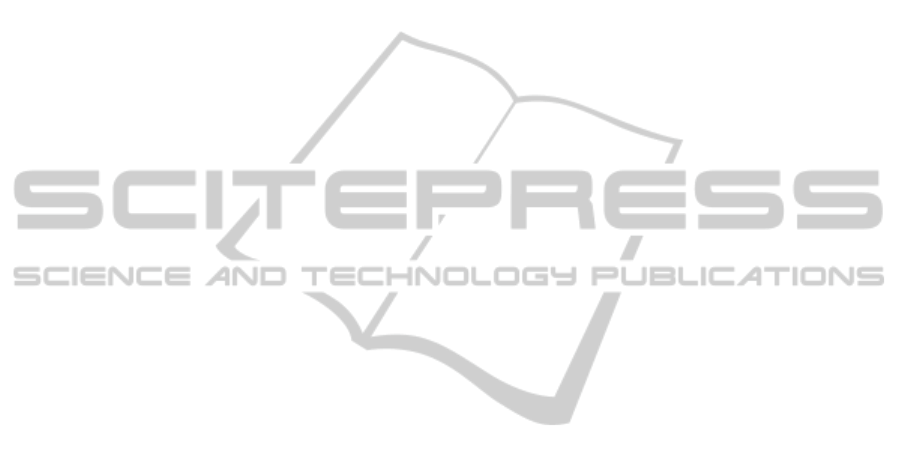
2002) that a hand, during grasping action, can effec-
tively assume a reduced number of hand shapes. For
this reason it is reasonable to conjecture that vision-
based hand pose estimation becomes simpler if one
“knows” which kind of action is going to be executed.
This is the main rationale behind our system. The re-
sults of the DA-TEST show that this system is able
to give a good estimation of hand pose in the case of
different grasping actions.
In the first phase of the DV-TEST comparable re-
sults with respect to the DA-TEST have been ob-
tained. It must be emphasized, moreover, that al-
though the system has been tested on the same view-
points it was trained on, the system does not know in
advance which viewpoint a frame drawn from a test
action belongs to.
In the second phase of the DV-TEST an accept-
able error was obtained from one viewpointonly. This
negative outcome is likely to depend on excessively
high differences in degrees between two consecutive
training viewpoints. Thus a more precise investiga-
tion must be performed with a more comprehensive
set of viewpoints. A linear combination of the out-
puts of the CHC modules, on the basis of the produced
errors, can be investigated too. Furthermore, the FE
module can be replaced with more sophisticated mod-
ules, in order to extract more significant features such
as Histograms of Oriented Gradients (HOGs) (Dalal
and Triggs, 2005). A comparison with other ap-
proaches must be performed. In this regard, however,
the lack of some benchmark datasets make meaning-
ful comparisons between different systems difficult to
produce. Finally, an extension of this model might
profitably take into account graspable object proper-
ties (Prevete et al., 2010) in addition to hand visual
features.
ACKNOWLEDGEMENTS
This work was partly supported by the project Dex-
mart (contract n. ICT-216293) funded by the EC un-
der the VII Framework Programme, from Italian Min-
istry of University (MIUR), grant n. 2007MNH7K2
003, and from the project Action Representations
and their Impairment (2010-2012) funded by Fon-
dazione San Paolo (Torino) under the Neuroscience
Programme.
REFERENCES
Aleotti, J. and Caselli, S. (2006). Grasp recognition in vir-
tual reality for robot pregrasp planning by demonstra-
tion. In ICRA 2006, pages 2801–2806.
Bishop, C. M. (1995). Neural Networks for Pattern Recog-
nition. Oxford University Press.
Chang, L. Y., Pollard, N., Mitchell, T., and Xing, E. P.
(2007). Feature selection for grasp recognition from
optical markers. In IROS 2007, pages 2944 – 2950.
Dalal, N. and Triggs, B. (2005). Histograms of oriented gra-
dients for human detection. In CVPR’05 - Volume 1,
pages 886–893, Washington, DC, USA. IEEE Com-
puter Society.
Erol, A., Bebis, G., Nicolescu, M., Boyle, R. D., and
Twombly, X. (2007). Vision-based hand pose estima-
tion: A review. Computer Vision and Image Under-
standing, 108(1-2):52–73.
Friston, K. (2005). A theory of cortical responses. Philos
Trans R Soc Lond B Biol Sci, 360(1456):815–836.
Ju, Z., Liu, H., Zhu, X., and Xiong, Y. (2008). Dynamic
grasp recognition using time clustering, gaussian mix-
ture models and hidden markov models. In ICIRA ’08,
pages 669–678, Berlin, Heidelberg. Springer-Verlag.
Keni, B., Koichi, O., Katsushi, I., and Ruediger, D. (2003).
A hidden markov model based sensor fusion ap-
proach for recognizing continuous human grasping
sequences. In Third IEEE Int. Conf. on Humanoid
Robots.
Kilner, J., James, Friston, K., Karl, Frith, C., and Chris
(2007). Predictive coding: an account of the mirror
neuron system. Cognitive Processing, 8(3):159–166.
Napier, J. R. (1956). The prehensile movements of the hu-
man hand. The Journal of Bone and Joint Surgery,
38B:902–913.
Palm, R., Iliev, B., and Kadmiry, B. (2009). Recognition of
human grasps by time-clustering and fuzzy modeling.
Robot. Auton. Syst., 57(5):484–495.
Poppe, R. (2007). Vision-based human motion analysis:
An overview. Computer Vision and Image Under-
standing, 108(1-2):4 – 18. Special Issue on Vision
for Human-Computer Interaction.
Prevete, R., Tessitore, G., Catanzariti, E., and Tamburrini,
G. (2010). Perceiving affordances: a computational
investigation of grasping affordances. Accepted for
publication in Cognitive System Research.
Prevete, R., Tessitore, G., Santoro, M., and Catanzariti,
E. (2008). A connectionist architecture for view-
independent grip-aperture computation. Brain Re-
search, 1225:133–145.
Romero, J., Kjellstrom, H., and Kragic, D. (2009). Monoc-
ular real-time 3d articulated hand pose estimation .
In IEEE-RAS International Conference on Humanoid
Robots (Humanoids09).
Santello, M., Flanders, M., and Soechting, J. F. (2002). Pat-
terns of hand motion during grasping and the influ-
ence of sensory guidance. Journal of Neuroscience,
22(4):1426–1235.
Weinland, D., Ronfard, R., and Boyer, E. (2010). A Survey
of Vision-Based Methods for Action Representation,
Segmentation and Recognition. Technical report, IN-
RIA.
AN ACTION-TUNED NEURAL NETWORK ARCHITECTURE FOR HAND POSE ESTIMATION
363