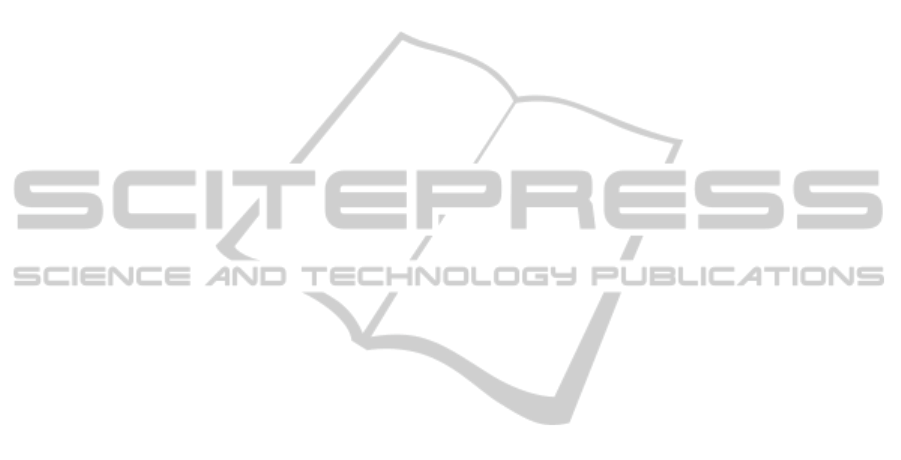
Altschul, S., Madden, T., Schaffer, A., Zhang, J., Zhang,
Z., Miller, W., & Lipman, D., 1997. Gapped BLAST
and PSI-BLAST: a new generation of protein database
search programs. Nucleic Acids Res, 25(17) 3389-
3402.
Berezin, C., Glaser, F., Rosenberg, J., Paz, I., Pupko, T.,
Fariselli, P., Casadio, R., & Ben-Tal, N., 2004.
ConSeq: the identification of functionally and
structurally important residues in protein sequences.
Bioinformatics, 20, (8) 1322-1324.
Bondugula, R. & Xu, D., 2008. Combining Sequence and
Structural Profiles for Protein Solvent Accessibility
Prediction, In Comput Syst Bioinformatics ConfC,
195-202.
Carugo, O., 2003. Prediction of polypeptide fragments
exposed to the solvent. In Silico Biology, 3(4), 417-
428.
Chen, H., Zhou, H.-X., Hu, X., & Yoo, I., 2004
Classification Comparison of Prediction of Solvent
Accessibility from Protein Sequences, In 2nd Asia-
Pacific Bioinformatics Conference (APBC), 333-338.
Cheng, J., Sweredoski, M., & Baldi, P., 2006. DOMpro:
Protein Domain Prediction Using Profiles, Secondary
Structure, Relative Solvent Accessibility, and
Recursive Neural Networks. Data Mining and
Knowledge Discovery, 13(1) 1-10
Cortes, C. & Vapnik, V., 1995. Support vector networks.
Machine Learning, 20, 1-25.
David, M. P., Asprer, J. J., Ibana, J. S., Concepcion, G. P.,
& Padlan, E. A., 2007. A study of the structural
correlates of affinity maturation: Antibody affinity as a
function of chemical interactions, structural plasticity
and stability. Molecular Immunology, 44 (6), 1342-
1351.
Fan, R. E, Chen, P. H. and Lin, C. J., 2005. Working set
selection using second order information for training
SVM. Journal of Machine Learning Research, 6,
1889-1918.
Gianese, G., Bossa, F., & Pascarella, S., 2003.
Improvement in prediction of solvent accessibility by
probability profiles. Protein Engineering Design and
Selection, 16(12) 987-992.
Huang, G. B., Zhu, Q. Y., & Siew, C. K., 2006. Extreme
learning machine: Theory and applications.
Neurocomputing, 70, (1-3) 489-501
Kim, H. & Park, H., 2004. Prediction of protein relative
solvent accessibility with support vector machines and
long-range interaction 3D local descriptor. Proteins -
Structure, Function, and Bioinformatics, 54 (3), 557-
562.
Manesh, N. H., Sadeghi, M., Arab, S., & Movahedi, A. A.
M., 2001. Prediction of protein surface accessibility
with information theory. Proteins - Structure,
Function, and Genetics, 42 (4) 452-459.
Meshkin, A. & Ghafuri, H., 2010. Prediction of Relative
Solvent Accessibility by Support Vector Regression
and Best-First Method. EXCLI, 9, 29-38.
Mucchielli-Giorgi, M. H., Hazout, S., & Tuffery, P., 1999.
PredAcc: prediction of solvent accessibility.
Bioinformatics, 15 (2) 176-177.
Nguyen, M. N. & Rajapakse, J. C., 2005. Prediction of
protein relative solvent accessibility with a two-stage
SVM approach. Proteins, 59, (1) 30-37.
Ooi, T., Oobatake, M., Namethy, G., & Scheraga, H. A.,
1987. Accessible surface areas as a measure of the
thermodynamic parameters of hydration of peptides.
Proceedings of the National Academy of Sciences of
the United States of America, 84 (10) 3086-3090.
Petersen, B., Petersen, T. N., & Andersen , P., Nielsen,
M., Lundegaard, C., 2009. A generic method for
assignment of reliability scores applied to solvent
accessibility predictions. BMC Structural Biology,
9:51.
Pollastri, G., Baldi, P., Fariselli, P., & Casadio, R., 2002.
Prediction of coordination number and relative solvent
accessibility in proteins. Proteins, 47(2) 142-153.
Pollastri, G., Martin, A., Mooney, C., & Vullo, A., 2007.
Accurate prediction of protein secondary structure and
solvent accessibility by consensus combiners of
sequence and structure information. BMC
Bioinformatics, 8, (1) 201
Saraswathi, S., Suresh, S., Sundararajan, N., Zimmerman,
M. and Nilsen-Hamilton, M., 2010. ICGA-PSO-ELM
approach for Accurate Cancer Classification Resulting
in Reduced Gene Sets Involved in Cellular Interface
with the Microenvironment. IEEE Transactions in
Bioinformatics and Computational Biology,
http://www.computer.org/portal/web/csdl/doi/10.1109/
TCBB.2010.13.
Suresh, S., Saraswathi, S., Sundararajan, N., 2010.
Performance Enhancement of Extreme Learning
Machine for Multi-category Sparse Data Classification
Problems. Engineering Applications of Artificial
Intelligence,
http://dx.doi.org/10.1016/j.engappai.2010.06.009.
Shandar, A. & Gromiha, M. M., 2002. NETASA: neural
network based prediction of solvent accessibility.
Bioinformatics, 18(6), 819-824.
Shen, B. & Vihinen, M., 2003. RankViaContact: ranking
and visualization of amino acid contacts.
Bioinformatics, 19(16), 2161-2162.
Sim, J., Kim, S.-Y., & Lee, J., 2005. Prediction of protein
solvent accessibility using fuzzy k -nearest neighbor
method. Bioinformatics, 21(12), 2844-2849.
Singh, Y. H., Gromiha, M. M., Sarai, A., & Ahmad, S.,
2006. Atom-wise statistics and prediction of solvent
accessibility in proteins. Biophysical Chemistry,
124(2), 145-154.
Wagner, M., Adamczak, R., Porollo, A., & Meller, J.,
2005. Linear regression models for solvent
accessibility prediction in proteins. J Comput Biol,
12(3), 355-369.
Wang, J. Y., Lee, H. M., & Ahmad, S., 2007. SVM-
Cabins: prediction of solvent accessibility using
accumulation cutoff set and support vector machine.
Proteins, 68(1), 82-91.
Zarei, R., Arab, S., & Sadeghi, M., 2007. A method for
protein accessibility prediction based on residue types
and conformational states. Computational Biology and
Chemistry, 31(5-6) 384-388.
AN EXTREME LEARNING MACHINE CLASSIFIER FOR PREDICTION OF RELATIVE SOLVENT
ACCESSIBILITY IN PROTEINS
369