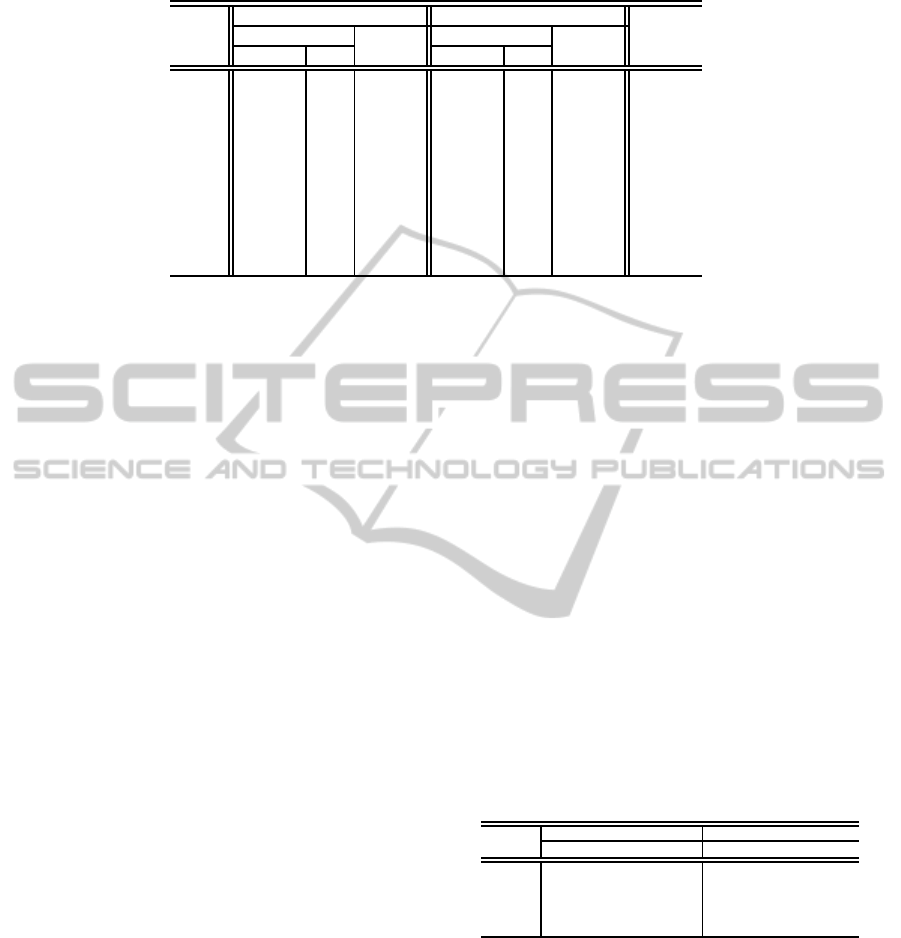
Table 1: Model parameters of the proposed and Borda’s aggregation models.
Query Order based Time based Unified
Proposed Borda’s Proposed Borda’s
ID π
0
ˆ
θ
(o)
π
0
π
0
ˆ
θ
(t)
π
0
π
0
Q1 1,2,3,5,4 1.23 1,2,3,5,4 1,2,3,5,4 0.94 1,2,3,5,4 1,2,3,5,4
Q2 2,1,3,4,5 0.25 2,1,4,3,5 2,1,4,3,5 0.21 2,1,4,3,5 2,1,3,4,5
Q3 2,5,3,1,4 0.65 2,5,3,1,4 5,2,3,1,4 0.48 5,2,3,1,4 2,5,3,1,4
Q4 4,5,2,1,3 0.51 4,5,2,1,3 4,2,5,1,3 0.47 4,2,5,1,3 4,5,2,1,3
Q5 5,3,4,1,2 0.59 5,3,4,1,2 5,3,4,1,2 0.48 5,3,4,1,2 5,3,4,1,2
Q6 5,4,3,1,2 0.43 5,4,3,1,2 4,5,3,2,1 0.24 3,5,4,1,2 5,4,3,1,2
Q7 1,4,2,3,5 0.28 1,4,2,3,5 1,4,2,3,5 0.33 1,4,2,3,5 1,4,2,3,5
Q8 4,5,1,2,3 0.36 4,5,1,2,3 4,5,1,3,2 0.26 4,5,3,1,2 4,5,1,2,3
Q9 2,5,3,4,1 0.72 2,5,3,4,1 2,5,3,4,1 0.69 2,5,3,4,1 2,5,3,4,1
Q10 2,3,4,1,5 0.52 2,3,4,5,1 2,3,4,5,1 0.48 2,3,5,4,1 2,3,4,5,1
4.1 Results and Discussion
The sessionwise feedback data for the click sequence
based and time based feedbacks have been aggre-
gated through, the proposed method and the Borda’s
method and the results are tabulated in Table 1.
From Table 1, it can be observed that the π
0
ob-
tained through the proposed method for click se-
quence based and time based feedbacks are same as
that of their respective Borda’s aggregations (8 out of
10 cases in both the schemes). This is supportive of
the fact that the maximum likelihood estimator for π
0
would converge to the ordering obtained by arranging
the elements based on the vector of average ranks
¯
π,
(Fligner and Verducci, 1988).
The partial orders obtained for a feedback from
various sessions are all observations from model
(3). For a particular feedback, sessionwise obser-
vations are all from the same population and there-
fore, they all get equal weights in the aggregation
process, resulting in an ordering same as that of
Borda’s aggregation. But in contrast, while aggregat-
ing the observations from different feedbacks, since
they are all from population differing in their dis-
persion parameter (precision), fusioning with equal
weights would be misleading. Borda’s method in-
stead gives equal weights to the ranking schemes ig-
noring the differences in accuracies. Eventhough the
weighted Borda’s method proposes to use precision
based weights, it appears less attractive, because, the
precision values are not readily available and need
to be fed externally which is impractical. But in the
proposed method, the weights (intuitively dispersion
parameters) are assigned inherently by the very con-
struction of the model and hence, the feedback rank-
ings are combined in to final ranking of documents
based on their expertise.
From Table 1, it can be observed that the
ˆ
θ
(t)
s of
time based aggregation are found to be lesser when
compared to the respective
ˆ
θ
(o)
s of click sequence
based aggregation. This can be supported by the ar-
gument that the click sequence based information is
ordinal and the time based information is continu-
ous. Therefore, the time based ranking will be more
sensitive, i.e., even a second difference in the read-
ing time will force a document to be ranked differ-
ently. Though it will have a modal ranking π
0
, the
mass won’t be concentrated heavily on one ranking.
This very fact can be seen in the frequency Table 2
for a typical query. Since the time based rankings
are widely dispersed, its aggregation parameter θ
(t)
is expected to be less when compared to the aggre-
gation parameter θ
(o)
of highly skewed (biased) click
sequence based ranking. This motivates the need of
the proposed unified aggregation model (Section 3.2)
which can make trade off between the two aggrega-
tions and fully extract the advantages of both the feed-
backs.
Table 2: Order-based and time-based rank frequency distri-
bution of the documents for a typical query.
Order-based Time-based
URLs 1 2 3 4 5 1 2 3 4 5
URL31 6 4 1 1 0 7 3 1 1 0
URL32 1 5 1 1 1 5 2 1 1 0
URL33 4 12 4 0 0 9 8 3 0 0
URL34 12 6 0 0 0 8 9 0 0 1
URL35 16 2 1 0 0 10 7 2 0 0
From Table 1, it can be seen that for relatively
lower values of
ˆ
θ
(t)
(when compared to
ˆ
θ
(o)
) the uni-
fied aggregation model produces π
0
equal to that of
the click sequence based aggregation and for rela-
tively higher values of
ˆ
θ
(t)
it produces a π
0
which
forms the trade off between the two implicit feedback
rankings considered. As the loglikelihood of com-
bined aggregation model is the summation of loglike-
lihoods of its component rankers, it could break the
ties that emerge more frequently while estimating π
0
s
from the maximum loglikelihood. The preliminary
results hinted the possibility of using the unified ag-
AGGREGATION OF IMPLICIT FEEDBACKS FROM SEARCH ENGINE LOG FILES
273