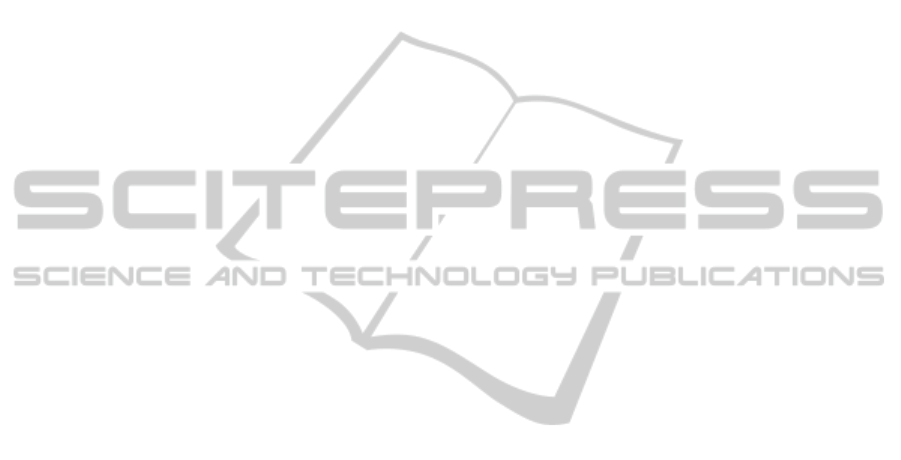
human-like limb consisting of arm and shoulder-
girdle can be modelled by a comparatively simple
closed-loop control schema that comprises small
neural network modules and physiological
movement synergies.
In classical studies, only artificial or reduced data
have been used as a basis for the training of neural
networks for motor control (e.g., Massone and Bizzi,
1989, Kawato, et al., 1990, Massone and Myers
1994, Karniel and Inbar, 1997). More recent efforts
to model reaching movements based on ANNs do
take a physiologically oriented approach (Koike, et
al., 2006, Choi, et al., 2009) but still somewhat
neglect the importance of motor primitives or
synergies.
In contrast, numerous studies find evidence in
favor of a modular organization of the nervous
system (e.g. Mussa-Ivaldi, et al., 1994, d'Avella, et
al., 2006). Although these studies propose potential
CNS structures that might be important for motor
primitives, these studies often keep silent with
regard to more concrete neural models and how
exactly movement modules might be combined in a
task- or goal-dependent manner in order to produce
meaningful behavior.
The approach presented here tries to
accommodate both aforementioned aspects: We
combine a connectionist approach based on ANNs
with experimentally observed movement synergies
during a natural reaching task. Combining several
DoFs within one synergies and thereby reducing the
complexity of the control problem allows us to
exploit a bijective mapping between movement
synergy space and task space.
Comparative evaluation of the two controllers
indicates that, for the present problem, the recurrent
Elman network is less appropriate, owing to
insufficient end-point stability. Given, that the
context layer could be interpreted as an internal
model, and that internal models are assumed to be an
important computational element central to nervous
motor control (Wolpert & Ghahramani, 2000), this is
somewhat surprising.
Another notable aspect of the control schema
presented here is the low number of necessary
neuronal units. A feed-forward network with five
hidden units seems to be sufficient for the task of
accurately controlling three movement synergies.
There are two possible explanations for this: On the
one hand, the dissociation of the neuronal substrate
into two distinct modules, i.e. into a controller ANN
and a synergy network, might be more efficient that
a monolithic architecture of similar size. On the
other hand, the approach described here is solely
based on joint angle kinematics and neglects a
further potential source of complexity:
transformation of movement kinematics into a
muscle activation pattern. Again, this transformation
is a one-to-many mapping and might exacerbate the
necessary computations.
REFERENCES
Atkeson, C. G., Hollerbach, J. M. (1985). Kinematic
features of unrestrained vertical arm movements. The
Journal of Neuroscience 5, 2318 – 2330.
Bernstein, N. (1967). The co-ordination and regulation of
movements. New York: Pergamon Press Ltd.
Bockemühl, T., Troje, N. F., and Dürr, V. (2010). Inter-
joint coupling and joint angle synergies of human
catching movements. Human Movement Science 29,
73 – 93.
Choi, K., Hirose H., Sakurai, Y., Iijima, T., Koike, Y.
(2009). Prediction of arm trajectory from the neural
activities of the primary motor cortex with modular
connectionist architecture. Neural Networks 22, 1214
– 1223.
d’Avella, A., Portone, A., Fernandez, L., Lacquaniti, F.
(2006). Control of Fast-Reaching Movements by
Muscle Synergy Combinations. The Journal of
Neuroscience 26, 7791 – 7810.
Elman, J. L. (1990). Finding Structure in Time. Cognitive
Science 14, 179 – 211.
Flash, T., Hochner, B. (2005). Motor primitives in
vertebrates and invertebrates. Current Opinion in
Neurobiology 15, 660 – 666.
Flash, T., Hogan, N. (1985). The coordination of arm
movements: An experimentally confirmed
mathematical model. The Journal of Neuroscience, 5,
1688 – 1703.
Karniel A., Inbar G. (1997). A model for learning human
reaching movements. Biological Cybernetics 77, 173 –
183.
Kawato, M., Maeda Y., Uno, Y., Suzuki R. (1990).
Trajectory formation of arm movement by cascade
neural network model based on minimum torque-
change criterion. Biological Cybernetics 62, 275 –
288.
Koike, Y., Hirose, H., Sakurai, Y., Iijima, T. (2006).
Prediction of arm trajectory from a small number of
neuron activities in the primary motor cortex.
Neuroscience Research 55, 146 – 153.
Levenberg, K. (1944). A method for the solution of certain
nonlinear problems in least squares. Quarterly Journal
of Applied Mathematics 2, 164 – 168.
Marquardt, D. W. (1963). An algorithm for least-squares
estimation of nonlinear parameters. Siam Journal on
Applied Mathematics 11, 431 – 441.
Massone, L., Bizzi, E. (1989). A neural network model for
limb trajectory formation. Biological Cybernetics 61,
417 – 425.
A MINIMAL CONTROL SCHEMA FOR GOAL-DIRECTED ARM MOVEMENTS BASED ON PHYSIOLOGICAL
INTER-JOINT COUPLINGS
225