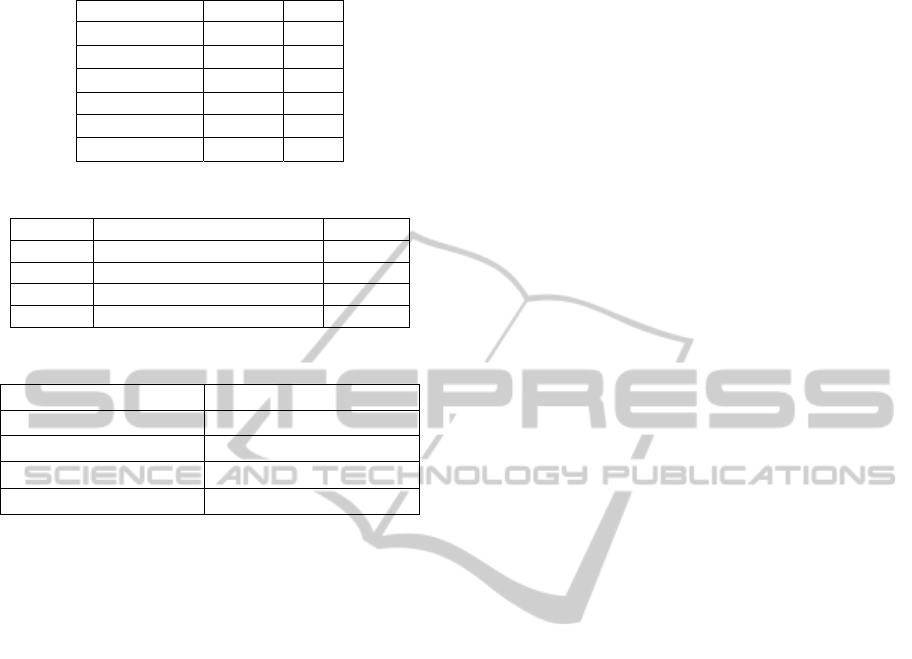
Table 4: The second-level AHP optimization results.
Efficient supplierPerf. index Position
f
PI
iAH
i
3
0.132 4
5
0.117 5
10
0.273 1
11
0.219 3
14
0.258 2
Table 5: The third-level linear programming problem data.
Supplier Minimum requested quantity Capacity
f δ
i
γ
i
10 0.200 0.600
11 0.200 0.400
14 0.200 0.700
Table 6: The third-level linear programming results.
Supplier Required quantity
f q
i
10 0.400
11 0.200
14 0.400
After rnking the efficient suppliers, the linear
programming problem (13)-(14)-(15)-(16) is defined
and solved for a multiple sourcing strategy with μ=3.
The values of minimum required quantities δ
i
and
percentage capacities γ
i
of suppliers are collected in
Table 5. Table 6 shows the results of the linear
programming problem solution, i.e., the required
quantities from the three effective suppliers s
10
, s
11
,
and s
14
. Results show that 40% of the supply will be
provided in turn by each of the two most effective
suppliers, i.e., s
10
and s
14
, whereas the remaining
20% of the requested product will be provided by
the third-ranked supplier s
11
.
4 CONCLUSIONS
The paper focuses on a crucial issue of purchasing in
supply chains, i.e., vendor evaluation and selection.
A novel three-step methodology based on the Data
Envelopment Analysis (DEA) approach, the
Analytic Hierarchy Process (AHP), and linear
programming is presented. At the first level of the
hierarchical technique, a vendor rating technique
based on DEA is devised to obtain efficient and
inefficient suppliers. Hence, the AHP process is
applied to rank the efficient vendors based on the
overall performance index. Finally, a linear
programming problem is solved to split the supply
adopting a multiple sourcing strategy. To the best of
the authors’ knowledge, no one in the related
literature has ever joined these three approaches for
supplier selection in such a context. A numerical
case study shows the effectiveness of the presented
three step method for a C class component. Future
perspectives are identifying a real case study to
further verify the approach flexibility and simplicity
of use by the firm purchasing manager.
ACKNOWLEDGEMENTS
This work was supported by the TRASFORMA
“Reti di Laboratori” network funded by Apulia
Italian Region.
REFERENCES
Charnes A., Cooper W.W., Rhodes E., 1978. Measuring
the efficiency of decision making units; European
Journal of Operational Research, 2, 429–444.
Costantino N., Dotoli M., Falagario M., Fanti M.P.,
Iacobellis G., 2009. A Decision Support System
Framework for Purchasing Management in Supply
Chains, Journal of Business and Industrial Marketing,
24(3/4): 278-290.
Degraeve Z., Labro E., Roodhooft F., 2000. An evaluation
of vendor selection models from a total cost of
ownership perspective; European J. of Operational
Research, 125(1), 34-58.
Dotoli M., Fanti M.P., Meloni C., Zhou M.C., 2006.
Design and optimization of integrated e-supply chain
for agile and environmentally conscious
manufacturing, IEEE Transactions on Systems Man
and Cybernetics, part A, 36(1), 62-75.
Ho W., Xu X., Dey P.K., 2010. Multi-criteria decision
making approaches for supplier evaluation and
selection: A literature review, European Journal of
Operational Research, 202, 16-24.
Krajewski L., Ritzman L., 2002. Operation Management:
Strategy and Analysis, Prentice Hall, London, UK, 6th
ed.
Saaty T.L., 1990. How to Make a Decision: the Analytic
Hierarchy Process, European Journal of Operational
Research, 48:9-26.
Talluri, S., Narasimhan, R., Nair, A., 2006. Vendor
performance with supply risk: A chance-constrained
DEA approach; International Journal of Production
Economics 100(2), 212–222.
Wang Y.-M., Chin K.-S., 2010. A neutral DEA model for
cross-efficiency evaluation and its extension; Expert
Systems with Applications, Elsevier, 37, 3666-3675.
KMIS 2010 - International Conference on Knowledge Management and Information Sharing
200