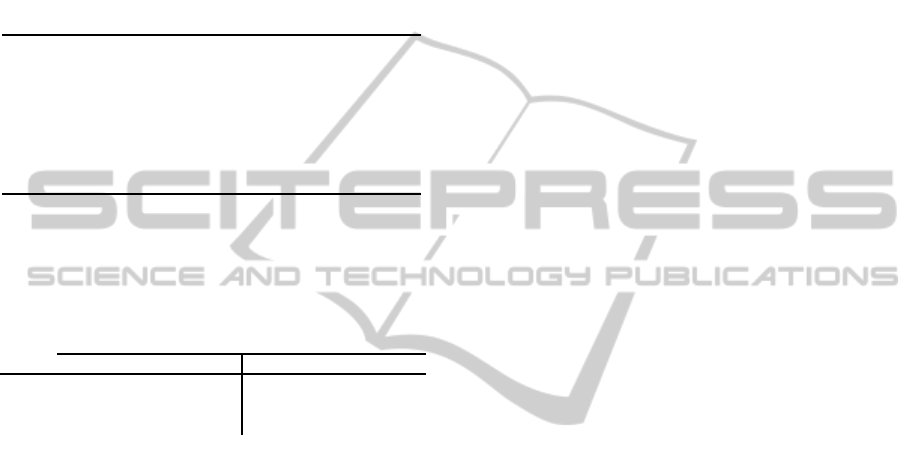
4.3 Parameter Settings
As stated before, the control parameters are never
set in advance and are not constant. They are de-
termined each time on the basis of statistical prop-
erties of each population. Besides the population size
changes through the search progress - to enable the
search with different population sizes.
Table 1: Results of optimization for Task 1.
0% 5% 10% 25%
Best 1.314×10
8
2.017×10
8
2.316×10
8
3.227×10
8
Mean 1.319×10
8
2.037×10
8
2.561×10
8
3.417×10
8
Worst 1.325×10
8
2.117×10
8
2.819×10
8
3.738×10
8
StD 3.495×10
5
4.039×10
6
1.531×10
7
1.821×10
7
Table 2: Results of optimization for Task 2.
0% 5% 10% 25%
Best 1.616×10
8
1.934×10
8
1.837×10
8
4.054×10
8
Mean 1.663×10
8
2.149×10
8
2.381×10
8
5.051×10
8
Worst 1.723×10
8
2.421×10
8
2.833×10
8
6.249×10
8
StD 4.814×10
6
1.592×10
7
3.744×10
7
5.836×10
7
Table 3: Comparison of delayed orders.
Task 1 Task 2
0% 5% 10% 25% 0% 5% 10% 25%
Best 13 20 23 32 16 19 18 40
Mean 13 20 25 34 16 21 23 50
Worst 13 21 28 37 17 24 28 62
4.4 Results
In Tables 1 and 2 best, mean, worst, and standard de-
viations of solutions are presented for each ratio of
fixed deadlines.
To show how some of the components of the cost
function behave during the search process, we present
Figures 1 and 2. It can be visually seen, how the num-
ber of orders with fixed deadlines influences the per-
formance of PLES+LS. Here, each line represent one
run. The number of delayed orders is increasing with
the growing ratio of fixed-deadline orders.
When comparing with the previous approach
(Koroˇsec et al., 2010) of production planning, the in-
fluence of fixed orders is seen on the number of de-
layed orders. The details are presented in Table 3.
5 CONCLUSIONS AND FUTURE
WORK
In this paper, we have shown an application of spe-
cialized hybrid parameter-less evolutionary algorithm
on a real-world production planning problem. The
presence of orders with fixed deadlines influences
the performance of the hybrid PLES+LS algorithm.
However, even at 25% of orders with fixed deadlines,
the results are still better than those provided by the
expert (Koroˇsec et al., 2010).
REFERENCES
B¨ack, T. (1996). Evolutionary Algorithms in Theory and
Practice. Oxford University Press.
Brest, J., Mernik, S. G. B. B. M., and
ˇ
Zumer, V. (2006).
Self-adapting control parameters in differential evolu-
tion: A comparative study on numerical benchmark
problems. IEEE Transactions on Evolutionary Com-
putation, 10(6):646–657.
Brucker, P. (1998). Scheduling algorithms. Springer, Hei-
delberg, 2nd edition.
Caumond, A., Lacomme, P., and Tchernev, N. (2008). A
memetic algorithm for the job-shop with time-lags.
Comput. Oper. Res., 35(7):2331–2356.
Chryssolouris, G. and Subramaniam, V. (2001). Dynamic
scheduling of manufacturing job shops using genetic
algorithms. Journal of Intelligent Manufacturing,
12(3):281–293.
Goldberg, D. (1989). Genetic Algorithms in Search, Opti-
mization, and Machine Learning. Addison-Wesley.
Harik, G. and Lobo, F. (1999). A parameter-less genetic
algorithm. In Proc. Genetic and Evolutionary Com-
putation Conference (GECCO 1999), pages 258–265.
Hasan, S. M. K., Sarker, R., Essam, D., and Cornforth,
D. (2009). Memetic algorithms for solving job-shop
scheduling problems. Memetic Computing, 1(1):69–
83.
Koroˇsec, P., Papa, G., and Vukaˇsinovi´c, V. (2010). Applica-
tion of memetic algorithm in production planning. In
Bioinspired Optimization Methods and their Applica-
tions, pages 163–175.
Ong, Y. and Keane, A. (2004). Meta-lamarckian learning
in memetic algorithms. IEEE Transactions on Evolu-
tionary Computation, 8(2):99–110.
Papa, G. (2008). Parameter-less evolutionary search. In
Proc. Genetic and Evolutionary Computation Confer-
ence (GECCO’08), pages 1133–1134.
Senthilkumar, P. and Shahabudeen, P. (2006). Ga based
heuristic for the open job shop scheduling problem.
The International Journal of Advanced Manufactur-
ing Technology, 30(3-4):297–301.
Vazquez, M. and Whitley, L. D. (2000). A comparison of
genetic algorithms for the static job shop scheduling
problem. In Parallel Problem Solving from Nature,
pages 303–312. Springer.
ICEC 2010 - International Conference on Evolutionary Computation
236