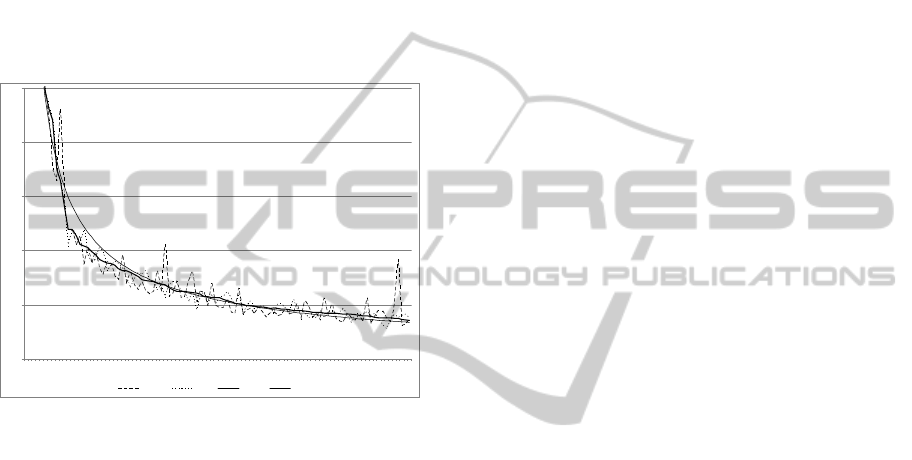
'a', 'an', 'the', 'in', 'and') are usually not presented as
single words in Hebrew, but rather as prefixes of the
words that come immediately after.
As can be seen from figure 1, Zipf’s law (with
the values of c=0.017 &
α
= 0.7 in formula (1)
succeeds to describe the distribution of the top 100
words according to the three implemented methods.
Another important finding is the fact that TFN
presents the smoothest curve. Indeed, this fact is
quite trivial since the graph deals with the
frequencies of top occurring words according to
their place and TFN is the only method that actually
expresses this relation.
0.00
0.10
0.20
0.30
0.40
0.50
1 6 11 16 21 26 31 36 41 46 51 56 61 66 71 76 81 86 91 96
IDFnorm TFDN TFnorm Zip f
Figure 1: Frequencies' rates according to Zipf's law and
the baseline methods for the top 100 words.
5 CONCLUSIONS AND FUTURE
WORK
In this ongoing work, we present an implementation
of three baseline methods that attempt to extract
stopwords in Hebrew for a data set containing Israeli
daily news. Two of the methods are state-of-the-art
methods previously applied to other languages and
the third method is proposed by us.
A comparison of the behavior of these methods
to the behaviour of the Zipf's law shows that Zipf’s
law succeeds to describe the distribution of the top
occurring Hebrew words.
Future directions for research are: (1) Applying
this research into larger and more diverse corpora to
produce a general stopword list and domain-specific
stopword lists, (2) Performing additional and
extended experiments to evaluate the various
stopword lists through retrieval of web queries in
Hebrew, (3) Investigating whether other methods
can be discovered to achieve more effective
stopword lists for IR tasks, and (4) Defining new
stopword lists using word lemmatization.
REFERENCES
Choueka, Y., Conley, E. S., Dagan, I. 2000. A
comprehensive bilingual word alignment system:
application to disparate languages - Hebrew, English.
in Veronis J. (Ed.), Parallel Text Processing, Kluwer
Academic Publishers, 69-96
Fox, C., 1990. A Stop List for General Text. ACM-SIGIR
Forum, 24, 19–35.
Fox, C., 1992. Lexical analysis and stoplists. In
Information Retrieval - Data Structures & Algorithms,
102-130, Prentice-Hall.
Francis, W., 1982. Frequency Analysis of English Usage:
Lexicon and Grammar. Houghton Mifflin.
Frakes, W., Baeza-Yates, R. 1992. Information retrieval:
Data Structures and Algorithms. Englewood Cliffs,
NJ: Prentice Hall.
Lazarinis, F., 2007. Engineering and utilizing a stopword
list in Greek Web retrieval. JASIST, 58(11): 1645-
1652.
Lo, R. T.-W., He, B., Ounis, I., 2005. Automatically
Building a Stopword List for an Information Retrieval
System. Journal Of Digital Information Management:
Special Issue On The 5th Dutch-belgian Information
Retrieval Workshop (dir'05), 31(3), 3-8.
Makrehchi, M., Kamel M. S., 2008. Automatic Extraction
of Domain-Specific Stopwords from Labeled
Documents. In proc. of ECIR-08, 222-233.
Raghavan, V. V., Wong S. K. M., 1986. A critical analysis
of vector space model for information retrieval,
Journal of the American Society for Information
Science, 37(5), 279-287.
Robertson S. E., Sparck-Jones. K., 1976. Relevance
weighting of search terms. Journal of the American
Society for Information Science, 27(3): 129-146.
Salton, G., McGill, M. J., 1983. Introduction to Modern
Information Retrieval. New York: McGraw-Hill.
Salton, G., Buckley, C., 1988. Term-Weighting
Approaches in Automatic Text Retrieval. Information
Processing and Management, 24, 513-523.
Savoy, J. A., 1999. Stemming Procedure and Stopword
List for General French Corpora. Journal of the
American Society for Information Science, 50(10),
944-952.
Sinka, M. P., Corne, D. W., 2002. A large benchmark
dataset for web document clustering, in Soft
Computing Systems: Design, Management and
Applications, Volume 87 of Frontiers in Artificial
Intelligence and Applications, 881-890.
Sinka, M. P., Corne, D. W., 2003. Evolving better stoplists
for document clustering and web intelligence. Design
and application of hybrid intelligent systems, 1015-
1023.
KDIR 2010 - International Conference on Knowledge Discovery and Information Retrieval
452