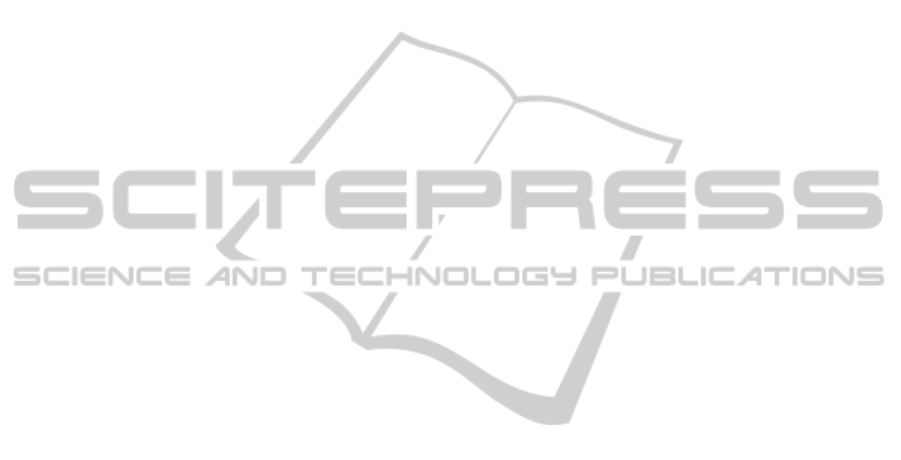
We developed an interestingness measure for pivot
objects to be able to decide which objects are more
relevant in sensemaking. In our experiments (on a
Bibsonomy dataset) we showed that, compared to a
baseline, groups of objects associated with the pivot
objects are of greater homogeneity than groups de-
rived from a baseline. Also we showed that with
decreasing interestingness, homogeneity decreases as
well. In another experiment we showed that depend-
ing on type of pivot objects (which may be user, tag
or document), structure of worlds can be completely
different. This shows that it makes a great difference
from which object type a newcomer would like to dis-
cover the folksonomy. Furthermore we could show
that worlds derived by our method differ from each
other, while baseline worlds are similar to each other.
This is desired since the newcomer expects different
worlds describing the folksonomy from different per-
spectives to be different in the objects these worlds
consist of.
REFERENCES
Abdu, E. and Salane, D. (2009). A spectral-based clustering
algorithm for categorical data using data summaries.
In KDD Workshop on Data Mining using Matrices
and Tensors.
Aggarwal, C. C. and Yu, P. S. (2006). A Framework
for Clustering Massive Text and Categorical Data
Streams. In Proceedings of the SIAM conference on
Data Mining 2006.
Banerjee, A., Basu, S., and Merugu, S. (2007). Multi-way
clustering on relation graphs. In SDM.
Begeman, G., Keller, P., and Smadja, F. (2006). Automated
tag clustering: Improving search and exploration in
the tag space. In Proceedings of the WWW’2006
Workshop on Collaborative Web Tagging.
Cattuto, C., Benz, D., Hotho, A., and Stumme, G. (2008).
Semantic grounding of tag relatedness in social book-
marking systems. In ISWC ’08: Proceedings of the
7th International Conference on The Semantic Web,
pages 615–631, Berlin, Heidelberg. Springer-Verlag.
de Gemmis, M., Lops, P., Semeraro, G., and Basile, P.
(2008). Integrating tags in a semantic content-based
recommender. In RecSys ’08: Proceedings of the
second ACM Conference on Recommender Systems,
pages 163–170, Lausanne, Switzerland. ACM.
Golder, S. and Huberman, B. (2006). Usage patterns of
collaborative tagging systems. Journal of Information
Science, 32(2):198–208.
Heymann, P. and Garcia-Molina, H. (2006). Collaborative
creation of communal hierarchical taxonomies in so-
cial tagging systems. Technical report, InfoLab, Com-
puter Science Department, Stanford University.
Hofmann, T. (2001). Unsupervised learning by proba-
bilistic latent semantic analysis. Machine Learning,
42(1):177–196.
Ipeirotis, P., Ntoulas, A., Cho, J., and Gravano, L. (2005).
Modeling and managing content changes in text
databases. In Proceedings of the IEEE Int. Conf. on
Data Engineering (ICDE’05).
Markines, B., Cattuto, C., Menczer, F., Benz, D., Hotho,
A., and Stumme, G. (2009a). Evaluating similarity
measures for emergent semantics of social tagging.
In WWW ’09: Proceedings of the 18th international
conference on World wide web, pages 641–650, New
York, NY, USA. ACM.
Markines, B., Cattuto, C., Menczer, F., Benz, D., Hotho,
A., and Stumme, G. (2009b). Evaluating similarity
measures for emergent semantics of social tagging. In
WWW’09, pages 641–650, Madrid, Spain.
Osinski, S. (2006). Improving quality of search results clus-
tering with approximate matrix factorisations. In Pro-
ceedings of the European Conf. on Information Re-
trieval (ECIR’06), LNCS 3936, pages 167–178.
Sun, J., Tao, D., and Faloutsos, C. (2006). Beyond streams
and graphs: dynamic tensor analysis. In KDD ’06:
Proceedings of the 12th ACM SIGKDD international
conference on Knowledge discovery and data mining,
pages 374–383, New York, NY, USA. ACM.
Symeonidis, P., Nanopoulos, A., and Manolopoulos, Y.
(2010). A unified framework for providing recom-
mendations in social tagging systems based on ternary
semantic analysis. IEEE Transactions on Knowledge
and Data Engineering, 22(2):179–192.
Zhou, Q., Xu, G., and Zong, Y. (2009). Web co-clustering
of usage network using tensor decomposition. In WI-
IAT ’09: Proceedings of the 2009 IEEE/WIC/ACM In-
ternational Joint Conference on Web Intelligence and
Intelligent Agent Technology, pages 311–314, Wash-
ington, DC, USA. IEEE Computer Society.
CrossSense - Sensemaking in a Folksonomy with Cross-modal Clustering over Content and User Activities
111