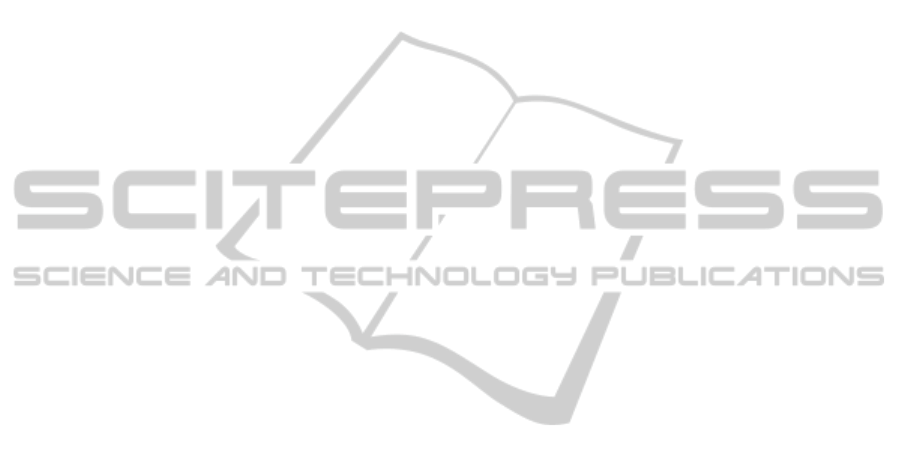
Statistics of China (NBS) (2008), the rural
population accounted for 54.3 per cent of the total
population. Due to the backwardness of rural
economic development and inadequate financial
resources, the improvement of rural education has
been seriously restricted. The statistical data shows
that 9.5 per cent of the rural labours are illiterate,
86.2 per cent are educated up to the junior high
school level which is the minimum nine-year
compulsory education in China, only 4.3 per cent
have received the high school education (NBS,
2008). The low education level of rural population
seriously hindered the development of rural
economy. Therefore, continuous education plays an
important role in improving the knowledge and
skills of people living in the rural areas. The rapid
development of information technology and the
Internet provides opportunities for them to
participate in adult continuous study in China.
With the increasing public investment in the
Internet infrastructures in China, the Internet
coverage has been extended to many rural areas,
especially in more developed regions. China Internet
Network Information Center (CNNIC) reported that
the rural Internet users have increased up to 84.6
million at a 60 per cent increasing rate each year
(CNNIC, 2009). E-learning has been considered as
an efficient solution for expanding adult education
and vocational training in rural areas (Ministry of
Agriculture, 2005). Although the applications of e-
learning have been enhanced by the government
promotion programmes and the rapid penetration of
the Internet into the rural regions, only 1.6 per cent
of 700 million rural population has registered in e-
learning programs in 2009 (CNNIC, 2010), despite
the fact that many institutions of higher education
offer e-learning courses and training course in rural
areas.
e-Learning implementation in China are facing
challenges. According to the survey of 150 e-
learning courses by Guo and Yuan (2009), there was
a lack of appropriate e-learning materials. Many
factors can affect e-learning success. For example,
Lang (2006) and Guo (2006) indicated that the
system service and system design were the problems
that could impend the system usage and student
motivation. Therefore, it is imperative to understand
the factors affecting e-learning success. It is argued
that the behavioural intention of re-use can be an
appropriate overall indicator of e-learning system
success.
This study aims to understand how e-learners
experience in the rural areas of China in using
existing e-learning system affects their behavioural
intention of re-use by developing and empirically
validating an integrated theoretical framework based
on Technology Acceptance Model (TAM),
Information System Success (ISS) model and self-
efficacy theory.
2 THE RESEARCH MODEL
The theoretical foundation and constructs were
established based on previous studies. TAM model,
ISS model and self-efficacy theory were simplified
and integrated. TAM model and ISS model
contributed to the “How success of the technology”,
and the self-efficacy theory, as an important
component of social cognition learning theory,
highlighted “How people learn with the technology”.
The research model was adopted to inform the
research hypotheses development.
TAM model which was developed by Davis et
al. (1989), has been widely used to predict user’s
acceptance of technology. In 1996, Davis et al.
modified the original TAM model and suggested
that perceived usefulness and perceived ease of use
has the direct effect on the individual’s intention to
system use. In the meanwhile, the system
characteristics, originated from external variables of
the original model, only provide the mediate effect
on intention by perceived usefulness and perceived
ease of use.
Based on a thorough and systematic analysis of
research publications in MISQ, Journal of
Management Information Systems and Information
Systems Research, DeLone and McLean (2003)
developed a modified ISS model, or D&M model in
2003. Compared with their original ISS model,
service quality is considered as an important new
dimension in the modified ISS model. The
dimension of system use is replaced by intension to
use, and individual benefit by overall benefit. Over
200 research papers have attempted to validate or
apply modified ISS model in various fields of
information system studies.
Self-efficacy was firstly introduced by
psychologist Bandura (1977). The concept has been
commonly used by researchers to investigate the
individual e-learner’s behavior. Computer-efficacy
is defined in this context as “confidence in one’s
ability to perform certain learning tasks using an e-
learning system” (Pituch and Lee, 2006). For
instance, it is found that women usually have more
computer anxiety but less computer self-efficacy
toward the internet (Whitely, 1997). Prior study has
identified that self-efficacy can influence the e-
UNDERSTANDING BEHAVIORAL INTENTION OF E-LEARNING SYSTEM RE-USE
219