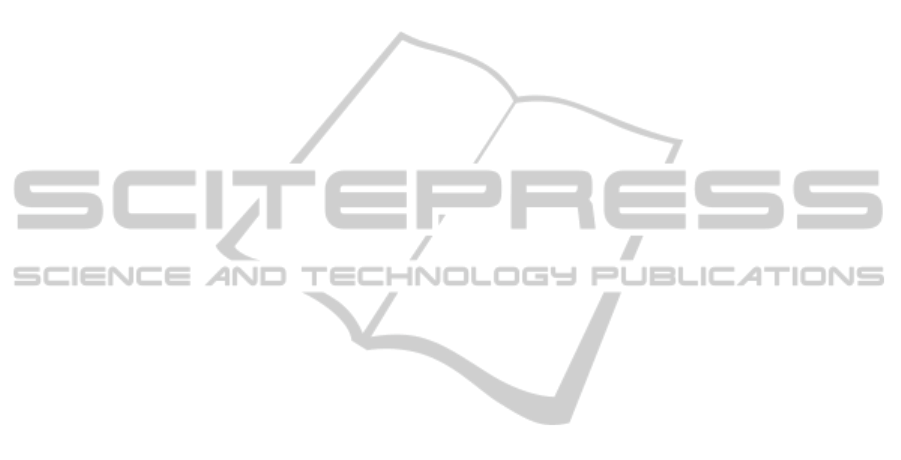
The new proposed criteria seem more restrictive
than the intrasum (used before), selecting fewer clus-
ters from the co-association matrixes obtained from
each algorithm instantiation. Nevertheless the inte-
gration of all the algorithm instantiations, for almost
all of the benchmark data-sets, resulted in the inclu-
sion of clusters that covered all the data-set.
To summarize the results for the benchmark data-
sets, we present in table 3 the CI index using the SL
and the AL hierarchical methods as extraction meth-
ods, marking for each data-set the maximal CI value.
The right half of the table presents more marked
cells, showing that the AL extraction method con-
ducted to better results. Comparison of the results
shows that the Silhouete (silh), the intermax, and the
Dunn index systematically leads to better results than
the original intrasum selection criterium. The re-
maining do not show an evident superiority, and in-
tramin is the index presenting the worst performance.
The intermax and Dunn criteria were the best in
almost every data-set. The cases in which they did not
obtain the best results correspond to situations where
only a subset of samples was selected, since these cri-
teria selected only a small part of the evaluated clus-
ters. This fact caused that some objects were not part
of any of the selected clusters, penalizing the over-
all result, since some natural clusters didn’t have any
match.
The criterion Silhouete gave also results compa-
rable with Dunn and intermax criteria in almost all
data-sets, allowing the coverage of all objects in ev-
ery data-set.
7 CONCLUSIONS
Adopting the Multiple-Criteria Evidence Accumula-
tion Clustering method (Multi-EAC) as baseline clus-
ter combination method, we addressed the issue of
selection of meaningful clusters from the multiple
data partitions. In previous work, the authors pro-
posed a cluster validity criterion based on cluster sta-
bility, assessed from intermediate co-association ma-
trices, obtained from clustering ensembles produced
by a single clustering algorithm by perturbing the
data set using sub-sampling. In this paper we pro-
posed new cluster validity criteria for the selection of
clusters from the same intermediate co-associations
matrices but using it on a different perspective. In-
stead of considering only the intra-cluster similarity,
we propose indexes based on inter-cluster similarity
and combination of intra-cluster and inter-cluster sim-
ilarities. Comparison of the several criteria was based
on the performance of the combined data partitions,
obtained by accounting only on clusters that are se-
lected according to the corresponding criteria.
Experimental results have shown that four out of
the the five proposed criteria lead in general to better
combination results than by using the cluster stabil-
ity criterion. In particular, the criterion Silhouete and
Dunn focusing both the intra and the inter-cluster sep-
arability, and the intermax focusing on intra-cluster
separability, gave the overall best results.
Furthermore, the new methods can also be ap-
plied to clustering ensembles that do not make use of
data sub-sampling, being of more general applicabil-
ity. Additional experiments on larger data sets and on
more real data sets are underway.
REFERENCES
Asuncion, A. and Newman, D. (2007). UCI ML repository.
Ayad, H. G. and Kamel, M. S. (2008). Cumulative voting
consensus method for partitions with variable number
of clusters. IEEE Trans. Pattern Anal. Mach. Intell.,
30(1):160–173.
Ben-Hur, A., Elisseeff, A., and Guyon, I. (2002). A stabil-
ity based method for discovering structure in clustered
data. In Pacific Symposium on Biocomputing.
Bezdek, J. C. and Pal, N. R. (1995). Cluster validation
with generalized dunn’s indices. In ANNES ’95: Pro-
ceedings of the 2nd New Zealand Two-Stream Interna-
tional Conference on Artificial Neural Networks and
Expert Systems, page 190, Washington, DC, USA.
IEEE Computer Society.
Bolshakova, N. and Azuaje, F. (2003). Cluster validation
techniques for genome expression data. Signal Pro-
cess., 83(4):825–833.
Dubes, R. and Jain, A. (1979). Validity studies in clustering
methodologies. Pattern Recognition, 11:235–254.
Dunn, J. C. (1974). A fuzzy relative of the isodata process
and its use in detecting compact, well separated clus-
ters. Cybernetics and Systems, 3(3):32–57.
Fern, X. Z. and Brodley, C. E. (2004). Solving cluster en-
semble problems by bipartite graph partitioning. In
ICML ’04: Proceedings of the twenty-first interna-
tional conference on Machine learning, page 36, New
York, NY, USA. ACM.
Fred, A. (2001). Finding consistent clusters in data parti-
tions. In Kittler, J. and Roli, F., editors, Multiple Clas-
sifier Systems, volume 2096, pages 309–318. Springer.
Fred, A. and Jain, A. (2005). Combining multiple cluster-
ing using evidence accumulation. IEEE Trans Pattern
Analysis and Machine Intelligence, 27(6):835–850.
Fred, A. and Jain, A. (2006). Learning pairwise similarity
for data clustering. In Proc. of the 18th Int’l Confer-
ence on Pattern Recognition (ICPR), volume 1, pages
925–928, Hong Kong.
KDIR 2010 - International Conference on Knowledge Discovery and Information Retrieval
498