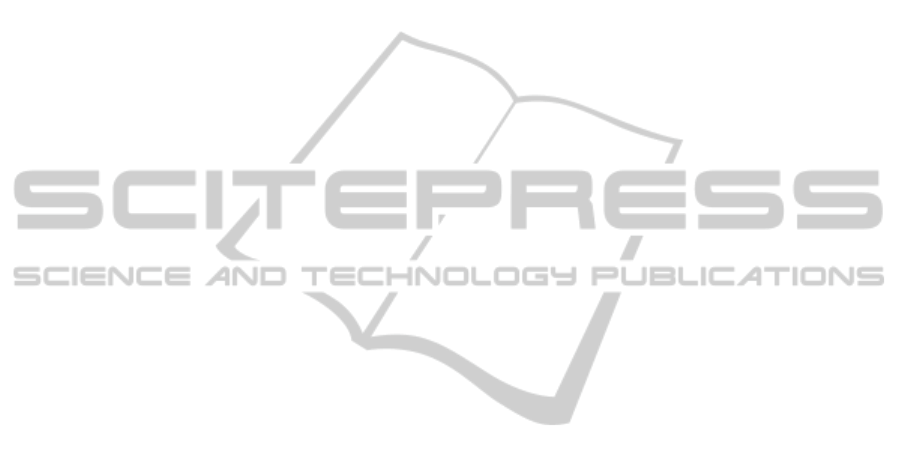
tations for the candidate solutions (respectively at-
tribute grammars and straight line programs). In ad-
dition, SPL-GP uses genetic operators that preserve
the structure of the “semantic blocks”. Even although
preserving semantic blocks is not the only difference
between AGE and SPL-GP, we think that a first com-
parison of both methods as they are defined could pro-
vide some information about the effect on the perfor-
mance of keeping semantic blocks invariant. One of
our future goals is to define an AGE extension adding
a mechanism able to keep the semantic blocks in-
spired by SPL-GP.
2 INTRODUCTION TO SLP-GP
It is easy to see that any program (algorithm) can be
expressed as a sequence of predefined atomic oper-
ations. A standard way of representing each opera-
tion is by means of vectors with n + 2 components.
The first component usually holds the operation per-
formed. Another element refers to the place where the
result will be stored (it usually is a different and new
variable for each operation). There are n additional
components for a maximum of n operands.
Quadruples belong to this kind of representations
(n+ 2 vectors with four elements, n = 2).
For example, the expression 2x
2
(x
1
+ 1)
2
− 2x
2
could be represented by the following sequence of
quadruples: { u
1
:= x
1
+ 1 , u
2
:= u
1
∗ u
1
, u
3
:=
x
2
+ x
2
, u
4
:= u
2
∗ u
3
, u
5
:= u
4
− u
3
}
It is easy to see that the semantic block of a given
element (quadruple) contains all the elements of the
SLP needed to properly evaluate it. For example: the
semantic block of the second quadruple (u
2
:= u
1
∗u
1
)
is the sequence { u
1
:= x
1
+ 1 , u
2
:= u
1
∗ u
1
}, while
the semantic block of the third element (u
3
:= x
2
+x
2
)
contains only this quadruple and the semantic block
of the last quadruple is the complete SLP.
Further details of SLP-GP and examples of its
crossover operator can be found in (Alonso et al.,
2008). The reader can find there a more formal ap-
proach and definitions of slp’s.
3 INTRODUCTION TO AGE
Attribute grammars (Knuth, 1968) are one of the tools
used to completely describe high level programming
languages (both their syntax and their semantics).
AGE (de la Cruz et al., 2005) is an extension to
Grammatical Evolution (ONeill and Conor, 2003).
Both techniques are automatic programming evolu-
tionary algorithms independent of the target program-
ming language, and include a standard representation
of genotypes as strings of integers (codons), and a for-
mal grammar (respectively attribute and context free
grammars) as inputs for the deterministic mapping of
a genotype into a phenotype. This mapping mini-
mizes the generation of syntactically (and in the case
of AGE also semantically) invalid phenotypes. Ge-
netic operators act at the genotype level, while the fit-
ness function is evaluated on the phenotypes.
Further details, deeper descriptions and examples
can be found in (de la Cruz et al., 2005).
4 SLP-GP VS. AGE
There are several differences that make it difficult to
compare both methods. We can briefly summarize
them as follows
• Length of Genotypes. SLP-GP uses slp’s
of “length” 12 (with 12 operations or instruc-
tions) while AGE uses genotypes with variable
length. Like other variable length evolutionary
algorithms, AGE suffers from bloating (the un-
bounded increase in the length of the genotypes
while the search evolves). Pruning (removing
some fragments of genotypes) is a genetic oper-
ator useful to control bloating. AGE removes the
codons not used after the translation into pheno-
type.
• Mapping from Genotype to Phenotype. AGE
includes a mapping from genotype to phenotype
driven by the attribute grammar that generates the
language of candidate solutions. SLP-GP uses
slp’s both as genotypes and as phenotypes. In
AGE, the number of codons (length of the corre-
sponding genotype) needed to generate a pheno-
type of a given length depends on the structure of
the specific grammar and it is, consequently, more
difficult to estimate.
• The Way in which Computational Effort is
Computed. In (Alonso et al., 2008) the number
of basic operations is used to measure the com-
putational effort. Each slp has 12 operations and
each generation contains 200 different genotypes.
Therefore, SLP-GP could compute the computa-
tional effort either by means of the total number
of basic operations, candidate solutions or gener-
ations needed to find the solution, because all of
them are directly proportional. In (Alonso et al.,
2008) the maximum number of basic operation is
10
7
. This condition is equivalentto a maximum of
5000 generations. Nevertheless, as we have pre-
viously introduced for AGE, it is difficult to es-
ICEC 2010 - International Conference on Evolutionary Computation
366