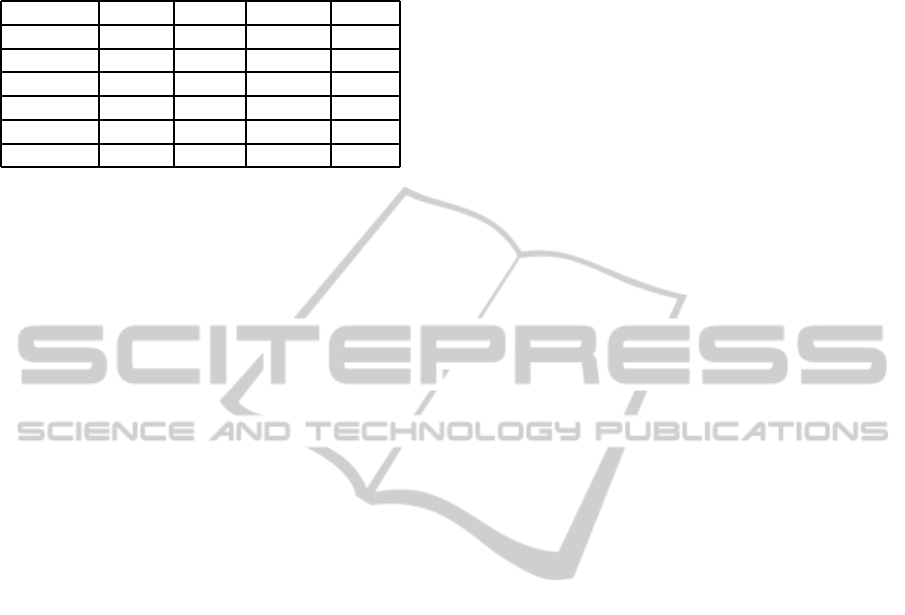
Table 1: Comparison between the particle-cloud modalities
and the reference misfit values found in the literature (Birge,
2003) for different benchmark functions in 50 dimensions.
Median Griewank Rastrigin Rosenbrock Sphere
Standard PSO 9.8E-03 81 90 6.9E-11
PSO 9.6E-03 92 86 8.9E-19
CC-PSO 7.4E-03 99 90 1.0E-15
CP-PSO 1.8E-02 86 223 2.0E-07
PP-PSO 1.0E-01 91 251 8.4E-02
RR-PSO 1.2E-02 39 89 2.9E-25
4 CONCLUSIONS
In this paper we present two more different members
of the PSO family: the PP-GPSO and the RR-GPSO.
Both versions are deduced from the PSO continuous
model adopting respectively a progressive and a re-
gressive discretization in velocities and accelerations.
Although they are PSO-like versions, PP-GPSO has
the same velocity update than GPSO and RR-GPSO
has the form of a PSO with constriction factor, its be-
havior is very different from the PSO case. Particu-
larly the the best parameters sets of the RR-PSO are
concentrated along a straight line located in the com-
plex zone of the first order stability region, but are
not in direct relation with the upper limit of the sec-
ond order stability zone. This behavior is very differ-
ent from others family members including PP-PSO.
The numerical comparison between all the members
of the PSO family using their corresponding cloud-
algorithmshas shown that RR-PSO has a veryimpres-
sive convergence rate while PP-PSO is a more explo-
rative version.
REFERENCES
Birge, B. (2003). PSOt - a particle swarm optimization tool-
box for use with Matlab. In Swarm Intelligence Sym-
posium, 2003. SIS ’03. Proceedings of the 2003 IEEE,
pages 182–186.
Carlisle, A. and Dozier, G. (2001). An off-the-shelf PSO.
In Proceedings of the Particle Swarm Optimization
Workshop, pages 1–6, Indianapolis, Indiana, USA.
Clerc, M. and Kennedy, J. (2002). The particle swarm -
explosion, stability, and convergence in a multidimen-
sional complex space. IEEE Transactions on Evolu-
tionary Computation, 6(1):58–73.
Fern´andez-Mart´ınez, J. L. and Garc´ıa-Gonzalo, E. (2008).
The generalized PSO: a new door to PSO evolu-
tion. Journal of Artificial Evolution and Applications,
2008:1–15.
Fern´andez-Mart´ınez, J. L. and Garc´ıa-Gonzalo, E. (2009).
The PSO family: deduction, stochastic analysis and
comparison. Swarm Intelligence, 3(4):245–273.
Fern´andez-Mart´ınez, J. L. and Garc´ıa-Gonzalo, E. (2010a).
Handbook of Swarm Intelligence –Concepts, Princi-
ples and Applications, chapter What makes Particle
Swarm Optimization a very interesting and powerful
algorithm? Adaptation, Learning and Optimization.
Springer.
Fern´andez-Mart´ınez, J. L. and Garc´ıa-Gonzalo, E. (2010b).
Stochastic stability analysis of the linear continuous
and discretePSO models. Technical report, Depart-
ment of Mathematics. University of Oviedo. Submit-
ted to IEEE Transactions on Evolutionary Computa-
tion.
Fern´andez-Mart´ınez, J. L., Garc´ıa-Gonzalo, E., and
Fern´andez-
´
Alvarez, J. (2008). Theoretical analysis of
particle swarm trajectories through a mechanical anal-
ogy. International Journal of Computational Intelli-
gence Research, 4(2):93–104.
Fern´andez-Mart´ınez, J. L., Garc´ıa-Gonzalo, E., Fern´andez-
´
Alvarez, J. P., Kuzma, H. A., and Men´endez-P´erez,
C. O. (2010a). PSO: A powerful algorithm to solve
geophysical inverse problems. application to a 1D-
DC resistivity case. Jounal of Applied Geophysics,
71(1):13–25.
Fern´andez-Mart´ınez, J. L., Garc´ıa-Gonzalo, E., and Naudet,
V. (2010b). Particle Swarm Optimization applied to
the solving and appraisal of the streaming potential
inverse problem. Geophysics. Accepted for publica-
tion.
Fern´andez-Mart´ınez, J. L., Kuzma, H. A., Garc´ıa-Gonzalo,
E., Fern´andez-D´ıaz, J. M., Fern´andez-
´
Alvarez, J.,
and Men´endez-P´erez, C. O. (2009). Application of
global optimization algorithms to a salt water intru-
sion problem. Symposium on the Application of Geo-
physics to Engineering and Environmental Problems,
22(1):252–260.
Fern´andez-Mart´ınez, J. L., Mukerji, T., and Garc´ıa-
Gonzalo, E. (2010c). Particle Swarm Optimization in
high dimensional spaces. In 7th International Confer-
ence on Swarm Intelligence (ANTS 2010), Brussels,
Belgium.
Garc´ıa-Gonzalo, E. and Fern´andez-Mart´ınez, J. L. (2009).
The PP-GPSO and RR-GPSO. Technical report, De-
partment of Mathematics. University of Oviedo. Sub-
mitted to IEEE Transactions on Evolutionary Compu-
tation.
Kennedy, J. and Eberhart, R. (1995). Particle swarm op-
timization. In Proceedings IEEE International Con-
ference on Neural Networks (ICNN ’95), volume 4,
pages 1942–1948, Perth, WA, Australia.
Trelea, I. (2003). The particle swarm optimization algo-
rithm: convergence analysis and parameter selection.
Information Processing Letters, 85(6):317 – 325.
ICEC 2010 - International Conference on Evolutionary Computation
242