorithm for
the design of wavelet network.
The problem was to find the optimal network
structure and parameters. In order to
determine the
optimal network, the proposed algorithms modify
the number of wavelets in the library.
The performance of the algorithm is achieved by
evolving the initial population and by using
operators that alter the structure of the wavelets
library.
Comparing to classical algorithms, results show
significant improvement in the resulting
performance and topology.
As future work, we propose to combine this
algorithm with GCV (Othmani, Bellil, Ben Amar
and Alimi, 2010) to optimize the number of wavelets
in hidden layer.
REFERENCES
Bellil, W. Othmani, M. and Ben Amar, C, 2007.
Initialization by Selection for multi library wavelet
neural network training. ICINCO, 30-37.
Bellil, W. Ben Amar, C. Zaied, M. and Alimi, M. A.
,2004. La fonction Beta et ses dérivées : vers une
nouvelle famille d’ondelettes, In SCS’04, 1st
International Conference on Signal, System and
Design (vol. 1, pp.201-207). Tunisia.
Colla, V. Reyneri, L. M and Sgarbi,M., 1999. Orthogonal
least squares algorithm applied to the initialization of
multi-layer perceptrons, In ESANN’1999 Proc.
European Symposium on Artificial Neural
Networks(pp. 363–369). Bruges:Belgium.
Daubechies, I. ,1992. Ten Lectures on Wavelets. Society
for Industrial and Applied Mathematics. Philadelphia:
Pennsylvania.
Gruau, F. and Whitley, D., 1993. Adding learning to the
cellular developmental process: a comparative study.
Evolutionary Computation, 1(3).
Lee, D., 1999. An application of wavelet networks in
condition monitoring. IEEE Power Engineering.
Review 19, 69-70.
Michalewicz, Z., 1993. A Hieararchy of Evolution
Programs: An Experimental Study, Evolutionnary
Computation, 1.
Othmani, M. Bellil, W. Ben Amar, C. and Alimi, M. A.,
2010. A new structure and training procedure for
multi-mother wavelet networks. International Journal
of Wavelets, Multiresolution and Information
Processing (IJWMIP), 1, World Scientific Publishing
Company (vol. 8pp.149-174).
Qian, S. and Chen, D., 1994. Signal representation using
adaptative normalized gaussian function. Signal
prossing (vol. 36).
Titsias, M. K. and Likas, A. C. (2001). Shared kernel
model for class conditional density estimation, IEEE
Trans. Neural Network, 12(5), 987–996.
Withleyet al, D., 1990. Genetic Algorithms and Neural
Networks: Optimizing Connections and Connectivity.
Parallel Computing, 14.
Yan, R. and Gao, R. X., 2009. Base wavelet selection for
bearing vibration signal analysis. Int. J. Wavelets
Multiresolut. Inf. Process. 7(4), 411–426.
Zhang, Q., 1997. Using wavelet network in nonparametric
estimation. IEEE Trans. Neural Networks, 8(2), 227–
236.
Zhang, Q. and Benveniste, A., 1992. Wavelet networks.
IEEE Trans. Neural Networks, 3(6), 889–898.
ICFC 2010 - International Conference on Fuzzy Computation
434