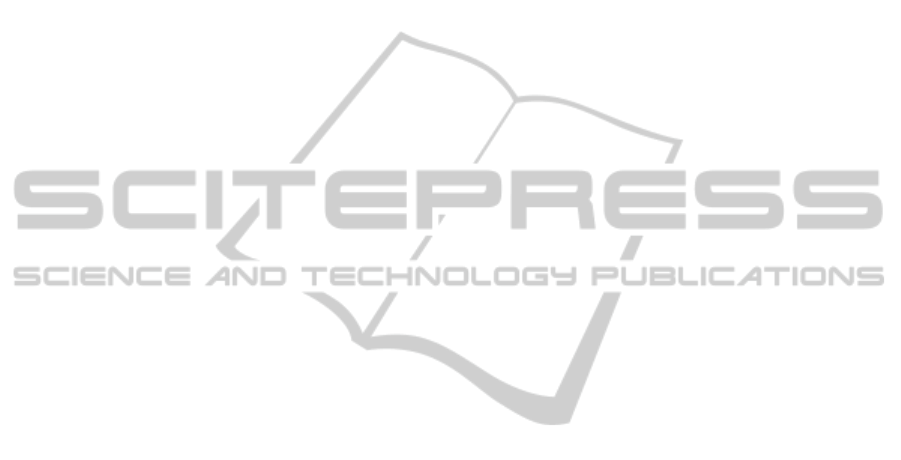
acquisition in real-time, storage, integration and
rapid availability of all clinical information.
5 CONCLUSIONS AND FUTURE
WORK
The main concern in ICU is to avoid or reverse
organ failure, in order to preserve the patients’ lives.
The INTCare system is being developed for hourly
prediction of the patients’ clinical condition, i.e. the
prediction of dysfunction/failure of the organ
systems (cardiovascular, respiratory, renal,
coagulation and liver systems) and outcome. We
believe that, with this fine grained prediction, it will
be possible for the healthcare professionals to have a
timely intervention and a proactive attitude so that
worst complications for the patients may be avoided.
Further work will encompass the test of the DM
models generated so far, with online and real-time
data from the ICU of HSA, in order to guarantee
their accuracy or, in case their performance decays,
to optimize them. The models presented used data
manually entered and the next step is to use them
with the new adjustments of the system, i.e., online
and in real-time. Prediction, optimization and
adaptability are features that make INTCare an ABI
system, whose maid goal it to allow the medical
staff to make better decisions, at the right time and
place, improving quality in health care.
The integration with the various data sources and
with the rest information systems of the hospital has
been supported by the development of an ENR and
further related work include its test in the ICU and
subsequently, its optimization.
REFERENCES
Abelha, A., J. Machado, M. Santos, S. Allegro, F. Rua, M.
Paiva & J. Neves. Agency for Integration, Diffusion
and Archive of Medical Information.
Arnott, D. & G. Pervan. 2004. A critical analysis of
decision support systems research. In Conference on
Decision Support Systems, 67-87. Prato, ITALY:
Palgrave Publishers Ltd.
Fayyad, U. M., G. Piatetsky-Shapiro & P. Smyth (1996)
From data mining to knowledge discovery: an
overview.
Fonseca, T., C. Ribeiro & C. Granja (2009) Vital Signs in
Intensive Care: Automatic Acquisition and
Consolidation into Electronic Patient Records. Journal
of Medical Systems, 33, 47-57.
Frawley, W. J., G. Piatetsky-Shapiro & C. J. Matheus
(1992) Knowledge Discovery in Databases: An
Overview. AI Magazine, 13, 57-70.
Gago, P. & M. F. Santos. 2008. Towards an Intelligent
Decision Support System for Intensive Care Units. In
18th European Conference on Artificial Intelligence.
Greece.
Gago, P., M. F. Santos, Á. Silva, P. Cortez, J. Neves & L.
Gomes (2006) INTCare: a knowledge discovery based
intelligent decision support system for intensive care
medicine. Journal of Decision Systems.
Gago, P., A. Silva & M. F. Santos. 2007. Adaptive
decision support for intensive care. In 13th Portuguese
Conference on Artificial Intelligence, ed. J. S. M. F.
M. J. M. Neves, 415-425. Guimaraes, PORTUGAL:
Springer-Verlag Berlin.
Gardner, R. M., W. L. Hawley, T. D. East, T. A. Oniki &
H. F. W. Young (1991) Real time data acquisition:
recommendations for the Medical Information Bus
(MIB). International journal of clinical monitoring
and computing, 8, 251-258.
Hall, J. B., G. A. Schmidt & L. D. H. Wood. 2005.
Principles of Critical Care. McGraw-Hill's
AccessMedicine.
Hooda, J. S., E. Dogdu & R. Sunderraman. Health Level-7
compliant clinical patient records system. 259-263.
ACM.
Jennings, N. R. (2000) On agent-based software
engineering. Artificial Intelligence, 117, 277-296.
Lourenco, A. & O. Belo (2003) Promoting agent-based
knowledge discovery in medical intensive care units.
WSEAS Transactions on Computers, 2, 403-408.
M. F. Santos M.F., V.-B. M., Machado and A. A. J.,
Neves J., Silva A., Rua F., Salazar M., Quintas C.,
Cabral A.F. Intelligent Decision Support in Intensive
Care Units - Nursing Information Requirements.
Mahmoud, M. 2003. Real-time data acquisition system for
monitoring patients in intensive care unit., 320-326.
Multisensor, Multisource Information Fusion:
Architectures, Algorithms and Applications.
Michalewicz, Z., M. Schmidt, M. Michalewicz & C.
Chiriac. 2007. Adaptive Business Intelligence
.
Springer.
Morik, K. (2003) Data analysis and knowledge validation
in intensive care monitoring.
Morik, K., P. Brockhausen & T. Joachims. Combining
statistical learning with a knowledge-based approach-a
case study in intensive care monitoring. 268-277.
Citeseer.
Negash, S. & P. Gray Business intelligence. Handbook on
Decision Support Systems 2, 175-193.
Pereira, M., A. Curra, R. Rivas, J. Pereira, G. Banos, J.
Teueiro & A. Pazos (2007) Computer aided
monitoring system of intensive care unit patients.
WSEAS Transactions on Information Science and
Applications, 4, 78-84.
Ramon, J., D. Fierens, F. Güiza, G. Meyfroidt, H.
Blockeel, M. Bruynooghe & G. Van Den Berghe
(2007) Mining data from intensive care patients.
Advanced Engineering Informatics, 21, 243-256.
REAL-TIME INTELLIGENT DECISION SUPPORT IN INTENSIVE MEDICINE
49